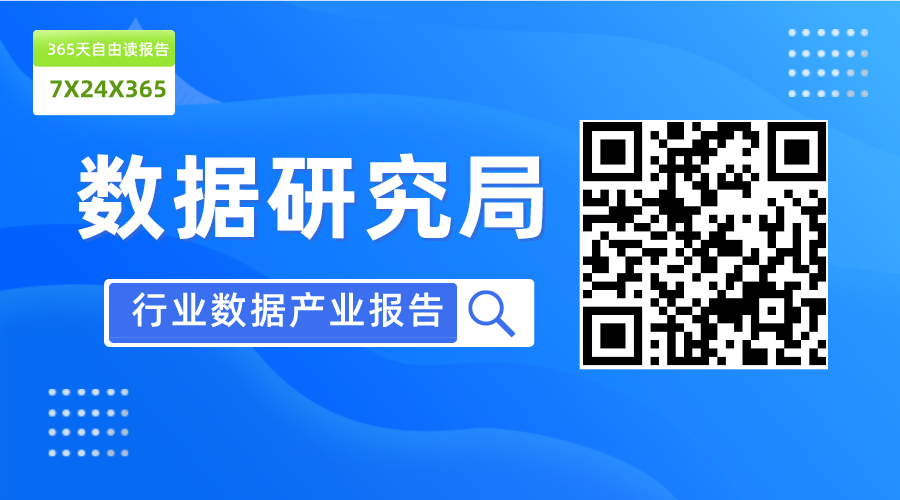
Public Disclosure Authorized e Economic Case for Nature Justin Andrew Johnson Giovanni Ruta Uris Baldos Raffaello Cervigni Shun Chonabayashi Erwin Corong Olga Gavryliuk James Gerber Thomas Hertel Christopher Nootenboom Stephen Polasky Public Disclosure Authorized Public Disclosure Authorized Public Disclosure Authorized A global Earth-economy model to assess development policy pathways e Economic Case for Nature A global Earth-economy model to assess development policy pathways © 2021 International Bank for Reconstruction and Development / The World Bank 1818 H Street NW Washington DC 20433 Telephone: 202-473-1000 Internet: www.worldbank.org This work is a product of the staff of The World Bank with external contributions. The findings, interpretations, and conclusions expressed in this work do not necessarily reflect the views of The World Bank, its Board of Executive Directors, or the governments they represent. The World Bank does not guarantee the accuracy, completeness, or currency of the data included in this work and does not assume responsibility for any errors, omissions, or discrepancies in the information, or liability with respect to the use of or failure to use the information, methods, processes, or conclusions set forth. The boundaries, colors, denominations, and other information shown on any map in this work do not imply any judgment on the part of The World Bank concerning the legal status of any territory or the endorsement or acceptance of such boundaries. Nothing herein shall constitute or be construed or considered to be a limitation upon or waiver of the privileges and immunities of The World Bank, all of which are specifically reserved. Rights and Permissions The material in this work is subject to copyright. Because The World Bank encourages dissemination of its knowledge, this work may be reproduced, in whole or in part, for noncommercial purposes as long as full attribution to this work is given. Any queries on rights and licenses, including subsidiary rights, should be addressed to World Bank Publications, The World Bank Group, 1818 H Street NW, Washington, DC 20433, USA; fax: 202-522-2625; e-mail: pubrights@worldbank.org. Cover photos: © Panos Tsolakis / Unsplash, © Nasa Earth Observatory / Flickr, © Egor Kamelev / Pexels, © Jeremy Zero / Unsplash Design by Voilà: chezVoila.com. v Acknowledgments “The Economic Case for Nature”, is part of a series of reports that lays out the economic rationale for investing in nature. Led by the Environment, Natural Resources and Blue Economy (ENB) Global Practice at the World Bank, the series aims to provide analytical insights to inform the process leading up to the 15th Conference of the Parties (COP-15) of the Convention on Biological Diversity, and assist countries implement the new post-2020 global biodiversity framework. The work has been undertaken in collaboration with the University of Minnesota and Purdue University and builds on previous analysis carried out with the World Wildlife Fund for Nature (WWF)–UK. The University of Minnesota team included Justin Johnson, James Gerber, Stephen Polasky, and Chris Nootenboom. The Purdue University team included Uris Baldos, Erwin Corong, Thomas Hertel, and Angel Aguiar. The World Bank team was led by Giovanni (Gianni) Ruta and Raffaello Cervigni and included Olga Gavryliuk, Shun Chonabayashi, and Fnu Hanny. The team worked under the guidance of Karin Kemper (Global Director for the ENB Global Practice), Christian Peter (Practice Manager for the ENB Global Practice, Global Engagement Unit), Iain Shuker (Practice Manager, ENB Global Practice, East Africa), and Benoit Blarel (former Practice Manager, ENB Global Practice). Sue Pleming and Sonu Jain from the World Bank’s External and Corporate Relations led the outreach and dissemination efforts. The authors are deeply grateful for the insightful comments and input received from the peer reviewers and colleagues. The peer reviewers for this report were Massimiliano Cali, Richard Damania, Madhur Gautam, and Svetlana Edmeades, from the World Bank, and Toby Roxbourg, Head of Sustainable Economic Policy, WWF-UK. Grzegorz Peszko, Garo Batmanian, Juliana Castaño, Fiona Stewart, Samantha Power, Marek Hanush, from the World Bank, Felix Nugee and Emily McKenzie, from the United Kingdom (UK) Her Majesty’s Treasury, and Alistair Rennie, from the UK Department for Environment, Food and Rural Affairs (DEFRA), also provided valuable feedback. The report received financial support from the Global Program on Sustainability and the Wealth Accounting and Valuation of Ecosystem Services (WAVES Plus) trust funds, generously supported by the UK DEFRA and the Department for International development (DfID); the Swiss State Secretariat for Economic Affairs; the European Commission; the Netherlands’ Ministry of Foreign Affairs; and the German Federal Ministry for Economic Cooperation and Development (BMZ). vi The Economic Case for Nature Execu ve summary Key messages The global decline of biodiversity and ecosystem services is a development issue: Economies, particularly in low-income countries, cannot afford the risk of collapse in the services provided by nature. The analysis in this report, the first-ofits-kind, shows that by a conservative estimate a collapse in select services such as wild pollination, provision of food from marine fisheries and timber from native forests, could result in a significant decline in global GDP: $2.7 trillion in 2030. Relative impacts are most pronounced in low-income and lower-middle-income countries, where drops in 2030 GDP may be more than 10 percent. — Nature-smart policies can reduce the risk of ecosystem collapse and are “win-win” policies in terms of biodiversity and economic outcomes. A combination of carefully crafted and coordinated policies, particularly those supporting innovation, can simultaneously benefit biodiversity and development. The policies considered in this report reduce Executive summary vii conversion of natural land and result in a general increase in global real GDP in 2030 that is estimated to be in the order of $50 billion to $150 billion. — The more countries cooperate, the better the outcomes are. The global community needs to put in place measures to incentivize such cooperation and to support an inclusive transition for those stakeholders who are affected by the economic reforms and face opportunity costs. — The nature and climate change agendas are complementary and there are synergies to be exploited to foster green, resilient, and inclusive development. The benefits of nature-smart policy increase substantially when the carbon sequestration services of nature are factored in. This analysis highlights the economic and environmental benefits to be gained by aligning global, regional, and national policies that address biodiversity loss as well as climate change mitigation and adaptation and improve local livelihoods. viii The Economic Case for Nature e global decline of biodiversi and ecosys m services is a development issue Economies are embedded in nature and depend profoundly on the flow of goods and services it generates, such as food and raw materials, pollination, water filtration, and climate regulation. Nature underpins all 17 Sustainable Development Goals and provides cost-effective mitigation options to the climate crisis. Yet, most indicators of the extent and health of natural ecosystems are sounding the alarm. Seventy-five percent of the Earth’s ice-free land surface has been significantly altered by human activity; the abundance of vertebrate species has declined by nearly 70 percent since the 1970s (WWF 2020); and 14 of the 18 assessed categories of ecosystem services have declined over the same period (IPBES 2019). These trends threaten the well-being and development prospects of entire communities and economies, including those that need this natural capital the most—whether to grow out of poverty or remain resilient to natural and economic shocks. Since economies are embedded in nature, policies to promote economic development should also be beneficial to nature. Our ability to produce valuable goods and services for a growing population is bounded by the fact that we cannot live and operate outside nature (Dasgupta 2021). At the heart of the challenge is the need to bring nature into decision making at all levels, to improve our collective ability to use the biosphere’s goods and services efficiently while allowing it to regenerate so that such goods and services may be sustained or enhanced over time. This report presents a first-of-its kind global integrated modeling exercise that demonstrates the economic importance of nature and helps the global community paint a landscape of possible scenarios of the interaction between nature’s services and the global economy to 2030. Recognizing that economies rely on ecosystem services and that loss of nature’s assets stems from economic decisions, this report presents a novel modeling framework that uses economic data to estimate how an economy might react to changes in selected ecosystem services. The model allows the study of the impact of changes in these ecosystem services—pollination, provision of timber and food from marine fisheries, and carbon sequestration by forests—on the global economy and vice versa between 2021 and 2030, to