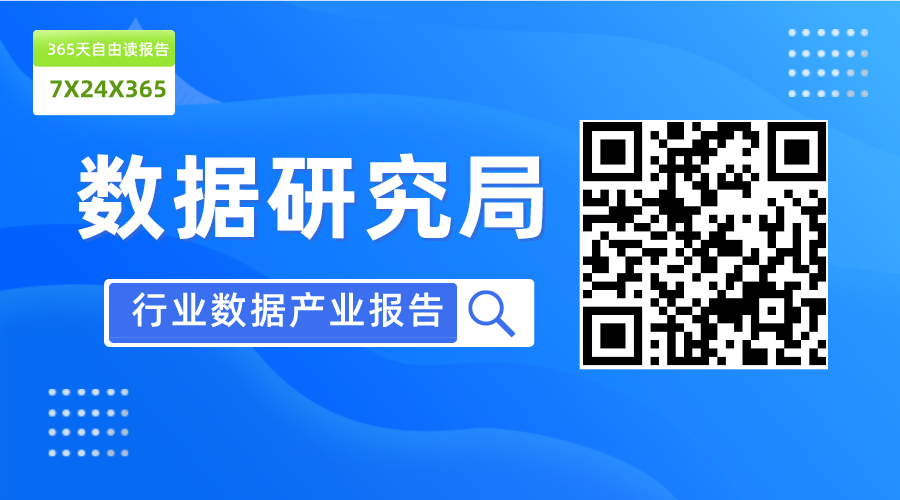
AUTHOR ACCEPTED MANUSCRIPTFINAL PUBLICATION INFORMATIONLives and Livelihoods Estimates of the Global Mortality and Poverty Effects of the COVID-19 PandemicThe definitive version of the text was subsequently published in World Development, (forthcoming 2021), 2021-09 Published by ElsevierTHE FINAL PUBLISHED VERSION OF THIS MANUSCRIPT IS AVAILABLE ON THE PUBLISHER’S PLATFORMThis Author Accepted Manuscript is copyrighted by World Bank and published by Elsevier. It is posted here by agreement between them. Changes resulting from the publishing process—such as editing, corrections, structural formatting, and other quality control mechanisms—may not be reflected in this version of the text. You may download, copy, and distribute this Author Accepted Manuscript for noncommercial purposes. Your license is limited by the following restrictions:(1) You may use this Author Accepted Manuscript for noncommercial purposes only under a CC BY-NC-ND 3.0 IGO license http://creativecommons.org/licenses/by-nc-nd/3.0/igo.(2) The integrity of the work and identification of the author, copyright owner, and publisher must be preserved in any copy.(3) You must attribute this Author Accepted Manuscript in the following format: This is an Author Accepted Manuscript by Decerf, Benoit; Ferreira, Francisco H.G.; Mahler, Daniel G.; Sterck, Olivier Lives and Livelihoods © World Bank, published in the World Development(forthcoming 2021) 2021-09 CC BY-NCND 3.0 IGO http://creativecommons.org/licenses/by-nc-nd/3.0/igo© 2021 World BankLives and livelihoods: Estimates of the global mortality and poverty eects of the Covid-19pandemic.January 11, 2021AbstractWe evaluate the global welfare consequences of increases in mortality and poverty generated by the Covid-19 pandemic. Increases in mortality are measured in terms of the number of years of life lost (LY) to the pandemic. Additional years spent in poverty (PY) are conservatively estimated using growth estimates for 2020 and two dierent scenarios for its distributional characteristics. Using years of life as a welfare metric yields a single parameter that captures the underlying trade-o between lives and livelihoods: how many PYs have the same welfare cost as one LY. Taking an agnostic view of this parameter, we compare estimates of LYs and PYs across countries for dierent scenarios. Three main ndings arise. First, we estimate that, as of early June 2020, the pandemic (and the observed private and policy responses) had generated at least 68 million additional poverty years and 4.3 million years of life lost across 150 countries. The ratio of PYs to LYs is very large in most countries, suggesting that the poverty consequences of the crisis are of paramount importance. Second, this ratio declines systematically with GDP per capita: poverty accounts for a much greater share of the welfare costs in poorer countries. Finally, a comparison of these baseline results with mortality estimates in a counterfactual herd immunity scenario suggests that welfare losses would be greater in the latter in most countries.JEL: D63, I15, I32, O15. Keywords: Covid, welfare, poverty, mortality1Highlights Years of life provide a theoretically-grounded and intuitive welfare metric for theevaluation of policies generating a lives vs livelihoods trade-o. As of early June 2020, the pandemic had generated 68 million additional povertyyears and 4.3 million years of life lost across 150 countries. The welfare costs of Covid-19 arising from increases in poverty, relative to mortality,are highest among poor countries and fall with GDP per capita. Counterfactual estimates suggest that a no-intervention scenario would have gen-erated larger welfare costs for almost all countries.21 IntroductionIn 2020-21, the world is experiencing possibly its most severe global crisis since the Second World War. The Covid-19 pandemic is rst and foremost a health crisis: in the six months since the rst cases were reported in Wuhan Province, China, in December 2019, 7.1 million cases and 406,000 deaths were conrmed worldwide by 9 June,1 and this is widely held to be an underestimate. Yet, there are other welfare costs beyond those associated with mortality induced by the disease. The disease itself and the policy and individual behavioral responses to it have induced massive economic supply and demand shocks to essentially every country in the world, triggering the deepest and most widespread economic crisis since (at least) the Great Depression of the 1930s.The fact that the bulk of the non-pharmaceutical interventions in response to the epidemic, such as lockdowns, mandatory social distancing, travel restrictions and the like, contribute to the economic costs - by preventing many or most workers from reaching production sites and consumers from demanding certain goods and services that cannot be consumed from home - has led to important debates on the optimal policy choice in the face of an apparent trade-o between lives and livelihoods (i.e. incomes and jobs). This trade-o is for instance discussed by Gourinchas (2020). Yet, as noted by Acemoglu et al. (2020), some policies can be dominated in terms of both their mortality and economic consequences.While we feel that the analysis of policy trade-os is best conducted at the level of the individual policy instrument, it may nonetheless be informative to assess the relative magnitudes of the mortality and economic costs of the pandemic. In particular, we are interested in the economic costs as manifested through increases in income poverty clearly a strict subset of all economic costs. Specically, this paper seeks to address two sets of questions: First, what were the social welfare costs of the pandemic (as of early June 2020) arising from increased mortality and higher poverty? What were their relative magnitudes, and did these magnitudes vary systematically across countries? Second, how did these welfare costs at baseline compare with plausible counterfactual estimates of the mortality costs under a hypothetical no intervention scenario in which infection rates were constrained only by herd immunity? Did these comparisons vary across countries?Naturally, a comparison of mortality and poverty costs is complicated by the fact that it inherently involves evaluating human lives. Economists typically compare economic costs to human lives using one of three approaches: attaching a monetary value to human life (Viscusi, 1993; Rowthorn and Maciejowski, 2020), estimating the indirect mortality that economic losses could imply (Ray and Subramanian, 2020), or resorting to social welfare dened as expected lifetime utility (Becker et al., 2005; Jones and Klenow, 2016;1This gure was reported on the Covid-19 Dashboard by the CSSE at Johns Hopkins University &Medicine (www.coronavirus.jhu.edu).3Alon et al., 2020). While all of these approaches have merits, each of them also has limitations. Althoughmany economists nd it meaningful to place a price on human life, most people nd the idea repugnant.2 This limits the ability of the rst approach to productively inform the public and political debate.3 The second approach, which involves computing the indirect mortality caused by the economic losses, requires making strong assumptions about governments and individuals' reactions to these losses.4 This arguably makes this approach too uncertain and hypothetical in order to serve as basis for a public debate. The third approach, based on social welfare analysis, has solid theoretical and ethical foundations (Adler et al., 2020). Yet, in its standard form, this approach generally requires selecting values for many parameters entering the denition of individuals' expected utility, such as a discount factor and the concavity of instantaneous utility. Importantly, none of their parameters directly and transparently captures the trade-o between human lives and economic losses. If this implicit trade-o can only be understood and discussed by specialists, this third approach cannot provide a decent basis for public debates on this trade-o.Our approach is rooted in social welfare analysis but diers from the earlier literature in at least two important respects. First, drawing on Baland et al. (2020), we express the key trade-o in terms of years of human life, rather than in monetary units. We measure the impact of the pandemic on human lives by the number of years of life lost to Covidinduced premature mortality (lost-years, LYs), and its economic impact by the additional number of years spent in poverty (poverty-years, PYs).5 The second dierence is that this change in metric allows us to focus on a single, central normative parameter, namely theα shadow price attached to one lost-year, expressed in terms of poverty-years. Essentiallythis parameter captures how many poverty-years are as costly (in social welfare terms) as one lost-year.This approach is clearly similar to the standard social welfare approach and we make no claim that it is theoretically superior to it. Indeed, it may even be argued to have some limitations relative to standard models, such as ignoring economic losses that do not imply a crossing of the poverty line. But we argue that our approach has three important advantages in terms of informing the public debate: First, the change in metric from monetary units to years of human life may make the relative assessment of the value2For instance, on May the 5th, New York governor Andrew Cuomo declared during his daily brieng:How much is human life worth? [. . . ] To me, [. . . ] a human life is priceless. Period.3Pindyck (2020) discusses additional limitations associated with evaluations that attach a monetaryvalue to human life.4When investigating the indirect mortality associated with the Great Recession, studies typically ndthat mortality was actually reduced, rather than increased, during the recession (Modrek et al., 2013; Tapia Granados and Iolides, 2017).5For instance, if an additional 4 percentage points of a 10 million people population spends two yearsin poverty, this implies 0.8 million poverty-years.4of a human life less shocking or repugnant to many members of the public. It may therefore overcome the emotional reaction of those who simply refuse to consider placing a monetary value on a life, and thus allow for a better-informed debate about real and important trade-os, which are otherwise simply negated.Second, we believe the use of a single, non-monetary, theoretically sound but easily interpretable parameter to express the trade-o can also be useful to inform the public debate. Finally, even the exclusive focus on poverty may make a consideration of the trade-o more acceptable to those who tend to think of economic costs as morally irrelevant when they embody losses to the rich. By focusing exclusively on increases in destitution we introduce (an admittedly coarse) distributional sensitivity which may, once again, make a public debate of the real trade-o between lives and livelihoods more acceptable, and therefore more likely. From this perspective, ignoring economic losses to the non-poor can be seen as a strength, rather than as a limitation. In short: while we do not claim that our approach is superior to those already used in the literature, we argue that it is a valuable alternative; an addition to the professional toolkit that may have some important advantages in informing the public debate.