inform policy making. This work represents an important steppingstone toward “nature-smart” economic decision making. The primary audience is policymakers, notably ministries of finance, economic planning, environment, and agriculture, who face the complex trade-offs involved in management of natural capital at the country level and must weigh the costs and benefits of alternative policy responses to the global biodiversity crisis. As nations formulate a new set of global biodiversity targets at a landmark Conference of the Parties (COP-15) of the Convention on Biological Diversity (CBD), this report shows that nature-smart policies, particularly those supporting innovation, are a win for biodiversity and economic outcomes. The analysis also contributes to the analytical underpinnings of green, resilient, and inclusive development, including for the post-COVID-19 recovery. Executive summary ix Not ac ng is not an op on: ere are no winners under business-as-usual Conventional economic models do not account for the declining trends in nature’s services and thus provide an overly optimistic scenario of economic growth. When the loss of those services is included, growth in global GDP by 2030 slows considerably. The decline in the ecosystem services analyzed, caused by the conversion of natural land to cropland, pastureland and forest plantations, results in a loss of global real GDP in 2030 of $90-225 billion (depending on whether the associated value of nature-based carbon sequestration services lost are considered), when compared to a scenario with no change in nature’s services. Under the business-as-usual scenario, the world is projected to lose about 46 million hectares of natural land and face a continuous decline in fish stocks. This translates into a decline in the ecosystem services analyzed in this report—pollination, provision of timber and marine fish stocks—with implications for agricultural yields, the fisheries sector, and the output of industries dependent on timber, among other sectors. While GDP grows in all the scenarios analyzed, if incorporated into the economic model, the decline in ecosystem services results in slower growth and hence a loss of global real GDP in 2030 of $90 billion, compared to the baseline scenario where ecosystem services are not accounted for. If the impact on carbon sequestration services is also considered, the projected economic cost increases to $225 billion. The economic damages are greater if the global economy is unable to quickly adjust to the loss of ecosystem services. Following a shock, economies adjust to a new equilibrium through changes to market prices for goods and services and the quantities of such goods and services exchanged, re-orienting demand and supply towards inputs and outputs less affected by shocks, both within and across countries (through trade). However, the impact of shocks may be greater if markets are less flexible in adapting, and economic models might overestimate how adaptable markets are, especially with natural capital that has few substitutes. To see how the inability of markets to adjust could affect the outcomes analyzed in this report, more conservative assumptions are also tested. Under the business-asusual scenario, a less-flexible global economy loses $152 billion compared to the baseline without ecosystem services (not accounting for the carbon sequestration services of nature). This represents a loss that is 72 percent higher than in the case where economies more readily adjust to a new equilibrium. x The Economic Case for Nature e world cannot a ord the collapse of ecosys m services, as such a collapse would cost 2.3 percent of global GDP (-$2.7 illion) annually by 2030 and some of the poorer coun ies would be hit hardest Environmental degradation can push an ecosystem to a “tipping point” beyond which it will shift to a new state or collapse entirely. Such a collapse would lead to a large-scale, abrupt decline in ecosystem services. Even if the likelihood of global ecosystem collapse today is small, the catastrophic losses it would entail justify action to mitigate such risks. To assess the benefits of conserving natural capital, the integrated model analyzes the potential economic impact of the collapse of wild pollination, marine fisheries, and timber provision in native forests (the latter due to a widespread dieback of tropical forests and its conversion into savannah). The results show that in the scenario where tipping points are exceeded for these three services, Figure ES.1. Low-income and lower-middle-income countries stand to lose the most in relative terms if ecosystem services collapse Change in 2030 real GDP under the partial ecosystem collapse scenario compared with the no-tipping-point scenario A) By income group (the bars are proportional to the population in 2030) Executive summary xi global real GDP in 2030 contracts by $2.7 trillion (-2.3 percent annually by 2030, mostly in low-income countries), compared with the baseline scenario (Figure ES.1). Low and lower-middle income countries stand to lose the most in relative terms if ecosystem services collapse, putting at risk their prospects to grow out of poverty. Sub-Saharan Africa and South Asia would be hit particularly hard by a collapse in ecosystem services. The two regions would experience the greatest relative contraction of real GDP: 9.7 percent annually by 2030 (-$358 billion) for Sub-Saharan Africa and 6.5 percent (-$320 billion) for South Asia. This is due to reliance on pollinated crops and, in the case of Sub-Saharan Africa, reliance on forest products along with limited ability to switch to other production and consumption options that are less affected by the collapse of select ecosystem services. Impacts are also distributed unevenly across income groups: low- and lower-middle-income countries are the hardest hit, with a 10 percent (-$81 billion) and a 7.3 percent (-$734 billion) drop in real GDP in 2030, respectively. Low-income and lower-middle income countries also suffer from important setbacks in their 2021-2030 growth rates, seriously jeopardizing their prospects to grow out of poverty. The findings should be seen as a first step to a stress-test of the global economy against the risks of loss of biodiversity and ecosystem services. Change in 2030 real GDP under the partial ecosystem collapse scenario compared with the no-tipping-point scenario B) By geographic region (the bars are proportional to the population in 2030) xii The Economic Case for Nature A globally coordina d policy response enables development-environment win-wins Nature-smart policies make economic and environmental sense. This analysis identifies a set of policy pathways (Figure ES.2) that make economic and environmental sense, and the model demonstrates that the best outcomes are achieved if a combination of policies is implemented. The policies considered have already been implemented with some success and could have an important impact if they are more widely adopted. Figure ES.2. Schematic overview of the policy scenarios Note: P1: Decoupled Support to Farmers; P2: Domestic Forest Carbon (FC) payment; P3: Global FC payment; P4: Decoupled Support to Farmers + Domestic FC payment; P5: Decoupled Support to Farmers + Global FC payment; P6: Decoupled Support to Farmers + Agricultural R&D; P7: Decoupled Support to Farmers + Agricultural R&D + Global FC payment Executive summary xiii P1 The first policy type is to repurpose public sector support to economic activities such as agriculture, so that such support is not linked to current or future production volume or value, thus removing incentives to maintain marginal land in production. This is an immediate opportunity for countries looking to realign support to agriculture with sustainable management of biodiversity and ecosystem services. P2 & P3 The second policy type is to create incentives for conservation, for example by paying landowners in exchange for the protection of forest carbon sinks. This can be done through domestic or global forest carbon payment schemes. The report looks at each of these modalities in separate policy scenarios. P6 & P7 The third policy type, which in the analysis is used in combination with the other two, is to increase public investment in agricultural research and development (R&D) as an incentive to increase output on existing agricultural areas, rather than expanding cultivated areas. These three policy types are tested individually and in combination to assess their impacts on ecosystems and the economy. All seven policy scenarios analyzed reduce the risk of ecosystem services collapse, delivering economic gains (global GDP increases of up to $150 billion (Figure ES.3, vertical axes), with most countries poised to gain) and avoiding up to 50 percent of business-as-usual conversion of natural land (Figure ES.3, horizontal axes). Agricultural subsidies often encourage degradation because they are structured so that production increases as subsidies increase. A naturesmart approach “decouples” the subsidy so that farmers receive the income even when they conserve the forest rather than converting it to grow crops. Reforming farmer subsidies by providing support based on land holdings (thus decoupled from output produced or inputs used) decreases natural land loss by 8 percent between 2021 and 2030, preventing the conversion of nearly 4 million hectares. Other policies are substantially more impactful in terms of avoiding land conversion. Domestic and global forest carbon payments reduce natural land loss by 26 percent (12 million hectares) and 35 percent (16 million hectares), respectively. In the scenario that combines a global forest carbon payment scheme with domestic subsidy reform, 38 percent of natural land loss is avoided (18 million hectares). In addition to avoiding land conversion, decoupled support to farmers and payments for forest carbon services increase real GDP by $50 billion to $56 billion, with the former having the largest economic impact (+$56 billion). Results-based forest carbon payments are much more effective in protecting land but provide slightly lower real GDP benefits (+$50 billion to $53 billion). Combining decoupled support to farmers with carbon payment schemes enhances outcomes, in terms of conservation and real GDP growth (+$53 billion to $58 billion). Adding investment in R&D to the policy mix results in substantial economic benefits (+$142 billion to $148 billion) and conservation benefits, particularly in developing countries. Low-income, lower-middle-income, and upper-middle-income countries see net real GDP increases of $41 billion in the policy scenario without R&D investment and $119 billion in the scenario with R&D (Figure ES.4). The share of xiv The Economic Case for Nature Figure ES.3. Nature-smart policies offer win-wins, and they can be combined with one another for additional impact Change in Global GDP and avoided conversion of natural land compared with business-as-usual, by policy Note: P1: Decoupled Support to Farmers; P2: Domestic Forest Carbon (FC) payment; P3: Global FC payment; P4: Decoupled Support to Farmers + Domestic FC payment; P5: Decoupled Support to Farmers + Global FC payment; P6: Decoupled Support to Farmers + Agricultural R&D; P7: Decoupled Support to Farmers + Agricultural R&D + Global FC payment Executive summary xv the total benefits accruing to these countries increases to 80 percent with R&D. The gain from R&D investment is high especially in the poorer countries, which has important implications for reducing poverty as well as promoting food security. Moreover, investment in agricultural technology substantially improves environmental outcomes, particularly in developing countries. The most impactful policy among those analyzed is the combination of decoupled support to farmers, redirecting part of the resulting savings toward agricultural R&D, and a global forest carbon payment scheme. The combination policy approach halves the loss in natural land compared with businessas-usual, corresponding to sparing 23 million hectares from conversion (50 percent of business-as-usual levels). Indonesia, Brazil, Mexico, South Africa, Angola, and the Democratic Republic of Congo account for nearly one-third of this result. Figure ES.4. Investment in R&D results in substantial economic benefits in developing countries Economic effect of including R&D investments to policy scenarios, by country income group High income countries Low and middle income countries $90B could be added in real GDP change with R&D 16.5 41.3 No R&D +$12B 16.5 +$78B in real GDP change for low- and middle-income countries with R&D 41.3 With R&D Note: The scenario “No R&D” presented here corresponds to P5—combination of decoupled support to farmers with global forest carbon payments; the scenario “With R&D” corresponds to P7—decoupled support to farmers combined with global forest carbon payments and R&D investment. Middle-income countries include the lower-middle-income and upper-middle-income brackets. xvi The Economic Case for Nature The results point to the benefits of aligning policy responses to the biodiversity crisis and climate change. Well-designed policies that address biodiversity loss can also tackle climate change mitigation and adaptation. Investments in biodiversity must be made in a way that exploits synergies with climate change mitigation and adaptation and improves livelihoods at the local level. The model shows that when landowners are rewarded, at the domestic or global level, for maintaining the carbon sequestration potential of the forests on their lands, domestic policies such as the decoupling of agricultural subsidies and increased investments in R&D become more effective at protecting nature while minimizing economic loss. Adding a global forest carbon payment scheme to a national-level policy to decouple farmer support augments the natural land saving potential of the policy by more than 300 percent. Adding a global forest carbon payment scheme to domestic decoupling of farmer support with R&D investment increases the natural land saving potential by nearly 150 percent. The economic benefits also increase on the order of 4 percent. Nature and climate are two sides of the same coin; the two agendas are complementary and there are synergies to be exploited. Even ambitious targets, such as protecting 30 percent of the planet by 2030 (the “30x30” goal) are within reach. When combined with the most conservation-effective of the policy scenarios, achievement of the 30x30 goal results in a 0.1 percent decline of global GDP in 2030, compared with business-as-usual. The global loss is even smaller when GDP is adjusted for the climate change mitigation benefits of the lower carbon emissions made possible by the extra conservation of natural areas. From the global perspective, the economic loss caused by restrictions on land use (red arrows in figure ES.5) is almost entirely offset by the economic gains resulting from improved provisions of ecosystem services (green arrows in figure ES.5). The more cooperation, the better the outcomes. If all countries cooperate and simultaneously adopt nature-smart policies, they all gain, with appropriate compensatory payments. The global community needs to put in place measures to incentivize such cooperation. Accounting for climate change mitigation services considerably increases the number of countries benefiting under all the policy scenarios. Political economy, between and within countries, however, poses the biggest challenge to adopting nature-smart policies. Although at the global aggregate level the case for these policies is clear, and many countries appear to gain from all seven policies analyzed, a small number of countries may see a decline in real income, thus requiring compensation. If even a few countries lose out from the reforms, there could be important ramifications for implementing these policies. It is important to note that the climate change co-benefits of restoring and preventing the conversion of natural land substantially improve the chances of having a larger coalition of countries backing the reform. In addition, country-level adoption of nature-smart policies crucially depends on reconciling the incentives across social groups. In many countries where nature-smart policies deliver net economy-wide gains, some social groups stand to gain from their adoption, while others stand to lose. This highlights the key importance of the specific design of nature-smart policies, to ensure that the economy-wide gains they generate are distributed fairly across social groups, thereby avoiding political obstacles to their adoption. Executive summary xvii Figure ES.5. Globally, the costs of achieving the 30x30 target are largely offset by the benefits from ecosystem service gains, but there are important geographic differences Change in 2030 real GDP under the 30x30 scenario, by income group and driver of change (the bars are proportional to the population in 2030) xviii The Economic Case for Nature e opportuni ahead The coming decade provides an important window of opportunity to put planetary and human health on the same course. Parties to the CBD are preparing for COP-15 in Kunming, China, during which a new deal on nature is expected. The post-2020 global biodiversity framework will provide a unique opportunity to mobilize, over the coming decade, a diverse set of stakeholders—economic, financial, and private—and commit them to decisive action to reverse nature loss through conservation, sustainable use, and equitable sharing of the benefits of biodiversity. Moreover, COP-26 of the United Nations Framework Convention on Climate Change will provide further momentum to the nature agenda because healthy ecosystems support climate change mitigation and increase society’s resilience to climate change. To seize the opportunities offered by nature-smart policies, as part of the definition and implementation of the Kunming agreement, countries would benefit from: • Strengthening coordinated action at the global level, which will ensure that nature-smart policies deliver their full benefits and reduce the risks of some countries free-riding. • Enhancing capacity to design and implement policy reforms capable of producing economic and ecological impacts. It is particularly important to address domestic political economy challenges linked to some social groups losing from these policies. Since most countries are projected to have net GDP gains at the national level when adopting nature-smart policies, it should be possible to compensate losers while ensuring that a net benefit remains for the global economy. • Making nature-smart policies an integral part of the pursuit