α Importantly, we do not take a view on the exact value for the normative parameter .Rather, we present estimates of the number of lost-years and the number of poverty-years induced by the pandemic for each country, under a few dierent scenarios. The estimatesα of PY/LY ratios are interpreted as empirical analogues to . They tell us how manyadditional years are spent in poverty for each year of life lost to Covid-induced mortality in that country and scenario. It is left to the reader to form an assessment of which source of welfare loss is dominant.We err on the side of caution by providing conservative estimates. The number of lostyears caused by a given death is taken to be the country-specic residual life expectancy at the age at which this death takes place.6 The number of poverty-years generated in a given country is taken to be the variation in the country's population living under the poverty threshold, caused by the Covid-induced drop in GDP forecast by Central Banks and the World Bank's Global Economics Prospect. This variation corresponds to a number of poverty-years because we assume that these individuals only remain poor for a single year. This is also a conservative assumption because we ignore any long-term eects of additional poverty, such as insults to child development from worse nutrition in early childhood; learning costs from school closures; possible hysteresis eects of unemployment, and so on. In our baseline scenario, we also assume that the economic contraction is distribution neutral, i.e. that there is no change in inequality. This is conservative since most available evidence so far suggests that the economic costs of the pandemic disproportionately burden poorer people (Bonavida Foschiatti and Gasparini,6This is conservative to the extent that individuals already having health issues such as diabetes orheart problems are more susceptible to die than healthy individuals.52020).7 To the best of our knowledge, we provide the rst global welfare analysis of the currentconsequences of the pandemic. Several papers provide a welfare analysis at national levels using the three approaches described earlier. Rowthorn and Maciejowski (2020) conduct a cost-benet analysis of the economic, social, and health consequences of the pandemic in the UK in which the value placed on a human life governs the optimal policy response. In their baseline scenario they nd the economic costs to be about four times larger than the health costs.8 This is not inconsistent with our baseline results for the UK in which we estimate that about nine years are spent in poverty for every year of life lost. Hall et al. (2020) likewise conduct a social welfare analysis which resorts to a price for a human life, computing the fraction of GDP that the United States would be willing to give up in order to avoid all potential Covid-induced deaths.Ray and Subramanian (2020) discuss the welfare implications of the pandemic in India and the indirect loss of lives that an economic lockdown entails. Though they refrain from quantifying these losses, they conclude that a lockdown without a social safety net is a less attractive option in India than in wealthier countries where the indirect mortality of such lockdowns is likely to be smaller relative to the direct health costs. These ndings are also consistent with the empirical evidence we lay out. Alon et al. (2020) investigate the dierential mortality risks between developed and developing countries using a model in which social welfare is dened as expected lifetime utility. In line with our ndings, they nd that Covid-induced mortality plays a larger role on the welfare consequences of lockdowns in developed countries than in developing countries. Also using an approach with social welfare dened as expected lifetime utility, Bethune and Korinek (2020) show that social welfare in the United States is not maximized under a no-intervention scenario, similar to what we nd for high income countries.Our analysis is also related to other recent papers. First, we borrow the time-units metric for a normative evaluation of the relative welfare costs of mortality and poverty from Baland et al. (2020). These authors employ that metric for an assessment of deprivation, whereas we use it for assessing welfare. Also, they apply their indicators to global deprivation before the outbreak of the pandemic. Second, Sumner et al. (2020), Laborde et al. (2020) and Lakner et al. (2020) estimate the Covid-induced increase in global poverty. We base our poverty estimates on the methodology developed by Lakner et al. (2020).The remainder of the paper is organized as follows. Section 2 provides a simple conceptual framework that explicitly anchors the comparison between lost-years and poverty-7For instance, Montenovo et al. (2020) show that already disadvantaged groups are disproportionatelyaected by Covid-induced job losses in the United States. Kikuchi et al. (2020) nd similar results for job and income losses in Japan. We therefore also discuss an alternative scenario where inequality is allowed to change during the pandemic.8Our calculations are based on Table 1 in Rowthorn and Maciejowski (2020).6years on standard social welfare analysis. Section 3 presents mortality and poverty estimates as of early June 2020, rst for a set of six countries (three developed and three developing) for which Covid-19 mortality data disaggregated by age are available (Section 3.1) and then for the rest of the world, using the age-distribution of deaths from population pyramids and infection-to-fatality ratios documented by Salje et al. (2020) and Verity et al. (2020) (Section 3.2). In both samples, the number of poverty-years is almost always at least ten times larger than the number of lost-years. In many cases, the PY/LY ratio is above one hundred or even one thousand. This suggests that, for most countries in the world, the welfare losses from the Covid-19 pandemic arise disproportionately from increases in poverty. This section also documents that the relative magnitude of human lives lost and poverty costs varies substantially with countries' GDP per capita, with developed countries facing both much larger mortality costs and much smaller poverty costs than developing countries - suggesting that the best policy responses may dier by country.Section 4 then compares the estimated welfare consequences as of early June to a counterfactual no-intervention scenario in which contagion only stops when herd immunity is reached.9 This permits a cleaner comparison of potential mortality burdens across dierent countries, since looking only at current mortality is hampered by the fact that countries are in dierent stages of the epidemic. In most countries, we nd that the number of lost-years under no-intervention are considerably larger than the sum of the lost-years and poverty-years generated as of early June. This implies that, even if we conservatively assume that the no-intervention scenario has no poverty consequences, its negative welfare consequences are larger than the current welfare consequences as of early June.10 This strongly suggests that no-intervention was - or would have been - a suboptimal policy, particularly in richer countries. Section 5 concludes.2 A simple conceptual frameworkThis section briey explains how our empirical comparison between years of life lost (LY) and additional years lived in poverty (PY) can be interpreted in terms of a standard utilitarian social welfare function, modied by the simplifying assumption that period utility depends only on being alive and non-poor, alive but poor, or dead. The approach is closely inspired by Baland et al. (2020), who apply it to a (dierent) problem in poverty measurement, namely developing poverty measures that account for premature mortality.9We follow Banerjee et al. (2020), whose no-intervention scenario assumes that herd immunity forCovid-19 comes about when 80% of the population is immunized.10Coming up with a convincing counterfactual under alternative policies than the one actually imple-mented is, of course, challenging. Nonetheless, several papers are now trying to model how behaviors and macroeconomic outcomes change during pandemics, using such counterfactuals (see Eichenbaum et al. (2020) or Garibaldi et al. (2020)).7It is modied to suit our present purpose.T Consider a xed calendar year . For a given country, denote the set of individuals who are alive at time T by I , indexed by i. The expected residual longevity of individual i at time T is given by di − T , where di is the expected year of her death. In each calendar year t with T ≤ t ≤ di, individual i has expected status sit, which is either being poor (P ) or being non-poor (N P ).11 The expected future lifetime utility of individual i isdiUi = u(sit),t=Twhere u is the instantaneous utility function, with u(N P ) > u(P ) > 0.12 Let the in-stantaneous utility of being dead equal zero. Abstracting from future births, a simple utilitarian expected social welfare function in this country is:W = Ui.i∈IT i Now assume that a pandemic starts in year and can aect individual 's lifetime utility in two ways. First, its economic costs may change her status from sit = N P to sit = P , for one or more years t following the outbreak. Let ∆up = u(N P ) − u(P ) denotethe instantaneous utility loss from becoming poor for one period. Second, the mortality associated with the pandemic can advance the year of the individual's death to an earliercalendar year di ≤ di. Let ∆ud denote the instantaneous utility loss of losing one perioddue to premature mortality.13Our denition of Ui implies that ∆ud should in principle depend on the counterfactual status sit that individual i would have had in t ≥ di in the absence of pandemic. To avoidthe normatively unappealing consequence of valuing the cost of premature mortalitys dierently for the poor and the non-poor, we impose that the counterfactual status it equals N P for all di ≤ t ≤ di and for all i. In other words, the utility loss from each year of life lost to the pandemic is ∆ud = u(N P ), identically for everyone.∆ Continuing to use the operator to denote the expected consequences of the pandemicrelative to a non-pandemic counterfactual, we can write the change in individual expected 11Since only two income categories are considered, our framework ignores changes in incomes that donot imply a change in income category.12For simplicity, we assume that individuals do not discount the future. Note also that this formulationis inherently conservative, in the sense that income losses that take place above or below the poverty line, without causing a crossing of that line, do not contribute to welfare losses by assumption.13Both ∆up and ∆ud are assumed to be constant over time and across individuals, and thus have no i or t subscripts. Because we focus in this paper on the mortality and poverty costs of the pandemic,we abstract from various other possible eects of a pandemic, such as the long-term eects of additional malnutrition for children, or of school stoppages. Similarly, for simplicity, we do not allow for people to be made richer or to gain additional life years from the pandemic.8utility as:didi∆Ui = Ui − Ui = 1(sit, sit)∆up + ∆ud,t=Tt=diwhere 1(sit, sit) takes value 1 if sit = N P and sit = P , and takes value 0 otherwise.Since u(P ) > 0, then ∆ud > ∆up, and we can write ∆ud = α∆up for some α > 1.Aggregating across individuals, the change in social welfare is given by: didi∆W = ∆Ui = 1(sit, sit)∆up + α∆up .i∈Ii∈I t=Tt=diNow dene LY and P Y as the sums of years lost to premature mortality and poverty,respectively, across the population, calculated as follows:LY = (di − di),i∈IPY =di1(sit, sit).i∈I t=T∆W Then the total impact on welfare of the pandemicis proportional to the weighedsum of the numbers of lost-years and poverty-years, i.e.:∆W= αLY + P Y,(1)∆upwhere the parameter α =∆ud ∆up> 1 captures how many poverty-years have the same impacton welfare as one lost-year. In our framework, this parameter captures the normativetrade-o between mortality and poverty costs. An individual might arise at their ownvaluation of the parameter by answering the following question: how many years of yourremaining life would you be willing to spend in poverty in order to increase your lifespan by one year?14 A societal value for the parameter would then be some aggregation of itscitizens' individual values. Although it is expressed in time-units instead of monetary-units, this parameter plays the same role as the dollar value of a human life in otheranalyses. In this paper, we leave to the reader the choice of her preferred value forα parameter , imposing only the lower bound at one derived above.14To be more precise, this question should specify that the number of years that the individual alreadyexpects to spend in poverty should not be counted in her answer.93 Welfare costs as of early JuneIn this section, we study the welfare consequences of the pandemic as of early June 2020.In particular, we are interested in the relative contribution of poverty and mortality coststo the overall welfare losses in each country. To answer this question, we estimate acountry's number of lost-years and compare it to an estimate of its number of poverty-years.Equation (1) tells us that to arrive at the relative contribution of the two components,α one would need a value for , which we wish to remain agnostic about. Our approach is α to compute for each country (in each scenario) the value of an observer would have tohold so as to judge that Covid-related mortality and additional poverty make identicalcontributions to the welfare costs of the pandemic, given the observed outcomes. UsingA α a superscript to denote actual, or estimated, outcomes, this "break-even" , which wecall αˆ, is given by:PY A αˆ = LY A .For any α < αˆ, additional poverty is the dominant source of the current welfare costs ofαˆ the pandemic. To be sure, we do not interpret the empirical of a given country as anαˆ estimate of this country's preference parameter. Besides policy choices, the empiricalreects a host of dierent factors, e.g. the date at which Covid-19 arrived in the country,the strength of its social safety net, its population pyramid, etc. Instead, we presentαˆ these so that the reader can form her own judgement about which form of welfare cost is dominant in the country, by comparing the empirical ratio αˆ to her own α value.3.1 Six countries with high-quality dataWe rst look at a restricted sample of six countries for which we have data on age-specic mortality from Covid-19.15 Three of them are high-income countries: Belgium, the United Kingdom (UK) and Sweden. We selected these three countries because they had some of the highest numbers of Covid-deaths per capita as of early June.16 The three remaining countries are developing countries for which age-specic mortality data are available as of early June: Pakistan, Peru and the Philippines. For each of these countries, we estimateLY A and P Y A as follows.To estimate a country's number of lost-years, we start from the age-specic mortality information: the number of Covid-related deaths distributed by age categories. Where available for individual countries, this information is obtained from Oces of National Statistics, Ministries of Health or other government oces. A specic list is included15It is not possible to nd age-specic mortality information for all countries. 16Belgium has the highest number, the United Kingdom has the second highest and Sweden hasthe fth highest, beyond Italy and Spain, but Sweden has particularly detailed age-specic mortality information.10Covid-19 Deathsin Table A1 in the Appendix.17 Then, for each death we assume that the number of lost-years is equal to the residual life expectancy at the age of death, as computed fromGlobal Burden the country's pre-pandemic age-specic mortality rates, obtained from the of Disease Database (Dicker et al., 2018). As noted earlier, we consider this assumptionconservative, since individuals who die from Covid-19 are given the same residual life expectancy as that of other individuals with the same age.One particularity of Covid-19 is that its mortality is concentrated among the old. This concentration is illustrated in Figure 1, which shows a histogram by age categories of the Covid-19 deaths observed in Sweden. This concentration implies that evaluations of mortality costs based on lost-lives, which disregard the age distribution of deaths, would tend to overestimate the welfare consequences of mortality, compared to our evaluation based on lost-years. In the case of Sweden, ignoring the age distribution of deaths would inate the importance of mortality by a factor of 4.5.182,0001,5001,0005000 0-9 10-19 20-29 30-39 40-49 50-59 60-69 70-79 80-89 90-99 Age categoriesFigure 1: Distribution of Covid-19 deaths per age in Sweden as of early June.To estimate each country's number of poverty-years, we rst take the income distribution for each country from PovcalNet for 2018, which is the latest year for which data are available. Next, we scale these distributions to 2020 by assuming that all household incomes grow in accordance with growth rates in real GDP per capita, meaning that the growth is distribution-neutral. We do so under two dierent growth scenarios: (1) using GDP growth estimates for 2019 and 2020 from around June 2020, which incorporate the expected impacts of the pandemic and associated policy responses, and (2) using GDP growth estimates for 2019 and 2020 from around January 2020, before Covid-1917All of our data sources are described in greater detail in the Appendix. 18If the same number of deaths were to be distributed at random in the population, they wouldgenerate 4.5 as many lost-years as the current distribution. This factor is equal to the average residual life expectancy in Sweden (43 years) divided by the average residual life expectancy of those dying (9.5 years).11took o. Central Bank growth estimates are used for Sweden, Belgium and the United Kingdom while growth estimates from the January and June 2020 edition of the WorldBank's Global Economic Prospects (GEP) are used for Pakistan, Peru and Philippines(The World Bank, 2020). Finally, following Lakner et al. (2020) but using each country's most recent nationalpoverty line, the impact of Covid-19 on poverty is computed by comparing the number of poor under the two growth scenarios.19 Denoting the number of poor people in yeary estimated on the basis of the growth estimate from month m in 2020 by Hym, P Y A isestimated by the dierence in dierences:P Y A = H2J0u2n0e − H2J0u1n9e − H2J0a2n0 − H2J0a1n9While we cannot rule out that GDP estimates might have changed for reasons unrelated to Covid over this time interval (January - June 2020), it is safe to say that most of the changes are due to Covid-19. The dierence in dierences calculation assures that changes in the 2019 growth rates, which cannot have been due to Covid-19, are eliminated. We assume that this additional poverty lasts only for one year, so that the number of poverty-years is simply equal to this additional number of poor people. Our analysis therefore focuses on the short-term eects of the pandemic on poverty.These are rather conservative assumptions, in the sense of yielding a small number of poverty-years, for at least two reasons. First, we assume that the additional poverty generated by the pandemic lasts only for one year. This assumption also allows us to avoid using GDP forecasts beyond 2020, the uncertainty around which is extremely large. Second, our baseline scenario assumes that all incomes grow - or shrink - in the same proportion, although there are reasons to believe that the poor could be aected more than proportionally by the recession (Bonavida Foschiatti and Gasparini, 2020). Yet, we acknowledge that, for the countries that enacted substantial social assistance policies aimed at protecting the incomes of their citizens during the crisis, our distribution-neutral assumption cannot be deemed conservative (Lustig et al., 2020). As this is mostly the case of developed countries, this assumption tends to downplay the dierence, across developed and developing countries, that we document in the relative sizes of the Covidinduced mortality and poverty welfare costs.19In all projections, it is assumed that only 85 percent of growth in GDP per capita is passed throughto growth in welfare observed in household surveys in line with historical evidence (Lakner et al., 2020). In contrast to the data from PovcalNet which are expressed in 2011 PPPs and in per capita terms, the national poverty lines for Pakistan, Peru and the Philippines are expressed in local currency units and per adult equivalents. To convert the national poverty lines into 2011 PPPs and per capita terms we follow the approach of Jollie and Prydz (2016), which is to nd the poverty line in per capita 2011 PPPs that gives the same poverty rate as the national poverty line in local currency units and per adult equivalents. The national poverty line used for Sweden, Belgium and the United Kingdom is 60% of median income in 2019. We keep this line xed at the 2019 level also for 2020 to avoid a shift in poverty lines.12The results for our six countries are summarized in Table 1. We explain how toread Table 1 using the case of Sweden. The rst six rows present basic economic anddemographic indicators, such as Sweden's GDP per capita, population, life expectancyat birth, etc. The second panel presents mortality statistics. Given the age-distributionof the 4639 Covid-related deaths recorded in Sweden (shown in row 7), each death leadson average to 9.5 lost-years. The total number of years of life lost in Sweden (up toearly June 2020) is obtained by multiplying these two numbers: 43,973. The third panelturns to the economic shock. The Central Bank of Sweden forecasts a GDP reductionof 11.5 percentage points as a result of the Covid-19 pandemic. Under our distribution-neutral growth assumption, this GDP reduction leads to an additional 410,000 poorpeople, with respect to the national poverty line of 28.9 dollars per person per day (in2011 PPP exchange rates). As we conservatively assume they are poor for one year only,this gure is directly equal to the number of poverty-years. The last row in Table 1αˆ provides the break-even ratios. In the case of Sweden, there are 9.4 times as manypoverty-years as lost-years. This means that the two sources of welfare costs wouldhave the same magnitude if 9.4 poverty-years were judged to be as bad as one lost-per-capita year. Finally, we provide thenumbers of lost-years and poverty-years, whichsimplies comparisons. In Sweden, the additional poverty corresponds to 0.0409 yearsper person while the number of lost-years corresponds to 0.0044 years per person.Table 1: Estimation of the pandemic's welfare costs in six countries as of early June2020 (baseline, distribution-neutral contraction)(1)(2)Economic and demographic charBaceltgeiruimsticSswedenGDP p.c. in 2017 (2011 PPP$)43,133 47,261National poverty line (2011 PPP$)2728.9Population (in millions)11.5910.10Life expectancy at birth81.1882.31Age (mean)41.4241.14Residual life expectancy (mean)42.0143.06(3) UK40,229 25.8 67.88 80.78 40.62 42.40(4) Pakistan4,764 2.8221.0 65.98 25.86 46.25(5) Peru12,517 5.332.98 80.24 32.53 50.55(6) Philippines7,581 2.6109.5 69.51 28.53 44.92Covid-19 mortality, current scenarioNumber of deaths9,605LYs per death9.467LYs per person0.007854,639 9.479 0.0043548,848 10.14 0.007302,056 18.46 0.0001725,465 21.97 0.003641,002 16.90 0.000155Covid-19 economic shockOn GDP per capita (in %) On poverty HC (in million) On poverty HCR-8.5 0.32 0.0279-11.5 0.41 0.0409-14.5 4.37 0.0644-6.7 7.39 0.0335-13.1 1.58 0.0480-8.4 2.96 0.0270Break-even αˆ3.5539.3838.816194.813.20174.8, The main nding of this section from an inspection of Table 1, is that the poverty costsare substantial relative to the mortality costs. This is obvious in the case of Pakistan and13αˆ the Philippines, for which the break-even are 195 and 175, respectively. It is relatively αˆ clear as well in the case of Peru, for which break-even is 13. If one were to take the view that α = 10, then Sweden and the United Kingdom would be very near the break-evenpoint at which the welfare costs of the pandemic arise in equal parts from greater povertyand mortality. Under that assumption (α = 10), Belgium (with αˆ = 3.6) would be theonly country in our sample for which Covid-induced mortality is clearly the dominant source of welfare losses from the current crisis. All things considered, it seems safe to say that, given individual and public policy responses, the poverty costs of the pandemic in these six countries are not dwarfed by its mortality costs. Indeed, for most plausible parameter estimates, the reverse is true (at least) in Pakistan and the Philippines.3.2 The whole worldThe ndings above suggest that the poverty eects of the pandemic are substantial, even in relation to its mortality eects. They also hint at a possible pattern where that cost ratio is much larger for developing countries relative to developed countries. To investigate those conjectures further we go beyond our six-country sample, and extend the analysis to as many countries as possible.Unfortunately, publicly available data on Covid-related deaths are not distributed by age-categories for most countries in the world, which prevents us from directly computing the number of lost-years, as we did in the previous subsection. In order to overcome this problem, we assume that country-specic infection probabilities are independent of age. Then, using estimates of Covid's age-specic infection-to-fatality ratios (IFR), we can infer the infection rate necessary to yield the number of deaths observed in the country, given its population pyramid. For developed countries, we use the age-specic IFRs estimated for France by Salje et al. (2020). For developing countries, we use analogous ratios estimated for China by Verity et al. (2020).20Formally, let Naj denote the size of the population of age a in country j, daj the number of Covid-related deaths at age a in country j, dj the total number of Covid-19 deaths in country j, and µaj the I F R at age a in country j. The proportion of people20The life expectancy at birth in China is larger than in many other developing countries. Thismay signal that, at a given age, Chinese citizens are aected by fewer health issues that increase the probability of dying from Covid-19 than their counterparts in the developing world. This may imply that the IFRs are smaller in China than in other developing countries. When distributing observed deaths across age-groups, the impact that larger IFRs in developing countries would have is a priori ambiguous, as this impact would depend on the relative increases of IFRs in dierent age-groups. However, given that the IFRs from France and China are not drastically dierent from one another and because we do not observe many Covid-induced deaths in these countries, we expect such impact on lost-years to be minor.14j who are or have already been infected by Covid-19 in country is estimated as:ϕj =dj99 a=0Najµaj.(2)a j The number of Covid-related deaths at age in country is given by:daj = ϕjNajµaj.(3)Our global estimates of the poverty impacts of Covid-19 come from Lakner et al. (2020). There are two methodological dierences between these estimates and those reported in Section 3.1. First, rather than using growth data from Central Banks, GDPGlobal estimates now come from the January and June 2020 editions of the World Bank's Economic Prospects (GEP) (The World Bank, 2020), supplemented with the April 2020 and October 2019 edition of IMF's World Economic Outlook (WEO) when countriesare not in the GEP (which mostly applies to high-income countries). As before, the extrapolation assumes distribution-neutral (negative) growth. Figure 2 below plots this dierence in forecasts the GDP shock due to Covid against GDP per capita for the 150 countries in our sample. We continue to assume that this additional poverty lasts only for a single year, so that the number of poverty-years is equal to this additional number of poor people.%0Burundi MozambiqGuaembia-5PakistanPhilippinesUBneitlegdiuKmingdom Sweden-10PeruZimbabweSeychelles-15Belize-20 500Maldives100020005000 10,000 20,000GDP per capita (PPP, constant 2011)GDP shock due to Covid-19 (in %)50,000 100,000Figure 2: Shock to GDP due to Covid-19 (baseline estimates)Second, instead of using national poverty thresholds, we use the World Bank's income class poverty thresholds, as derived by Jollie and Prydz (2016), namely $1.90 per person15per day in low-income countries (LICs); $3.20 a day in lower-middle-income countries (LMICs); $5.50 a day in upper-middle-income countries (UMICs); and $21.70 a day in high-income countries (HICs). These lines were obtained as the median national poverty lines for each income category in a dataset constructed by those authors.αˆ Figure 3 shows the break-even for all 150 countries in our global sample, plottedagainst GDP per capita. In order to average-out some of the measurement errors, we t a line through these ratios for each income-based group of countries: LICs, LMICs, UMICs, and HICs. Noting that both axes in Figure 3 are in logarithmic scale, we observe a very pronounced negative slope in the relationship, despite the fact that poverty lines increase between country categories. Naturally, the downward slope is even more pronounced when a constant poverty line is used, as shown in Figure A.9 in the Appendix, for theαˆ international poverty line of $1.90 per day. In fact, the tted break-even ratios forlow-income countries exceed 1,000, and, for middle-income countries, they are generallyα greater than 100. If we take seriously the interpretation of suggested earlier - a valueclose to the average answer that individuals would give as to how many years of their remaining life they would be willing to spend in poverty in order to increase their lifespanαˆ by one year - then these empirical estimates of the break-even are extremely large,suggesting that the welfare costs from poverty typically dominate the mortality costs in poor countries. They are also quite large in many high-income countries, though notuniformly so: about a third of the HICs have αˆ in the [1, 10] range.Break-even α̂11000,,000000 1000 100 10 10.1 500100020005000 10,000 20,000GDP per capita (PPP, constant 2011)1.9$ poverty line 3.2$ poverty line 5.5$ poverty line 21.7$ poverty line50,000 100,000Figure 3: αˆ Break-even in all countries as of early June 2020 (baseline, distribution-neutral contraction)This nding is further emphasized when considering less conservative estimates of the 16number of poverty-years generated by the pandemic. Our baseline estimates rely on the assumption that all incomes would shrink in the same proportion. Yet, the poor could be aected more than proportionally by the recession, in which case inequality would increase. The results of Bonavida Foschiatti and Gasparini (2020) for Argentina suggest that the recession generated by the response to the Covid-19 pandemic could increase the Gini coecient by 3.6%. In order to account for possible increases in inequality, we compute a second estimate of poverty-years that is based on a 3.6% increase in Gini coecients of all countries in 2020.21We also consider the poverty impact in case the pandemic requires more stringent and sustained lockdown measures. To this end, we utilize the fact that the GEP also provides a downside growth scenario, which precisely assumes a worse and longer recovery phase (see Figure A.8).22 Analogues of Figure 3 for each of these alternative poverty scenarios are shown in Figure A.10 in the Appendix.Overall, Figures 3 and A.10 conrm that, if the epidemic suddenly ended at the beginning of June 2020, its poverty costs would in most countries be very large relative to its mortality costs, and especially so in developing countries. Importantly, however, this nding does not mean that lockdowns were misguided, excessively long, or too strongly enforced. Our ndings so far do not allow any conclusions on the optimality of actual policy responses. They merely allow us to quantify dierent sources of the welfare impact of the crisis (the epidemic taken together with individual and policy responses), as it unfolded up to early June.There are two reasons why these results cannot be used to assess the policy response. First, dierent countries are still in dierent phases of the epidemic, and cross-countryαˆ comparisons of break-even must take that into account. Second, the observed outcomes(which incorporate the actual responses) must be compared to plausible counterfactuals corresponding to alternative policy responses, to assess their relative merits. This is what we turn to next, using a counterfactual no-intervention policy scenario as a comparator.4 Welfare costs under no-interventionIn this section, we compare the baseline estimates of welfare costs as of early June 2020, discussed above, with the potential mortality costs in a counterfactual no-intervention policy scenario. This allows us to shed some light on the question of whether nointervention could have been a superior policy response to what was observed until early21One challenge with modeling the impact of changes in Gini coecients is that there are innitelymany possible distributional changes resulting in the same change in the Gini. Using the framework of Lakner et al. (2020), we assume that inequality increases in a manner consistent with a linear growth incidence curve, approximating what was found in Argentina.22For most high-income countries, for which we are using WEO forecasts rather than GEP, there isonly one GDP scenario, so the baseline and downside scenarios coincide.17June 2020. A no-intervention scenario implies that the epidemic only stops in a country onceherd-immunity is achieved. We follow Banerjee et al. (2020) who consider that herdimmunity is achieved when 80% of the population has been infected by the virus.23 Under this scenario, all countries have the same infection rate, which allows comparing their potential mortality costs. We err on the side of caution by assuming that the poverty costs in the no-intervention scenario are zero. This is a conservative assumption since, even in the absence of lockdowns or other policy-driven measures, aversion behavior by individual workers and consumers is nonetheless likely to have led to non-trivial economic contractions (Sheridan et al., 2020).24 From Equation (1), we have that the actual welfareconsequences ∆W A would be equal to the no-intervention welfare consequences ∆W N IwhenαLY A + P Y A = αLY NI + P Y NI .P Y As we assume zero economic consequences under our no-intervention scenario (NI=0), this yields a second, dierent threshold value for the α parameter, namely α˜:PY A α˜ = LY NI − LY A This is the value of α at which, given the estimated magnitudes of LY A, P Y A, and LY N I , the counterfactual welfare costs of mortality under the no-intervention scenario would equal the actual costs, αLY A + P Y A. For any α > α˜, the welfare consequencesunder the no-intervention scenario would have been worse than those estimated for theα˜ actual outcome.25 Again, we do not interpret the empirical of a given country as an α˜ estimate of this country's preference parameter. Instead, we present these such thatthe reader can form an opinion as to whether no-intervention would have led, in her ownview, to worse welfare consequences.4.1 Heterogenous mortality under no-interventionIn this section, we perform a cross-country comparison of potential mortality burdensLY N I under the no-intervention scenario. This exercise reveals large quantitative dier-ences in the potential number of lost-years between developing and developed countries.23We also investigate the robustness of our results under lower infection rates. 