of global conservation goals, such as the ‘30x30’ goal, that are expected to be adopted at the CBD COP-15: while there will be a need to assist low-income countries mobilize the resources necessary to make up for the net economic loss they are projected to incur to achieve the 30x30 target, the cost of such compensation is likely to be lower if nature-smart policies were adopted at the same time, as they would reorient resources away from conversion of natural land, thereby reducing the opportunity cost of not converting natural land. Executive summary xix How the study was conducted This report presents a novel modeling framework that integrates select ecosystem services into a computable general equilibrium (CGE) model. This allows the study of the impact of changes in ecosystem services on the global economy and vice versa between 2021 and 2030, to inform policy making. The report assesses the link between the decline of the select ecosystem services—pollination of crops by wild pollinators, climate regulation from carbon storage and sequestration, provision of food from marine fisheries, and provision of timber—and the performance of key sectors that rely on these services, such as the agriculture, forestry, and fisheries sectors and related industries, as well as the effect this has on the broader economy through trade and changing demand for factors of production. The CGE model is linked to a suite of high-resolution, spatially explicit ecosystem services models. The model allows policymakers to analyze the global-to-local and local-to-global dynamics between nature’s services and the economy for the first time. The integrated model is used to compare the baseline (economy-only) scenario with a set of scenarios that simulate the interactions between ecosystems and the global economy to 2030—the “businessas-usual” scenario, where economic growth leads to a decline in the ecosystem services analyzed, and a “partial ecosystem collapse” scenario, where pressure on the selected ecosystems pushes them to tipping points, with dire economic consequences. A third set of scenarios assesses the effects of introducing various nature-smart policy reforms on environmental and economic outcomes in 2030. This type of model does not offer precise predictions about what the world will look like in the future. Rather, the scenarios described in this report illustrate the direction and range of possible outcomes of various policy approaches. Although they are the best available for assessing policy options related to biodiversity and ecosystem services, these tools are limited in the range of ecosystem services considered and analyze a relatively short time horizon. The results presented here, given their constrained application to a selected number of ecosystem services, point at possibly much larger impacts in the real world. In addition to considering more ecosystem services, a key opportunity for follow-up work would be to apply this analysis to country-specific contexts. xx The Economic Case for Nature Content Acknowledgments p.v Execu ve summary p.vi Acronyms p.xxii Glossary p.xxiii 1 In oduc on: Nature as a development asset p.1 2 Methods: Linking nature and the economy for improved policy making p.10 3 Se ng the scene: e business-as-usual scenario p.24 4 What happens when nature’s services collapse? p.38 5 Policy response and the poli cal economy p.58 6 Implica ons for the 30x30 global rget p.82 7 Conclusions and the way forward p.92 Content xxi Appendices p.97 A Coun y and region classi ca on p.98 B InVEST ecosys m services methods p.104 C De iled methodology p.106 D Measuring impacts on biodiversi in the in gra d ecosys m-economy model p.120 E Key produc on and ade parame rs modi ed to account for the impact of economic rigidi es in GTAP p.124 F Produc vi growth om agricultural R&D p.126 G Decoupling of agricultural subsidies p.130 H GTAP core model de ils p.132 I Es ma ng the opportuni cost of pro c ng 30 percent of rres ial land by 2030 p.136 References p.148 xxii The Economic Case for Nature Acronyms Note: All dollar amounts are U.S. dollars unless otherwise indicated. AEZ BAU BES CES CBD CGE COP EPPA ESA ESM FAO FCPF FISH-MIP GAMS GBO5 GDP GEMPACK GFP GTAP IAM IBRD IDA IEEM IIASA InVEST IPBES IPCC IUCN LUH2 LULC MAGNET OECD RCP REDD+ SEALS SEEA SSPs TFP UN UNFCCC UNEP WEF WHO WWF agro-ecological zone business-as-usual biodiversity and ecosystem services constant elasticity of substitution Convention on Biological Diversity Computable General Equilibrium Conference of Parties Economic Projection and Policy Analysis European Space Agency Earth System Model Food and Agriculture Organization Forest Carbon Partnership Facility Fisheries and Marine Ecosystem Model Intercomparison Project General Algebraic Modeling System Global Biodiversity Outlook, fifth edition Gross Domestic Product General Equilibrium Modelling PACKage Global Futures Project Global Trade Analysis Project Integrated Assessment Model International Bank for Reconstruction and Development International Development Association Integrated Economic-Environmental Modeling International Institute for Applied Systems Analysis Integrated Valuation of Ecosystem Services and Tradeoffs Intergovernmental Science-Policy Platform on Biodiversity and Ecosystem Services Intergovernmental Panel on Climate Change International Union for Conservation of Nature Land-Use Harmonization 2 land use/land cover Modular Applied GeNeral Equilibrium Tool Organization for Economic Cooperation and Development Representative Concentration Pathway Reducing Emissions from Deforestation and forest Degradation, plus sustainable management of forests, and conservation and enhancement of forest carbon stocks Spatial Economic Allocation Landscape Simulator System of Environmental and Economic Accounts Shared Socio-Economic Pathways total factor productivity United Nations United Nations Framework Convention on Climate Change United Nations Environment Programme World Economic Forum World Health Organization World Wildlife Fund xxiii Glossary 30x30 goal is a proposed target in the draft post-2020 global biodiversity framework, defined as follows: “By 2030, protect and conserve through well connected and effective system of protected areas and other effective area-based conservation measures at least 30 per cent of the planet with the focus on areas particularly important for biodiversity.” (CBD Secretariat) Aichi (Biodiversity) Targets are the 20 targets set by the Conference of the Parties to the Convention for Biological Diversity at its 10th meeting, under the Strategic Plan for Biodiversity 2011-2020. (Convention on Biological Diversity) Biodiversity is the variability among living organisms from all sources, including, inter alia, terrestrial, marine, and other aquatic ecosystems and the ecological complexes of which they are part; this includes diversity within species, between species, and of ecosystems. (Convention on Biological Diversity) Biodiversity loss is the reduction of any aspect of biological diversity (that is, diversity at the genetic, species, and ecosystem levels); it is lost in a particular area through death (including extinction), destruction, or manual removal; and it can refer to many scales, from global extinctions to population extinctions, resulting in decreased total diversity at the same scale. (IPBES) Biosphere is the sum of all the ecosystems of the world. It is both the collection of organisms living on the Earth and the space that they occupy on part of the Earth’s crust (the lithosphere), in the oceans (the hydrosphere), and in the atmosphere. The biosphere is all the planet’s ecosystems. (IPBES) Carbon sequestration is the process of storing carbon in a carbon pool. (IPCC) Climate change is change of climate which is attributed directly or indirectly to human activity that alters the composition of the global atmosphere and which is in addition to natural climate variability observed over comparable time periods. (UNFCCC) Decoupled is not linked to current or future production volume or value. For a policy measure to be agricultural support deemed decoupled, that production (or trade) should not differ from the level that would have occurred in the absence of the measure (OECD 2020a). Drivers of change, in the context of the Intergovernmental Science-Policy Platform on Biodiversity and Ecosystem Services and this report, are all the factors that, directly or indirectly, cause changes in nature, anthropogenic assets, and nature’s contributions to people and a good quality of life. Drivers have direct physical (mechanical, chemical, noise, light, and so forth) and behavior-affecting impacts on nature. They include, inter alia, climate change, pollution, different types of land or sea use change, invasive alien species and zoonoses, and exploitation. Indirect drivers are drivers that operate diffusely by altering and influencing direct drivers, as well as other indirect drivers. They do not impact nature directly. Rather, they do it by affecting the level, direction, or rate of direct drivers. Global indirect drivers include economic, demographic, governance, technological, and cultural ones. (adapted from IPBES) Ecological footprint is a measure of how much area of biologically productive land and water an individual, population, or activity requires to produce all the resources it consumes