24Under this clearly extreme assumption of a zero poverty eect, this no-intervention scenariocan eectively be interpreted as a no-response scenario: neither governments nor individual rms or consumers adjust their behavior to the pandemic. It is an evidently unrealistic scenario, useful only to generate the counterfactual mortality costs as a clear underestimate of the total welfare costs of a pure herd-immunity strategy.25It should be evident that α˜ is completely dierent from αˆ, for any given country. In particular, α˜depends on counterfactual estimates of mortality under no-intervention in that country.18We compute a country's potential number of lost-years under herd-immunity as follows. We assume an 80 % infection rate for all age categories of the population.26 The number of infected individuals in each age-category is computed from the country's population pyramid. The probability that an infected individual of a given age dies is deduced from age-specic infection-to-fatality ratios (IFR), once again using the IFR estimates for China from Verity et al. (2020) for developing countries, and those for France from Salje et al. (2020) for developed countries.27 Then, the number of lost-years for a given death is the country's residual life expectancy at the age of death. Summing the lost-years over all deaths provides the potential number of lost-years.We consider two no-intervention scenarios. Under the saturation scenario, we assume that contagion is fast and hospitals are overwhelmed by the ow of infected individuals in need of care. In order to reect this, the IFRs used assumes that all patients who would need intensive care cannot access ICU and die. This is our reference no-intervention scenario. Under the no-saturation scenario, we assume that contagion is slow and hospitals are not overwhelmed by the ow of infected individuals in need of care. Then, the IFRs used assume that all patients who would need intensive care have access to ICU. See Appendix A2 for more details.Our estimates of the potential number of lost-years can dier across countries for three reasons: (i) age distributions; (ii) residual life expectancies; and (iii) IFRs used. We nd that the potential mortality burden is several times larger in high-income countries than in low-income countries. This is despite the use of IFRs from China in the latter countries, which implies more lost-years than the IFRs from France would. To a large extent, this dierence is the consequence of the concentration of Covid-deaths in oldage categories, as already illustrated in Section 3.1. Hence, younger populations in less developed countries reduce their potential mortality burden. An additional, but related, reason for this dierence is that residual life expectancies at given ages are smaller in less developed countries.Table 2 illustrates the heterogeneous mortality burdens for two countries: Japan and Zimbabwe. The population of Japan is considerably older than that of Zimbabwe. For instance, a third of Japan's population is older than 60 years, whereas less than 5% of Zimbabwe's population falls into that category. Residual life expectancy in Japan is larger than that of Zimbabwe in each age-category presented. For instance, individuals26Assuming a constant infection rate for everyone is a strong assumption because infection rates areexpected to vary by age and by socio-economic status (Lustig et al., 2020). In the conclusion, we discuss this assumption, its impact on our results and argue that further research on the topic should try to relax this assumption, once the necessary evidence is available.27As noted earlier, it is possible that the IFRs are smaller in China than in other developing coun-tries. If this is the case, then we underestimate the number of lost-years under no-intervention in developing countries, and, as a consequence, underestimate the number of developing countries in which no-intervention was a dominated option. However, given that the IFRs from France and China are not drastically dierent from one another, we expect such impact on lost-years under no intervention to be limited.19on average in the 60+ age category can expectto live for 16 years in Japan, and only for11 years in Zimbabwe.Table 2: Mortality risks in Japan and ZimbabwePopulation per age group (%)(1)(2)Japan Zimbabwe0-29 30-59 60+26.6 39.0 34.469.5 25.8 4.69Residual life-expectancy per age group0-29 30-59 60+69.1 40.1 15.652.7 28.8 11.2Covid-19 LYs per personNo intervention and no saturation No intervention and saturation0.102 0.2040.0212 0.0298Moving beyond that illustrative two-country example, Figure 4 plots years of lifeLY lost to Covid-19 under the no-intervention scenarios ( N I per person) - both with andwithout saturation - against GDP per capita for all countries in our sample. Notice that once again both axes are in logarithmic scale. The gure shows that potential mortality burdens are several times larger in high-income countries than in low-income countries. Under the saturation scenario, a large fraction of high-income countries has a per capita number of lost-years above 0.1 years per person. This is in sharp contrast with low-income countries, whose per capita number of lost-years is always below 0.05 years per person under the same scenario. In particular, the number is 0.2 years per person in Japan and 0.03 years per person in Zimbabwe, implying that the potential mortality burden in Japan is more than six times larger. Strikingly, high-income countries still have considerably larger potential mortality burdens under the no-saturation scenario than low-income countries under the saturation scenario. In particular, the number of lost-years per person is more than three times larger in Japan under the no-saturation scenario than in Zimbabwe under the saturation scenario.Another striking aspect of Figure 4 is the heterogeneity of mortality burdens even among countries with similar levels of GDP per capita. Above $5,000 per capita, one country can have more than twice the mortality burden of another country with similar GDP per capita. This illustrates the roles that population pyramids and residual life expectancies play in shaping Covid-19 mortality, quite separately from pure income considerations.20Figure 4: Per capita number of lost-years under two no-intervention scenariosWe also observe that the size of potential mortality burdens seems limited. Theselimited burdens follow from the small fraction of infected people who eventually die(typically smaller than 1% (Salje et al., 2020)) and the high concentration of deathsamong the very old. In the case of Japan, whose population is particularly old, thepotential number of lost-years under saturation corresponds to less than 0.4% of thetotal number of years at stake (the population multiplied by its average residual lifeexpectancy). This explains why economic losses may be a signicant source of welfarecosts in this pandemic.Finally, recall that these mortality comparisons are based on a policy scenario implyingsimilar infection rates in all countries. At this stage, many alternative scenarios are stillrealized possible, and we do not speculate on which are more likely. Yet,mortality tollscould still be larger in some low- and middle-income countries than in some high-incomecountries. This would for instance be the case if the latter are able to stop the epidemicat current infection rates while the former are unable to do so.4.2 Actual versus no-intervention welfare consequencesFinally, we turn to the comparison between the actual and no-intervention welfare con-sequences. As explained above, the latter are larger than the former if α > α˜.The rst nding of this section is that, except for a few very poor countries, the welfareworse consequences under our no-intervention scenario are greater (i.e.) than the actual21α welfare losses as of early June, for any plausible .28 This implies that doing nothingwas a dominated policy response in many countries, at least if the epidemic progressively disappears after early June and if Covid-induced poverty lasts for one year only.α˜ This nding ensues from Figure 5, which shows break-even under saturation mor-tality and with poverty computed for income class-specic poverty thresholds, as before. The gure uses the baseline growth estimates and the distribution-neutral growth as-α˜ sumption. The graph shows that break-even are less than one in almost all developed α and in most developing countries. But, as we saw in Section 2, is theoretically bounded 1 below at , so the estimated welfare outcomes are worse under no-intervention for alladmissible normative criteria.1010.1Break-even α̃500 1000 20005000 10,000 20,00050,000 100,000GDP per capita (PPP, constant 2011)1.9$ poverty line 3.2$ poverty line 5.5$ poverty line 21.7$ poverty lineFigure 5: α˜ Break-even in all countries as of early June 2020 (baseline, distribution-neutral contraction, no-intervention scenario with saturation, group-specic povertythresholds)in developed countries We argue that the sub-optimality of no-interventionis robust totaking less conservative poverty estimates. First and foremost, we assume conservativelythat the no-intervention scenario has zero poverty costs, which is implausible. Second, ifwe relax our assumption that additional poverty only lasts for one year, and assume thatthe current poverty costs are in fact two or three times larger than our estimates, the resultstill holds for developed countries. Indeed, as shown in Table 3, the median break-evenα˜ for this group of countries is never larger than one-third under the various scenarios α˜ considered. In the case of upper-middle income countries, the median break-even isnever greater than one under the various scenarios considered, which also suggests sub-28Recall that α > 1.22optimality of no-intervention for these countries. Lastly, this sub-optimality is also largely robust to assuming smaller infection rates under no-intervention than 80% (as suggested by Banerjee et al. (2020)). Under smaller infection rates, the mortality consequencesα˜ of no-intervention are smaller and therefore break-even values are larger. Even if theinfection rate leading to herd immunity varies across populations and periods (Randolph and Barreiro, 2020), this threshold infection rate is not expected to be lower than 50%α˜ (Fontanet and Cauchemez, 2020).29 Figure A.11 in Appendix shows the break-evenvalues under an infection rate of 50%. This smaller infection rate does make a dierence:α˜ about half of the low-income countries have their break-even values above one and itis also the case for a non-negligible minority of middle-income countries, as well as three developed countries. However, this does not necessarily imply that no-intervention wouldα˜ have been better for these countries. First, their break-even values are almost always α below two or three, which could very well be below the reader's value. Second, these α˜ break-even values assume zero poverty consequences in the no-intervention scenario, α˜ which articially increases break-even values. Finally, the vast majority of developed α˜ countries still have their break-even values below one-half, implying for these countriesthat no-intervention would have been dominated.Table 3: α˜ Lives and livelihoods - break-even as of early June 2020, under no interven-tion scenario and saturation, medians by World Bank (WB) income groups(1)(2)(3)(4)(5)Median of economic and demographiLcIC characLtM erIiCsticsUMIC HIC WorldGDP p.c. in 2017 (2011 PPP$) Residual life expectancy1,697 47.825,481 46.4713,822 45.1135,938 41.8611,676 45.12Median of Covid-19 mortality, no intervention and saturationDeaths per capita LYs per death LYs per person0.00169 17.77 0.03020.00289 16.10 0.04450.00503 14.97 0.07620.0113 11.89 0.1280.00434 14.95 0.0655Median shock on poverty HCRPoverty line (median) Baseline scenario, distribution-neutral Baseline scenario, +3.6% in Gini1.9 0.019 0.0363.2 0.021 0.03555.5 0.021 0.04321.7 0.028 0.0405.5 0.023 0.037Median break-even α˜, no intervention and saturationBaseline scenario, distribution-neutral 0.6090.4910.323Baseline scenario, +3.6% in Gini1.0890.8690.6420.259 0.3540.387 0.64629The infection rate leading to herd immunity depends on preventive behaviors, e.g., use of masksor social distancing practices. Our no-intervention scenario should account for typical behavior at the outbreak of the pandemic, when pre-pandemic behavior was still largely the norm. With less preventive behaviors, the threshold infection rate is expected to be larger.23Yet, we cannot rule out no-intervention as a plausible policy in some low-incomeα˜ countries. Their median break-even is slightly larger than one under one of the twoscenarios shown in Table 3 (under our baseline 80% infection rate). This is the scenario in which we allow inequality to increase, which leads to higher poverty costs of theα˜ pandemic and therefore larger break-even values. At least some low-income countries have break-even α˜ larger than two (see Figure 5). For values of α˜ in that range, thewelfare comparison in the poorest countries remains theoretically ambiguous, although only for observers placing a low normative value on an additional year of life relative to a year in poverty.Given an observer's xed normative choice of α, the lower α˜ the greater the welfarelosses associated with the no-intervention scenario, relative to the actual early June estimates. The downward-sloping relationship in Figure 5 thus indicates that the welfare losses from policy inaction relative to the responses observed until early June 2020 increase sharply with GDP per capita. This eect is emphasized when using a common poverty line for all countries, such as the international extreme poverty line (IPL) of $1.90α˜ a day (Ferreira et al., 2016). Figure 6 provides a scatter plot of for all countries usingthe IPL. The slope of the tted line is very steep. When using extreme poverty in allα˜ countries, we obtain values well below 0.1 in high-income countries.30 This is becauseeven in the case of a deep recession, high-income countries would have very little extreme poverty, because incomes are far above the extreme threshold.31This nding suggests that a country's optimal intervention is likely to dier as a function of its development level. For a given rate of infection and negative GDP shock, the relative sizes of the two sources of welfare consequences vary greatly with GDP per capita. On average, the more developed the country, the larger are the mortality costs and the smaller are the poverty costs. We have shown that these dierences are quantitatively large. This implies that best policy responses might be more targeted towards containing infections in developed countries and towards containing poverty in developing countries - even though we treat the value of a year of human life as identical across countries throughout.Table 4 summarizes our main estimates for the four income categories of countries and for the world, under our dierent scenarios. These estimates are the building blocksbehind our break-even αˆ and α˜. The table reiterates the point that for LICs, PYs areon average of the same magnitude as LYs in all no-intervention scenarios, while for HICs LYs dominate in the no-intervention scenarios or if considering the frugal $1.90 poverty line. For the world as a whole, PYs dominate greatly in the current scenario but are surpassed by LYs in both no intervention scenarios. Table 4 also provides a sense of30Even when using the $3.2 a day or $5.5 a day lines instead of the extreme line, break-even α˜ valuesremain below 0.1 in high-income countries. See Figure A.13 in the Appendix.31Even assuming a 50% infection rate under no-intervention, break-even α˜ values remain below 0.1 inhigh-income countries. See Figure A.12 in the Appendix.2410Zimbabwe Sierra LeoneTimor-Leste1Philippines PakistanPeru0.1Break-even ̃αUnited Kingdom500 1000 20005000 10,000 20,00050,000 100,000GDP per capita (PPP, constant 2011)1.9$ poverty lineFigure 6: α˜ Break-even in all countries as of early June 2020 (baseline, distribution-neutral contraction, no-intervention scenario with saturation, extreme poverty threshold)the additional increases in poverty likely to arise if the pandemic-reduced recession is inequality-increasing, instead of distribution neutral. Applying a stylized "Argentina" growth incidence curve across all countries, as described above, adds 62 million PYs when the IPL is used for all countries, and 133 million PYs when the income-class specic "WB classication" poverty lines are used.325 Concluding remarksThe Covid-19 pandemic has generated huge losses in well-being around the world, by increasing mortality, causing ill-health and suering, closing schools, etc. In combination with individual and policy responses, the pandemic has also generated a large global negative economic shock, with GDP declines currently expected to range from 4.8% on average in low-income countries to 8.9% on average in high-income countries. These marked economic contractions are causing substantial increases in poverty, reversing at least temporarily a hitherto sustained trend of global poverty decline which had been in place since 2000.In this paper we focus on the mortality and poverty costs of the pandemic. We propose a simple framework to conduct a welfare evaluation of those costs, relying on a comparison of the number of years lost to Covid-19 deaths (lost-years) with the number32These conclusions are qualitatively unchanged when using the downside growth scenario (resultsavailable upon request).25Table 4: Lives and livelihoods - pandemic's aggregate eects on mortality and poverty(Current = early June 2020)(1)(2)(3)Total number of Covid-19 deatLhIsC, in mLiM lliIoCn UMICCurrent scenario0.000773 0.0171 0.0892No intervention, no saturation1.0159.396 14.82No intervention, saturation1.15910.23 15.61(4) HIC0.288 7.387 13.28(5) World0.396 32.66 40.32Total increase in lost-years, in millionCurrent scenario No intervention, no saturation No intervention, saturation0.0113 14.73 20.590.221 121.4 153.71.217 195.9 227.82.875 73.75 157.94.325 406.4 560.7Total increase in poverty-years, in millionDistribution-neutral scenario1.9 PPP-$ poverty line13.7447.12WB classication poverty line13.74138.7+3.6% in Gini scenario1.9 PPP-$ poverty line21.0091.58WB classication poverty line21.00196.46.780 53.900.235 28.4668.22 234.816.43 106.50.569 44.44130.5 368.3of additional person-years spent in poverty in 2020. Drawing on a rich combination of data sources on Covid-19 mortality; demographic structures and age distributions; and on income distributions for 150 countries in the world, we estimate that the pandemic had generated 4.3 million lost years and as many as 68.2 million additional poverty yearsglobally (using the extremely frugal international poverty line of 1.90 per day) by earlyJune 2020. If median poverty lines are used for LICs, LMICs, UMICs and HICs, we estimate that 235 million poverty-years are being generated this year.Across countries, the poverty-years to lost-years ratio ranges from just above 1 to more than 10,000. It is 3.6 in Belgium and 195 in Pakistan, for example. We document a strong association between this ratio and GDP per capita, with poverty costs being systematically larger in poorer than in richer countries, both in absolute terms and relative to mortality costs. These results are for our baseline scenario, which conservatively assumes a distribution-neutral allocation of the income losses associated with the declines in GDP. Poverty costs are even greater in an alternative scenario where we allow for some increase in inequality, based on the pattern estimated for Argentina by Bonavida Foschiatti and Gasparini (2020). For most developing countries, it is dicult then to avoid the conclusion that one would have to place a very low weight on the welfare cost of falling into poverty to conclude that the mortality costs of the pandemic exceed its poverty costs.26not It is important to note that this doesmean that public interventions aimed atcontaining the spread of the virus have been a cure worse than the disease. Indeed weshow that, for most countries, the absence of any non-pharmaceutical interventions wouldhave led to mortality costs which exceed, on their own, the total welfare losses accruedα as of early June, for any plausible value of . Thus, we must conclude that, for the vastmajority of countries, no-intervention was a dominated response. Interventions were suc-cessful in slowing the spread of the disease and, even if they led to increases in poverty,welfare losses were substantially lower than under no-intervention. As with our estimatesfor early June, the mortality costs of the pandemic dier markedly between developedand developing countries in the counterfactual, no-intervention scenario. For given in-fection rates, developed countries face mortality costs several times higher than those ofdeveloping countries, because their populations are considerably older, and because theyhave longer residual life expectancies at given ages.Taken together, the evidence we present makes it dicult to escape the conclusionthat the optimal policy responses to the pandemic cannot be one size ts all. Responsesshould almost certainly dier across countries, with policymakers in poorer countriesbeing justiably more concerned with the poverty costs faced by their populations thanthose in richer countries. That said, we have not sought to identify the optimal positioncountries should take when facing policy trade-os. We do not even believe that thereis necessarilya trade-o between lives and livelihoods for every policy response toCovid-19. Developing a vaccine, for example, would clearly reduce both lost-years andpoverty-years in the future. The same