and to absorb the waste it generates, using prevailing technology and resource management practices. (The Global Footprint Network) The Dasgupta Review on the Economics of Nature defines the global ecological footprint as “humanity’s demands on the biosphere per unit of time […]. The ecological footprint is affected by the size and composition of our individual demands, the size of the human population, and the efficiency with which we both convert Nature’s services to meet our demands and return our waste back into Nature.” (Dasgupta 2021) Ecosystem is a dynamic complex of plant, animal, and microorganism communities and their nonliving environment interacting as a functional unit. (adapted from IPBES) xxiv The Economic Case for Nature Ecosystem services are the benefits people obtain from nature (Millennium Ecosystem Assessment). Ecosystem (also referred to as nature’s services are organized into four types: (i) provisioning services, which are the products people contributions to people) obtain from ecosystems and which may include food, freshwater, timbers, fibers, and medicinal plants; (ii) regulating services, which are the benefits people obtain from the regulation of ecosystem processes and which may include surface water purification, carbon storage and sequestration, climate regulation, and protection from natural hazards; (iii) cultural services, which are the nonmaterial benefits people obtain from ecosystems and which may include natural areas that are sacred sites and areas of importance for recreation and aesthetic enjoyment; and (iv) supporting services, which are the natural processes that maintain the other services and which may include soil formation, nutrient cycling, and primary production. (World Bank) Global Trade Analysis is a global network of researchers and policymakers conducting quantitative analyses of Project (GTAP) international policy issues. GTAP is coordinated by the Center for Global Trade Analysis in Purdue University’s Department of Agricultural Economics. In this report, the term GTAP is also used to identify a model that runs on the database developed by the network. GTAP-InVEST is the integrated model that uses the GTAP-based model complements it with the InVEST model. InVEST is able to calculate ecosystem services flows globally, at very high resolution (30m to 300m grid cells), under a variety of future scenarios. This ability is included to calculate global ecosystem services in GTAP-InVEST to analyze how changes in future scenarios would shock the global economy through a computable general equilibrium. This allows identifying the impact on indicators such as real gross domestic product, trade flows, employment, and commodity prices. Integrated assessment is a method of analysis that combines results and models from the physical, biological, model (IAM) economic, and social sciences, and the interactions among these components in a consistent framework to evaluate the status and consequences of environmental change and the policy responses to it. (adapted from IPCC) InVEST (Integrated is a suite of models used to map and value the goods and services from nature that sustain Valuation of Ecosystem and fulfill human life. It helps explore how changes in ecosystems can lead to changes in the Services and Tradeoffs) flows of many different benefits to people. Land use is the human use of a specific area for a certain purpose (such as residential, agriculture, recreation industrial, and so forth). Land use is influenced by, but not synonymous with, land cover. Land use change refers to a change in the use or management of land by humans, which may lead to a change in land cover. (IPBES) Materiality refers to the significance of a matter in relation to a set of financial or performance information. If a matter is material to the set of information, then it is likely to be of significance to a user of that information. (OECD) Materiality is rarely determinable by bare quantitative equation; rather, it requires an assessment of whether a reasonable investor would consider the information relevant to its decision whether or not to invest in a company. That assessment may require consideration of quantitative and qualitative factors. (Commonwealth Climate and Law Initiative) Nature, in the context of this report, refers to the natural world, with an emphasis on biodiversity. Within the context of science, it includes categories such as biodiversity, ecosystems, ecosystem functioning, evolution, the biosphere, humankind’s shared evolutionary heritage, and biocultural diversity. Within the context of other knowledge systems, it includes categories such as Mother Earth and systems of life. Other components of nature, such as deep aquifers, mineral and fossil reserves, and wind, solar, geothermal, and wave power, are not the focus of the report. Nature contributes to societies through the provision of contributions to people. (adapted from IPBES) Nature-based solutions are actions to protect, sustainably manage, and restore natural or modified ecosystems that address societal challenges effectively and adaptively, simultaneously providing human wellbeing and biodiversity benefits. (IUCN) Glossary xxv Nature-smart, in the context of this report, refers to approaches to policy, investments, and practices that include biodiversity and ecosystem services considerations from the perspectives of mitigating risks arising from the loss of nature and harnessing the economic and social benefits and opportunities that ecosystem services provide. Payment for ecosystem in this report, refers to mechanisms under which those who provide positive externalities services, are compensated for doing so, usually through payments from the beneficiaries. There is no settled definition of the term, however, and it can be used very broadly to include, for example, pollution charges. (World Bank) Precautionary principle pertains to risk management and states that if an action or policy has a suspected risk of causing harm to the public or the environment, in the absence of scientific consensus that the action or policy is not harmful, the burden of proof that it is not harmful falls on those taking the action. The principle is used to justify discretionary decisions when the possibility of harm from making a certain decision (for example, taking a particular course of action) is not, or has not been, established through extensive scientific knowledge. The principle implies that there is a social responsibility to protect the public from exposure to harm when scientific investigation has found a plausible risk or if a potential plausible risk has been identified. (IPBES; see also UNFCCC Article 3) Regime shift is a substantial reorganization in ecosystem structure, functions, and feedbacks that often occurs abruptly and persists over time. (Crépin et al. 2012) Shared Socioeconomic are part of a new framework that the climate change research community has adopted to Pathways (SSPs) facilitate the integrated analysis of future climate impacts, vulnerabilities, adaptation, and mitigation. (adapted from IIASA) Social cost of carbon is the net present value of aggregate climate damages (with overall harmful damages expressed as a number with a positive sign) from one more ton of carbon in the form of carbon dioxide (CO2), conditional on a global emissions trajectory over time. (IPCC) Spatial Econometric creates a replicable and empirically calibrated algorithm that allocates changes in land use Allocation Landscape and land cover (LULC) to resolutions applicable to ecosystem service models. Simulator (SEALS) Tail risks refer to events that have a small probability of occurring, namely those that fall outside three standard deviations above the mean under a normal distribution. Empirical studies in macroeconomics tend to approximate the deviations of aggregate economic variables from their trends with a normal distribution, which does not provide a good approximation of the distribution of aggregate variables at the tails and may significantly underestimate the frequency of large economic downturns (Acemoglu, Ozdaglar, and Tahbaz-Salehi 2017). In this report, the concept is applied in the context of nature loss, which is increasingly seen a source of “fat” tail risks, like those arising from climate change (Weitzman 2011). Tipping points refer to critical thresholds in an ecological system that, when exceeded, can lead to a significant change in the state of the system and prevent the system from returning to its former state. (adapted from Hoegh-Guldberg et al. 2019; IPBES). Zoonotic disease is an infectious disease that has jumped from a nonhuman animal to humans. (WHO) (or zoonosis) In oduc on: Nature as a development asset 1 2 The Economic Case for Nature In oduc on: Nature as a development asset The goal of this report is to provide evidence on the importance of nature to development and to identify win-win policy pathways that could deliver improved environmental and economic outcomes. There is growing evidence that, akin to climate change, the risks associated with biodiversity1 and ecosystem services2 loss are systemic. They threaten communities, value chains, and entire economies. Severe degradation of nature has the potential to undo development gains and strip some of the poorest economies of the foundations for future growth. At the same time, there are untapped development opportunities in conservation and sustainable use of nature. Yet, the unfolding global biodiversity crisis continues to be seen as a niche issue within the development agenda, in part due to data gaps and lack of understanding of the feedback effects between nature loss and the global economy. This report attempts to assess these interactions, highlighting the relevance of nature loss to economic outcomes and identifying potential win-win policy responses. 