is likely true for early contact-tracing and large-scale testing. Imposing social-distancing early likely dominates doing it late (Demirguc-Kunt et al., 2020), and so on. Optimal policy choices require examining these trade-osat the level of the individual policy, in a manner informed both by the demographic andsocio-economic context, and by the set of other policy options available to the government.What we hope to have contributed is a simple and transparent approach to esti-mating the relative welfare costs of Covid-induced increases in mortality and poverty.This approach may also be relevant for other economic problems that involve similarlives-versus-livelihoods trade-os. For instance, assessments of the welfare costs of pastpandemics mostly relies on methods attributing a monetary value to human life (Mar-tin and Pindyck, 2019). Also, well-being comparisons across countries and time, whichinvolve accounting for the quality and quantity of life, are typically based on expectedlifetime utility (Becker et al., 2005; Jones and Klenow, 2016). Cost-benet analysis ofenvironmental projects is regularly performed on the basis of a monetary value to humanlife (Robinson et al., 2019; Agency, 2014). Another major eld where this trade-o ispervasive is health economics, where cost-benet analysis of drugs and policies are alsobased on a monetary value to human life (Hausman, 2015). In the latter case, the healthimpact of diseases is typically measured based on quality-adjusted life-years (QALYs), a27concept that takes into account not only the length of life but also health losses incurred by those surviving these diseases (Whitehead and Ali, 2010), physical losses or mental losses (Chisholm et al., 1997). Our methodology could readily be adapted in order to account for these costs by replacing lost-years by QALYs.That said, there are also many ways in which our analysis can be improved in future research. Our estimates of lost-years and poverty-years are based on several strong assumptions that further research should try to relax, once the required evidence and data becomes available. For instance, our estimates of poverty-years should be rened for the countries that enacted substantial social assistance programs.33 Also, our estimates of lost-years under no-intervention assume a constant infection rate across age categories. However, given the larger IFRs aecting the elderly, one might expect these individuals to adopt more cautious behavior and, therefore, observe lower infection rates in their age categories. In that case, herd-immunity could be reached with fewer deaths, implying that our estimates of lost-years are too high. Alternatively, infection rates might also be higher among the elderly if their immune system is less ecient at protecting them when they are in contact with an infected individual. Reviewing the early literature on the topic, Biswas et al. (2020) do not nd signicantly dierent risk of infection between individuals younger than 50 years and individuals older than 50 years. More generally, we ignore many important questions that may arise in the presence of a trade-o between lives and livelihoods. For instance, if the additional poverty-years are mostly concentrated among the young while the lost years of life are mostly concentrated among seniors, this may spark debates around inter-generational justice that are not tackled in this paper. These and other questions will certainly warrant renements to some of our assumptions and modeling choices, as better data about this pandemic becomes available.ReferencesAcemoglu, D., V. Chernozhukov, I. Werning, and M. D. Whinston (2020). A Multi-RiskSIR Model with Optimally Targeted Lockdown. NBER, Working Paper 27102 .Adler, M. D., R. Bradley, M. Ferranna, M. Fleurbaey, J. Hammitt, and A. Voorhoeve(2020). Assessing the wellbeing impacts of the covid-19 pandemic and three policyMimeo types: Suppression, control, and uncontrolled spread..Agency, U. E. P. (2014). U.s. environmental protection agency: Guidelines for preparingeconomic analyses. U.S. Environmental Protection Agency Report.33Lustig et al. (2020) nd early evidence of considerable heterogeneity in the impacts of changesin social protection programs implemented in Argentina, Brazil, Colombia and Mexico during 2020 in response to the pandemic.28Alon, T., M. Kim, D. Lagakos, and M. VanVuren (2020). How should policy responsesto the Covid-19 pandemic dier in the developing world? CEPR, Covid Economics, Issue 15 .Baland, J., G. Cassan, and B. Decerf (2020). Too young to die. deprivation measurescombining poverty and premature mortality. American Economic Journal: Applied Economics Forthcoming.Banerjee, A., L. Pasea, S. Harris, G.-I. A., T. A., L. Shallcross, and C. Pagel (2020).Estimating excess 1-year mortality associated with the covid-19 pandemic accordingThe Lancet to underlying conditions and age: A population-based cohort study..Becker, G. S., T. J. Philipson, and R. R. Soares (2005). The Quantity and Quality ofLife and the Evolution of World Inequality. The American Economic Review 95 (1),277291.Bethune, Z. and A. Korinek (2020). Covid-19 Infection Externalities: Trading O Livesvs. Livelihoods. NBER, Working Paper 27009 .Biswas, M., S. Rahaman, T. K. Biswas, Z. Haque, and B. Ibrahim (2020). Eects of sex,age and comorbidities on the risk of infection and death associated with covid-19: ASSRN 3566146 meta-analysis of 47807 conrmed cases..Bonavida Foschiatti, C. and L. Gasparini (2020). El Impacto Asimetrico de la Cuarentena.Documentos de Trabajo del CEDLAS N. 261. CEDLAS-Universidad Nacional de La Plata.Social Chisholm, D., A. Healey, and M. Knapp (1997). Qalys and mental health care. Psychiatry and Psychiatric Epidemiology 32 (2), 6875.Demirguc-Kunt, A., M. Lokshin, and I. Torre (2020). The Sooner, the Better: The EarlyEconomic Impact of Non-Pharmaceutical Interventions during the Covid-19 Pandemic.World Bank Policy Research Working Paper 9257 .Dicker, D., G. Nguyen, D. Abate, K. H. Abate, S. M. Abay, C. Abbafati, N. Abbasi,H. Abbastabar, F. Abd-Allah, J. Abdela, et al. (2018). Global, regional, and nationalage-sex-specic mortality and life expectancy, 19502017: A systematic analysis forThe Lancet 392 the global burden of disease study 2017.(10159), 16841735.Eichenbaum, M. S., A. Atkeson, G. Barlevy, F. Ciocchini, and W. Cornwall (2020). TheMacroeconomics of Epidemics. NBER Working Paper .Ferreira, F. H., S. Chen, A. Dabalen, Y. Dikhanov, N. Hamadeh, D. Jollie, A. Narayan, E. B. Prydz, A. Revenga, P. Sangraula, U. Serajuddin, and N. Yoshida (2016). A29global count of the extreme poor in 2012: Data issues, methodology and initial results.Journal of Economic Inequality 14 (2), 141172. Nature Fontanet, A. and S. Cauchemez (2020). Covid-19 herd immunity: Where are we?Reviews Immunology, 12.Garibaldi, P., E. R. Moen, and C. A. Pissarides (2020). Modelling contacts and transitionsin the sir epidemics model. Covid Economics Vetted and Real-Time Papers, CEPR. EconFIP Policy Gourinchas, P. O. (2020). Flattening the pandemic and recession curves.Brief 23..Hall, R. E., C. I. Jones, and P. J. Klenow (2020). Trading o consumption and covid-19deaths. Stanford University Working Paper. Hausman, D. M. (2015). Valuing health: Well-being, freedom, and suering. OxfordUniversity Press.Jollie, D. and E. B. Prydz (2016). Estimating international poverty lines from compa-rable national thresholds. The World Bank .Jones, C. I. and P. J. Klenow (2016). Beyond GDP? Welfare across countries and time.American Economic Review 106 (9), 24262457.Kikuchi, S., S. Kitao, and M. Mikoshiba (2020). Who Suers from the COVID-19 Shocks?CEPR, Covid Eco- Labor Market Heterogeneity and Welfare Consequences in Japan. nomics, Issue 40 .Laborde, D., W. Martin, and R. Vos (2020). Poverty and food insecurity could growdramatically as covid-19 spreads. International Food Policy Research Institute (IFPRI), Washington, DC .Lakner, C., D. G. Mahler, M. Negre, and E. B. Prydz (2020). How much does reducing inequality matter for global poverty? global poverty monitoring technical note 13.Lustig, N., V. M. Pabon, F. Sanz, and S. D. Younger (2020). The impact of covid19 lockdowns and expanded social assistance on inequality, poverty and mobility inargentina, brazil, colombia and mexico. CEQ Working Paper 92.Martin, I. W. and R. S. Pindyck (2019). Welfare costs of catastrophes: Lost consumptionand lost lives. National Bureau of Economic Research 26068.Modrek, S., D. Stuckler, M. McKee, M. R. Cullen, and S. Basu (2013). A review of health consequences of recessions internationally and a synthesis of the us response during thegreat recession. Public Health Reviews 35 (1), 10.30Montenovo, L., X. Jiang, F. Lozano Rojas, I. M. Schmutte, K. I. Simon, B. A. Weinberg,NBER, and C. Wing (2020). Determinants of Disparities in Covid-19 Job Losses. Working Paper 27132 .NBER, Pindyck, R. S. (2020). COVID-19 and the Welfare Eects of Reducing Contagion. Working Paper 27121 .The Journals Promislow, D. E. (2020). A geroscience perspective on covid-19 mortality. of Gerontology: Series A.Randolph, H. E. and L. B. Barreiro (2020). Herd immunity: Understanding covid-19.Immunity 52 (5), 737741.Indian Eco- Ray, D. and S. Subramanian (2020). India's lockdown: an interim report. nomic Review 55 (1), 8181.Robinson, L. A., J. K. Hammitt, and L. O'Keee (2019). Valuing mortality risk reductionsin global benet-cost analysis. Journal of Benet-Cost Analysis 10(S1), 1550.Rowthorn, R. and J. Maciejowski (2020). A costbenet analysis of the covid-19 disease.Oxford Review of Economic Policy 36, S38S55.Salje, H., C. T. Kiem, N. Lefrancq, N. Courtejoie, P. Bosetti, J. Paireau, A. Andronico,N. Hoze, J. Richet, C.-L. Dubost, et al. (2020). Estimating the burden of sars-cov-2 infrance. Science.Sheridan, A., A. L. Andersen, E. T. Hansen, and N. Johannesen (2020). Social distancinglaws cause only small losses of economic activity during the covid-19 pandemic inscandinavia. Proceedings of the National Academy of Sciences 117 (34), 2046820473.Sumner, A., C. Hoy, and E. Ortiz-Juarez (2020). Estimates of the impact of covid-19 onglobal poverty. UNU-WIDER Working Paper 43.Tapia Granados, J. A. and E. L. Iolides (2017). Population health and the economy:Health economics 26 Mortality and the great recession in europe.(12), 219235.The World Bank (2020). Global Economic Prospects. Washington, DC: The World Bank.Verity, R., L. C. Okell, I. Dorigatti, P. Winskill, C. Whittaker, N. Imai, G. Cuomo-Dannenburg, H. Thompson, P. G. Walker, H. Fu, et al. (2020). Estimates of theThe Lancet Infectious severity of coronavirus disease 2019: a model-based analysis. Diseases .Journal of Economic Viscusi, W. K. (1993). The value of risks to life and health. Literature 31 (4), 19121946.31Whitehead, S. J. and S. Ali (2010). Health outcomes in economic evaluation: The qalyand utilities. British Medical Bulletin 96 (1), 521.32A AppendixA.1 Data sources33Table A.1: Data sources34Variables Population per age groupSourcesTransformationGlobal Burden of Disease (Dicker et al., 2018) UN population divisionMissing data imputed using IHME data Smoothing using 5-year moving averageCovid-19 mortalityOur World in Data (7/6/2020)Covid-19 mortality per age group Belgium Sweden England and Wales Scotland Northern Ireland Pakistan Peru Philippines United KingdomAll countriesSciensano (7/6/2020) Statista (5/6/2020) Oce for National Statistics (22/5/2020) National Records of Scotland (31/5/2020) Department of Health (6/6/2020) http://covid.gov.pk (8/6/2020) Ministry of Health (7/6/2020) Department of Health (7/6/2020) Authors' estimatesAuthors' estimatesSmoothing using 9-year moving average Smoothing using 9-year moving averageSmoothing following data from England and Wales Smoothing following data from England and Wales Smoothing using 9-year moving average Smoothing using 9-year moving average Smoothing using 9-year moving average Aggregation of data from constituent countries Smoothing using 5-year moving average See section A.2Infection to Fatality Ratio (IFR) IFR in LIC and MIC IFR in HIC IFR if health care saturationVerity et al. (2020) Salje et al. (2020) Authors' estimatesExponential t (see Section A.2) Exponential t (see Section A.2) Maximum of P(ICU|infected) and IFRsMortality from other causes in 2017 Global Burden of Disease (Dicker et al., 2018)GDP per capita in 2011 PPP$World Bank, World Development IndicatorsGDP growth forecast Belgium Sweden United Kingdom LIC and MIC HICNational Bank of Belgium Sveriges Riksbank (Central Bank of Sweden) Bank of England World Bank, Global Economic Prospects IMF, World Economic OutlookPoverty forecastPovcalNet and Lakner et al. (2020)A.2 VariablesData sources are described in table A.1. The following transformations were applied to the data.Population by age The population data from the UN population division are orga-nized in 5-year categories. The population data from the IHME are also organized in 5-year categories, except the category 0-4 which is split in two (0-1 and 1-4). For each country, we rst split the population of the age category 0-4 into two sub-categories following the same distribution as the 0-1 and 1-4 categories in the IHME data. We smooth the resulting data using 5-year moving averages. The results are shown in gure A.1 for Japan and Zimbabwe. Smoothing is expected to lead to more precise results. For Belgium, for example, the correlation between the 5-year moving average and population by age from STATBEL is slightly larger (0.997) than the correlation between the original data and STATBEL population by age (0.994).# #2000000 1500000 1000000 5000000 020406080100AgePopulation per age group Population per age, 5-year moving average400000 300000 200000 1000000 020406080100AgePopulation per age group Population per age, 5-year moving average(a) Japan(b) ZimbabweFigure A.1: Age pyramids for Japan and Zimbabwe.Mortality by age We use data on population and number of deaths by age categoryfrom the Institute of Health Metrics and Evaluation. The most recent data are for the year 2017. We rst smooth the two variables using 5-year moving averages. We then calculate the mortality by age as the ratio of the number of deaths by age (moving average) to the population by age (moving average). The results are shown in gure A.2 for Japan and Zimbabwe.Residual life expectancy by age m The maximal age in our data is 99. We denote ajthe probability that an individual of age a in country j has of dying within a year and λajits residual life expectancy. For each country, we calculate the residual life expectancy at99 as 1/m99,j . We calculate the median value of the estimates obtained for each country35.4.3P(dying).2.10020406080100AgeJapan, mortality per age (IHME data) Japan, 5-year moving average Zimbabwe, mortality per age (IHME data) Zimbabwe, 5-year moving averageFigure A.2: Mortality by age for Japan and Zimbabweand assume that the result - 2.9 years - is the residual life expectancy at 99 for all countries.We then calculate λaj backwards for all ages, as λaj = 0.5maj + (1 + λa+1,j )(1 − maj ).The life expectancy at age a is given by laj = a + λaj . The residual life expectancy anda life expectancy at age are shown in gure 3(a) for Japan and Zimbabwe. The averageresidual life expectancy in country j is given by l¯j =99 a=0Najλaj/Nj,whereNajisthesize of the population of age a in country j and Nj is the total population of country j.Infection Fatality Rate (IFR) For high-income countries, we use IFR estimatesfrom Salje et al. (2020), who analyzed data on Covid-19 mortality from France. For low-income and middle-income countries, we use IFR estimates from Verity et al. (2020), who analyzed data on Covid-19 mortality from China. Both IFR estimates are provided for 10-year age categories below 80 years old and then one 80+ residual category. The literature on Covid-19 suggests that Covid-19 mortality is increasing exponentially with age (Promislow, 2020). We therefore smooth the IFR estimates of Verity et al. (2020) and Salje et al. (2020) using an exponential t. Results are shown in gure A.4. For the no-intervention scenario leading to herd immunity, it is likely that the IFR would be higher than the estimates of Verity et al. (2020) and Salje et al. (2020) because health care systems would be saturated. For this no-intervention scenario with saturation of health care systems, we construct a higher-bound IFR by assuming that all infected individuals needing intensive care die. For this purpose, we use the data of Salje et al. (2020) and multiply the probability to be hospitalized if infected by the probability to go to intensive care if hospitalized. We smooth the series using an exponential t. When this higherbound IFR is lower than the IFR estimated by Verity et al. (2020) and Salje et al. (2020), we consider the maximum of the values.36E(years)806040200020406080100AgeJapanZimbabwe(a) Residual life expectancy by ageYears5550454035 020000400006000080000GDP per capita (PPP, constant 2011)100000(b) Average residual life expectancy100909080Years807070 60020406080100AgeJapanZimbabwe6050 020000400006000080000GDP per capita (PPP, constant 2011)(c) Life expectancy by age(d) Life expectancy at birthFigure A.3: Residual life expectancy and life expectancy.100000E(age)IFR.4 .5.3.4IFR.3 .2.2.1 .100020406080100020406080100AgeAgeOriginal Exponential fitOriginal Exponential fit(a) IFR from (Salje et al., 2020)(b) IFR from (Verity et al., 2020)Figure A.4: IFR estimates in China (Verity et al., 2020) and France (Verity et al., 2020).Covid-19 deaths by age For Belgium, Sweden, the United Kingdom (UK), Pakistan,Peru, and the Philippines, we have data on Covid-19 deaths by age category from early June 2020. We use a 5-year moving average to smooth the UK data, which are organized in 5-year categories. For other countries, categories are of 10 years or larger. We use379-year moving averages to smooth the data. Results are shown in gure A.5.For other countries, we estimate Covid-19 deaths by age by exploiting the IFR es-timates and by assuming that the probability of Covid-19 infection is independent ofd age. We consider three scenarios. First, the current scenario. We denote aj the numberCovid-19 deaths and µaj the I F R at age a in country j. The total number of Covid-19j d deaths in country is denoted j (data were taken from Our World in Data). Our esti-mate of the proportion of people infected in country j is given by ϕ = dj /99 a=0Najµaj.The number Covid-19 deaths at age a in country j is given by daj = ϕNaj µaj . The secondand third scenarios assume that nothing is done to stop the spread of the epidemic, whichinfects 80% of the population until reaching herd immunity (Banerjee et al., 2020). Inthis case, daj = 0.8Naj µaj . The two herd immunity scenarios dier in the IFR assumed(no saturation versus saturation of the health care systems).Covid-19 Deaths Covid-19 Deaths2000 1500 1000 5000 020406080100AgeONS data 5-year moving average150100500 020406080100AgeMinistry of Health data 9-year moving average(a) United Kingdom(b) PeruFigure A.5: Covid-19 deaths.Years of life lost due to Covid-19 The total number of years of life lost due toCovid-19 in country j is given by δj =99 a=0daj λaj .Results areshown ingureA.6forthe current scenario and in gure A.7 for the herd immunity scenarios.38% Years0.02 0.01PeruBelgium UnSitewdedKeinngdom0.001 0.0001PakistanPhilippines0.00001500 1000 20005000 10,000 20,000 50,000 100,000GDP per capita (PPP, constant 2011)0.01 0.001 0.0001 0.00001PeruBelgium UnSitewdedKeinngdomPakistanPhilippines500 1000 20005000 10,000 20,000 50,000 100,000GDP per capita (PPP, constant 2011)(a) Per years of life left (in %)(b) Per capitaFigure A.6: Years of life lost due to Covid-19 - current scenario.0.20.1Japan0.05Years per personZimbabwe0.020.01 500100020005000 10,000 20,000GDP per capita (PPP, constant 2011)50,000 100,000No saturationSaturation (ICU = dying)(a) Per years of life left (in %)(b) Per capitaFigure A.7: Years of life lost due to Covid-19 - herd immunity scenarios.39B Supplementary gures and tables for publication online0-5-10%-15-20-25 500100020005000 10,000 20,000GDP per capita (PPP, constant 2011)50,000 100,000GDP shock due to Covid-19 (in %, baseline estimates) GDP shock due to Covid-19 (in %, downside estimates)Figure A.8: Shock to GDP due to Covid-19 (baseline and downside estimates)Break-even ̂α100,000 10,0001000 100 1010.1 500ZimbabweSierra LeonePhilippines PakistanPeruUnited Kingdom100020005000 10,000 20,000GDP per capita (PPP, constant 2011)1.9$ poverty line50,000 100,000Figure A.9: αˆ Break-even for the 1.9 PPP-$ poverty line and the baseline growthscenario, Covid-19 mortality as of early June 202040Break-even ̂α11000,,000000 1000 100 10 10.1 500100020005000 10,000 20,000GDP per capita (PPP, constant 2011)1.9$ poverty line 3.2$ poverty line 5.5$ poverty line 21.7$ poverty line50,000 100,000(a) Baseline, distribution-neutralBreak-even ̂α11000,,000000 1000 100 10 10.1 500100020005000 10,000 20,000GDP per capita (PPP, constant 2011)1.9$ poverty line 3.2$ poverty line 5.5$ poverty line 21.7$ poverty line50,000 100,000(b) Downside, distribution-neutralBreak-even ̂α11000,,000000 1000 100 10 10.1 500100020005000 10,000 20,000GDP per capita (PPP, constant 2011)1.9$ poverty line 3.2$ poverty line 5.5$ poverty line 21.7$ poverty line50,000 100,000Break-even ̂α11000,,000000 1000 100 10 10.1 500100020005000 10,000 20,000GDP per capita (PPP, constant 2011)1.9$ poverty line 3.2$ poverty line 5.5$ poverty line 21.7$ poverty line50,000 100,000(c) Baseline, +3.6% in Gini(d) Downside, +3.6% in GiniFigure A.10: αˆ Break-even as of early June 2020 for the dierent scenarios of economicshock.41Break-even α̃10 1 0.1500 1000 20005000 10,000 20,00050,000 100,000GDP per capita (PPP, constant 2011)1.9$ poverty line (herd=80%) 3.2$ poverty line (herd=80%) 5.5$ poverty line (herd=80%) 21.7$ poverty line (herd=80%)1.9$ poverty line (herd=50%) 3.2$ poverty line (herd=50%) 5.5$ poverty line (herd=50%) 21.7$ poverty line (herd=50%)Figure A.11: α˜ Break-even and herd immunity thresholds of 50% vs. 80% in allcountries as of early June 2020 (baseline, distribution-neutral contraction, no-interventionscenario with saturation, group-specic poverty thresholds)Break-even α̃10Zimbabwe Sierra LeoneTimor-Leste1Philippines PakistanPeru0.1United Kingdom500 1000 20005000 10,000 20,00050,000 100,000GDP per capita (PPP, constant 2011)Herd immunity at 80% Herd immunity at 50%Figure A.12: α˜ Break-even and herd immunity thresholds of 50% vs. 80% in allcountries as of early June 2020 (baseline, distribution-neutral contraction, no-interventionscenario with saturation, extreme poverty threshold)4210 1 0.1Break-even α̃500 1000 20005000 10,000 20,00050,000 100,000GDP per capita (PPP, constant 2011)1.9$ poverty line 3.2$ poverty line 5.5$ poverty line 21.7$ poverty lineFigure A.13: α˜ Break-even for dierent poverty lines (baseline scenario, distribution-neutral), Covid-19 mortality estimated in a no-intervention scenario with saturation ofhealth care system43
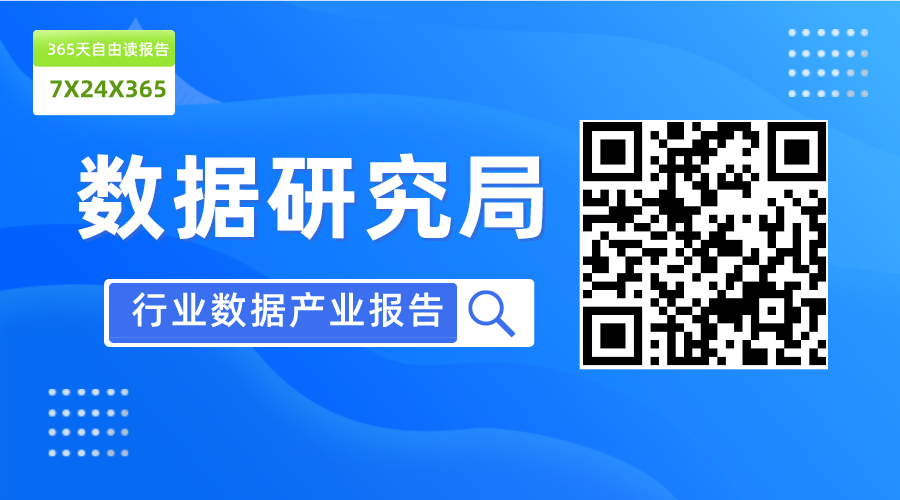
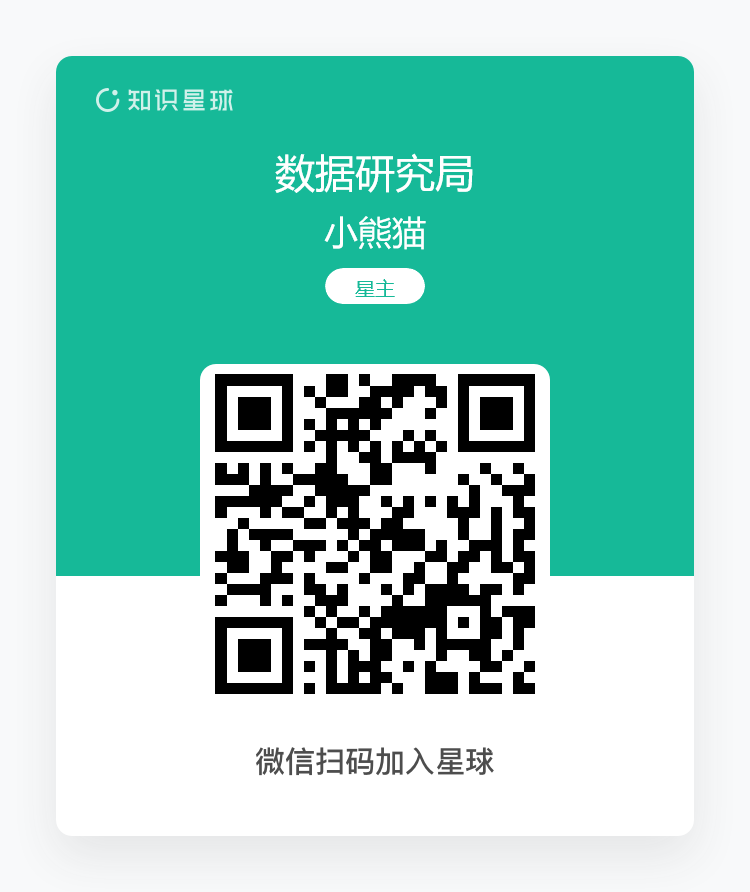