1. Biodiversity is the variability among living organisms from all sources, including, inter alia, terrestrial, marine, and other aquatic ecosystems and the ecological complexes of which they are part; this includes diversity within species, between species, and of ecosystems (Convention on Biological Diversity). Biodiversity is the characteristic of ecosystems that makes them resilient to shocks and change and allows them to thrive. 2. Ecosystem services (also referred to as nature’s contributions to people) are the benefits people obtain from nature (Millennium Ecosystem Assessment). Ecosystem services are organized into four types: provisioning, regulating, cultural, and supporting services (World Bank). 1 | Introduction: Nature as a development asset 3 1.1 Nature ma ers for development What do we mean by “nature”? This report uses the terms “nature” and “biodiversity and ecosystem services,” which are closely related. Nature refers to the ensemble of living organisms and the functions of the biosphere. The symbiosis between living organisms and the abiotic (nonliving physical and chemical) environment gives rise to ecosystems that control fluxes of water, carbon, energy, and nitrogen, among others. Biodiversity is the variability of genes, species, and ecosystems. The more diversity there is, the more ecosystems are resilient to shocks and hence able to sustain natural processes and provide valuable services to people. These natural processes provide ecosystem services that are in turn essential to our existence. In this report, the term “nature” is used to encompass “biodiversity” and “ecosystem services.” Humanity is embedded in nature, entirely dependent on it for survival, wellbeing, and economic prosperity (Dasgupta 2021). The benefits that humanity derives from nature are the flow of goods and services it generates, called ecosystem services. They include the provision of food, fresh water, timber, and fuelwood (provisioning services); regulation of climate and extreme weather; control of diseases and removal of toxic pollution (regulating services); and a basis for spirituality, personal enjoyment, and inspiration (cultural services). Underpinning these are the supporting services such as soil formation, the nutrient cycle, and primary production. One way to illustrate how ecosystem services contribute to economic activity is to look at sectors. It is estimated that $44 trillion of global value added—corresponding to more than half of the world’s gross domestic product (GDP)3—is generated in sectors such as construction and agriculture that highly depend ($13 trillion) or moderately depend ($31 trillion) on ecosystem services (WEF 2020).4 Other major sectors, such as travel and tourism, real estate, and retail, have hidden dependencies through their supply chains (WEF 2020). Sustainable development is a process of building and managing a portfolio of assets, including natural capital. A nation’s income is generated by its wealth, measured comprehensively to include all assets—produced, human, and natural capital (renewables and non-renewables) (World Bank, forthcoming). Building on the Brundtland Commission’s definition of sustainable development,5 this means that each generation should leave to its successor at least as large a productive base as it inherited from its predecessor, in which case the economic possibilities facing the successor would be no less than those the generation faced when inheriting the productive base from its predecessor (Dasgupta 2021). 3. Throughout the report, GDP is expressed in real terms. 4. The analysis of the World Economic Forum estimates the extent to which the global economy depends on nature, by assessing the reliance of 163 economic sectors on 21 ecosystem services (WEF 2020). This reliance is examined at the industry and regional levels, based on the economic value creation of each industry. 5. Brundtland Commission (1987) defined it as “... development that meets the needs of the present without compromising the ability of future generations to meet their own needs.” 4 The Economic Case for Nature Nature is a critical asset for low-income and lower-middle-income countries, where it represents a high share of the composition of national wealth. Renewable natural capital, such as forests, agricultural land, and fisheries, accounts for 23 percent of the wealth in low-income countries and 10 percent in lowermiddle-income countries (World Bank, forthcoming). Country-level analysis reveals the particularly high importance of renewable natural resources for development in low-income countries (dark blue bars in figure 1) and underscores the risks that the rapid biodiversity and ecosystem services loss represents to them (see sections 3 and 4). Nature is also an important source of livelihoods and a safety net for low-income households. Approximately 80 percent of the global population living below the poverty line resides in rural areas (World Bank 2018a), and they tend to depend greatly on biodiversity and ecosystem services for their livelihoods. Multiple examples of linkages between biodiversity, livelihoods, and jobs exist, particularly in the context of forest and coastal ecosystems. While one-third of humanity has a close dependence on forests and forest products, more than 90 percent of people living in extreme poverty depend on forests for at least part of their livelihoods (FAO and UNEP 2020). Research also shows that without income from natural resources, poverty among smallholders in Latin America, South Asia, East Asia, and Sub-Saharan Africa would be higher (Noack et al. 2015). There is also evidence that sustainable forest management can serve as a steppingstone out of poverty.6 For example, community-based forest management was shown to reduce overall and extreme poverty (without exacerbating inequality) across Nepal (Oldekop et al. 2019). Likewise, payment for ecosystem services helped conserve forests while achieving small poverty reduction gains in Mexico (Sims and Alix-Garcia 2017). Fishery-related livelihoods are equally important, particularly in rural and remote areas where alternative employment is limited. For example, inland fishing households in Cambodia get more than 50 percent of their income from fishing (FAO 2018). Fisheries also act as a safety net for vulnerable communities when economic or natural disaster strikes. 6. Causal links between good natural resource management and poverty reduction are hard to demonstrate. Miller et al. (2020) highlight how different social, economic, political, and environmental factors intersect to shape forest-poverty dynamics. More research, focused on spatially disaggregated poverty data, longitudinal approaches, causal chains, and comparative analyses, is needed for better understanding the role of socioeconomic, political, and biophysical factors in the forest-poverty dynamics. 1 | Introduction: Nature as a development asset 5 Figure 1. Renewable natural capital as a percentage of total wealth in select low-income and lower-middle-income countries, 2018 Renewable Natural Capital as a Percentage of Total Wealth 0% 25% 50% 75% Malawi Solomon Islands Guinea Mali Mozambique Guyana Liberia Sierra Leone Congo, Dem. Rep. Niger Suriname Belize Madagascar Chad Papua New Guinea Burkina Faso Kyrgyz Republic Lao PDR Cambodia Low income Lower middle Income 100% Source: Adapted from World Bank, forthcoming. Note: In this analysis, renewable natural capital includes land assets (agricultural land, protected forests and productive forests) and blue assets (fisheries and mangroves). This analysis looked at 146 countries, including 24 low-income and 36 lower-middle-income countries. Some countries are omitted; in 2018 the World Bank classified 31 countries as low-income and 47 countries as lower-middle-income (https://datahelpdesk.worldbank.org/ knowledgebase/articles/906519-world-bank-country-and-lending-groups). 6 The Economic Case for Nature 1.2 Nature is in rapid decline Accelerating changes in the socioeconomic sphere are dramatically decreasing the extent and condition of natural habitats and this is happening at a scale and rate that exceeds the ability of the biosphere to replenish, regenerate, and maintain balance. Since the 1970s, the human population has more than doubled, and global economic activity has increased sixfold.7 Incomes also increased, and the world has made remarkable and unprecedented progress in reducing poverty, which dropped from 60 percent in 1970 to less than 10 percent in 2018 (World Bank 2018b). Global demands and pressures on nature have drastically increased over the same period (box 1). The gap between humanity’s ecological footprint and the biosphere’s regenerative rate is widening, and this is inefficient from an economic standpoint and unsustainable (Dasgupta 2021). And it is taking its toll on biodiversity and ecosystems. Nearly one million animal and plant species (of an estimated eight million total) are now threatened with extinction and 14 of the 18 assessed categories of ecosystem services have declined since 1970 (IPBES 2019). Unabated nature loss and climate change reinforce each other and are capable of pushing the planet toward dangerous tipping points. Ecosystem dynamics are nonlinear and characterized by uncertain degradation thresholds and “tipping points” beyond which ecological regime shifts can occur, leading to drastic changes in an ecosystem’s capacity to provide services. Climate change is expected to exacerbate nature loss. It is a direct driver of biodiversity loss; and even under a 1.5°C to 2°C global warming scenario, the majority of terrestrial species ranges are projected to shrink dramatically (IPBES 2019). Species distribution, phenology, population dynamics, and, ultimately, ecosystem functioning are all likely to be adversely affected. Conversely, there is ample evidence that loss of nature contributes to climate change.8 The risks that nature loss represents are material9 and systemic. Building on the framework for climate risk classification developed by the Task Force on Climate-Related Financial Disclosure, biodiversity risks can be categorized as: (i) physical risks, related to the physical impacts of nature loss (these risks originate in the dependencies on ecosystem services, impacts of economic actors on them, and exposure to their loss); (ii) transition risks, related to the transition to the nature-smart economy (including potential effects of new regulation and 7. The population data were obtained from the World Bank Open Data (https://data. worldbank.org/); and data on global economic activity—output-side GDP at chained purchasing power parities (2017 US$)—were obtained from the Penn World Table, PWT 10.0 (https://www.rug.nl/ggdc/productivity/pwt/?lang=en). 8. For example, terrestrial and marine ecosystems sequester 60 percent of gross annual anthropogenic carbon emissions (IPBES 2019); their degradation results in the release of carbon and a reduction in their capacity to sequester carbon (IPCC 2019). 9. Materiality refers to the significance of a matter in relation to a set of financial or performance information. See the Glossary. 1 | Introduction: Nature as a development asset 7 public expectations); and (iii) systemic risks, related to impacts from extreme or compounding physical or transition risks that affect entire value chains or economies (World Bank Group 2020). The last category is described by the “Green Swan” report (Bolton et al. 2020) as “potentially extremely financially disruptive events that could be behind the next systemic financial crisis.” The COVID-19 pandemic, which may have its roots in environmental degradation,10 is an example of such “tail risks” playing out in the complex relationship between planetary and human health and the global economy. Market, policy, and institutional failures are facilitating the direct drivers of nature loss. A 2019 landmark report by the Intergovernmental SciencePolicy Platform on Biodiversity and Ecosystem Services (IPBES) identified five man-made direct drivers of biodiversity and ecosystem services loss: land and sea use change, direct exploitation, climate change, pollution, and invasive species. Facilitating these proximate drivers are market and policy failures that promote unsustainable production and consumption patterns. Public goods, positive and negative externalities, and information asymmetries are some of the market failures that misalign the private and social costs and benefits of the use of nature, encouraging loss and depletion beyond the level that is socially optimal. Policy intervention is essential, yet fiscal, economic, and trade policy have moved slowly to incorporate biodiversity values. Moreover, some policies, for example subsidies on fossil fuels and water, are to blame because they result in a negative price tag placed on nature’s goods and services (Dasgupta 2021). Markets for ecosystem services remain small and localized; adequate governance and institutional structures, such as property rights and enforcement of environmental laws, are lacking. Despite the broad recognition of the need to manage natural capital more sustainably, little progress has been made to date, suggesting that there may be “binding constraints” or factors that are preventing governments from taking action and thus keeping sectors and economies locked in unsustainable pathways.11 The opportunity ahead: the post-2020 global biodiversity framework. Present efforts to mitigate nature loss are insufficient, as evidenced by the indicators of ecosystem health globally, as well as lack of progress against the 2011–20 Aichi Biodiversity Targets. The international community fell short of meeting these targets, with none fully achieved and only six partially achieved, indicating insufficient progress in addressing the global crisis (CBD Secretariat 2020b). The new deal, dubbed the post-2020 global biodiversity framework, to be adopted at the 15th Conference of the Parties (COP-15) of the Convention on Biological Diversity (CBD) in Kunming, China, will provide a unique opportunity to mobilize a diverse set of stakeholders—economic, financial, and private. The new framework will commit the stakeholders to decisive action to reverse nature loss through conservation, sustainable use, and equitable sharing of the benefits of biodiversity. COP-26 of the United Nations Framework Convention on Climate Change (UNFCCC) is another opportunity to give further impetus to the nature agenda. 10. The origin of the COVID-19 outbreak and its transmission pathway are yet to be ascertained. However, multiple studies have shown a link between natural habitat destruction and greater risk of zoonoses (Olivero et al. 2017; Gibb et al. 2020). 11. They include short- and long-term trade-offs, lack of data and knowledge, capacity constraints, domestic political economy factors, and the global public good nature of many ecosystem services (World Bank Group, forthcoming). 8 The Economic Case for Nature This is because healthy ecosystems increase the resilience of society to climate change and are a powerful carbon sink, and addressing climate change is crucial to curbing nature’s loss. These milestones coincide with the COVID-19 recovery efforts, which could be effectively supported by investments in nature and should exploit opportunities to reorient development in a green, resilient, and inclusive direction. Box 1. Biodiversity and ecosystem services loss: trends and direct drivers Globally, biodiversity and ecosystem health are deteriorating at an unprecedented rate in human history. The past century has been dubbed “the age of the Anthropocene,”a denoting a geological era during which human activity has become the dominant influence on climate and the environment. The past 50 years, in particular, have witnessed a rapid decrease in the extent of natural habitats and the abundance of wildlife in them. One indicator of this is the average abundance of mammals, birds, fish, reptiles, and amphibians, which declined by 68 percent between 1970 and 2016; in South America—the worst affected region—it declined by 94 percent over the same period (WWF 2020). It is estimated that current extinction rates are 1,000 times as high as the background (pre-human) rate (Pimm et al. 2014), threatening to trigger a sixth mass extinction. Meanwhile, vital ecosystem services are starting to deteriorate worldwide, with 14 of the 18 assessed categories of nature’s services in decline since 1970 (IPBES 2019). The 2019 landmark report of the Intergovernmental Science-Policy Platform on Biodiversity and Ecosystem Services (IPBES 2019) attributes this to five direct “drivers of change” behind the unprecedented decline in biodiversity and ecosystem services: land use change, overexploitation,b pollution, climate change, and invasive species. The findings of the report include the following: • Approximately 75 percent of the Earth’s ice-free land surface and 66 percent of its marine environment have been significantly altered; more than 85 percent of the area of wetlands has been lost since 1700. • Between 1980 and 2000, 100 million hectares of tropical forests that are home to the highest levels of biodiversity were lost. Other sources point to a global loss of 178 million hectares of forests, an area the size of Libya, mainly to agricultural expansionc from 1990 to 2020 (FAO and UNEP 2020). • Live coral cover on reefs has nearly halved in the past 150 years, but the rate of decline has dramatically accelerated in recent decades, mostly because of climate change. • Humans are using the biosphere as a sink for unprecedented amounts of waste. • Cumulative records of alien species increased by 40 percent since 1980. a. Biologist Eugene Stormer and chemist Paul Crutzen coined the term and made it popular in the 2000s. b. Overexploitation means harvesting species from the wild at rates faster than natural populations can recover. It includes overfishing and overgrazing (IPBES 2019). c. Agricultural expansion continues to be the main driver of deforestation and forest fragmentation and the associated loss of biodiversity. Large-scale commercial agriculture (primarily cattle ranching and cultivation of soya bean and oil palm) accounted for 40 percent of tropical deforestation between 2000 and 2010, and local subsistence agriculture for another 33 percent (FAO and UNEP 2020). Source: Adapted from World Bank Group (forthcoming). 1 | Introduction: Nature as a development asset 9 1.3 Harnessing the economics toolbox Can economics help address the rapid decline in biodiversity and ecosystem services? At least since the end of World War II, economics has been mostly concerned with the evolution of GDP—a measure that provides a powerful indicator of an economy’s current level of production but that carries little meaning in terms of sustainability. Yet, economics is, according to the etymology of the term, the study of our home.12 Our home is, in some way, the environment that surrounds us. The classical economists held firmly that land, labor, and produced capital constituted the key assets that formed the basis for current and future consumption. Comprehensive wealth, including natural capital, is regularly measured by the World Bank as part of its Changing Wealth of Nations series (World Bank 2006, 2011, forthcoming; Lange, Wodon, and Carey 2018). The role of ecosystem services in economic accounts is increasingly being recognized through the work of United Nations (UN) Statistics Division. The UN System of Environmental Economic Accounting (SEEA) provides a framework that integrates economic and environmental data to provide a more comprehensive and multipurpose view of the interrelationships between the economy and the environment and the changes in the stock of environmental assets that generate benefits for humanity.13 A part of the SEEA, the SEEA Ecosystem Accounting, constitutes an integrated and comprehensive statistical framework for organizing data on habitats and landscapes, measuring ecosystem services, tracking changes in ecosystem assets, and linking this information to economic and other human activity.14 In March 2021, the UN Statistical Commission adopted the SEEA Ecosystem Accounting, turning the framework into a statistical standard. This report presents a first-of-its kind global integrated modeling exercise that integrates economic and ecosystem services data to demonstrate the importance of nature to economies and inform policy decisions. Recognizing that economic systems rely on nature’s services and that nature loss is driven in large part by economic decisions, the work developed here enhances an economy-wide, multi-region computable general equilibrium (CGE) model by incorporating a selected number of ecosystem services. The report is organized as follows. Section 2 provides an overview of the model, describing its key building blocks and mechanics. Sections 3, 4, and 5 analyze the model results. Section 3 compares a hypothetical “baseline” scenario, which does not consider ecosystem services, with one that considers trends in ecosystem services, termed the “business-as-usual” (BAU) scenario. Section 4 analyzes an extreme case of “BAU” as it explores the economic impacts of certain ecosystems reaching tipping points or regime shifts, causing a number of ecosystem services to collapse. Section 5 turns to policy analysis by modeling the impacts of implementing different policy scenarios on natural assets and economic variables. Section 6 exploits the model to analyze what the economic implications of protecting 30 percent of the terrestrial surface of the Earth by 2030 may be. Section 7 concludes with some recommendations on the way forward. 12. The word “economics” comes from two Greek words, “eco,” meaning home, and “nomos,” meaning management. The subject has developed from being about how to “manage the home” into a more wide-ranging subject. 13. UN SEEA website: https://seea.un.org/ (accessed March 16, 2021). 14. SEEA-EA website: https://seea.un.org/ecosystem-accounting (accessed March 16, 2021). Methods: Linking nature and the economy for improved policy making 2 12 The Economic Case for Nature Methods: Linking nature and the economy for improved policy making Synthesis This report analyzes two questions—how does a decline in ecosystem services affect economic indicators? And how can policy support both environmental and economic objectives? — These questions are answered with the help of a novel integrated modeling framework that combines a general equilibrium model with a set of ecosystem service models that cover pollination, timber provision, fisheries, and carbon sequestration. The framework paints a landscape of possible scenarios of the interaction between these ecosystem services and the economy to 2030. — The key lever in the model is land use change, which is an outcome of economic activity and a factor that determines the 2 | Methods: Linking nature and the economy for improved policy making 13 2 provision of ecosystem services that in turn affect the economy. A key novelty of this model is that land supply is not static—land use change is endogenously determined by changing conditions in the economy, reflecting more accurately the complexity of the dynamics analyzed. — The high spatial resolution of the model allows policymakers to analyze the global-to-local and local-to-global dynamics between nature’s services and the economy for the first time. 14 The Economic Case for Nature 2.1 Scope of the analysis This report considers two underlying questions of interest: • First, how does a change in ecosystem services impact economic variables, such as GDP, economic welfare, returns on factors of production, and output of sectors? • Second, how do changes in economic policy affect the achievement of environ- mental and economic objectives? To answer the first question, the report assesses the link between the decline of selected ecosystem services and the performance of key sectors that rely on these services, such as the agriculture, forestry, and fisheries sectors, and related industries, as well as the effect this has on the broader economy through trade and changing demand for factors of production. The analysis also considers the worst-case scenario where these selected ecosystem services collapse, sending ripple effects through the global economy. To answer the second question, the report compares the economic “BAU” scenario with one where a series of policy reforms are implemented to incentivize more sustainable practices. These include fiscal reforms, sectoral investments to increase productivity in agriculture, and results-based conservation schemes such as those made possible by forest carbon markets. The analysis focuses on four ecosystem services: pollination of crops by wild pollinators, climate regulation from carbon storage and sequestration, provision of food from marine fisheries, and provision of timber. It has been possible to run global ecosystem service models of the type presented in this report only since 2019, due to the (limited) availability of data at a high level of spatial resolution and computational challenges (Chaplin-Kramer et al. 2019). The model presented here overcomes these challenges, but only for a select number of ecosystem services that could be computed globally and linked to the economic model. Although the report only covers a limited range of ecosystem services, it still provides meaningful (albeit conservative) evidence of the importance of nature for economic development in the coming decades, demonstrating that the decline of even a limited range of nature’s services has implications for the global economy. As future research expands to cover more ecosystem services, estimates of these economic impacts are expected to be much larger. 2 | Methods: Linking nature and the economy for improved policy making 15 2.2 Overview of the methodology The integrated ecosystem-economy model presented in this report runs on three building blocks. These are a CGE economic model, a set of ecosystem service models, and a spatial simulator to improve the spatial granularity of the analysis. This section describes each of these building blocks, as well as the steps taken in the analysis. The core of the model is a CGE. CGE models are a class of economic models used to estimate how an economy may react to changes in policy, technology, or other external factors. They represent the economy through a series of equations that mimic the existence of multiple decision makers (for example, firms, households, and governments) that interact in multiple markets for intermediate and final goods and services. As demand and supply adapt in the different markets, so do the prices and quantities traded, resulting in an equilibrium level of global output, welfare, and use of resources. The CGE model used here is the Global Trade Analysis Project (GTAP) Data Base, which is the “common language” used by governments to conduct general equilibrium analyses on issues such as trade reform, climate policy, energy policy, and agricultural policy (Corong et al. 2017). This analysis uses a version of the model that has been expanded to agroecological zones, called the GTAP Agro-Ecological Zone (GTAP-AEZ) Data Base. The GTAP-AEZ adds elements to the original GTAP model that are useful for the analysis of competition for land and related issues, including (i) heterogeneous land endowment based on agro-ecological zones, (ii) land supply to activities that rely on land as a key factor of production (for example, forest, cropland, and pastureland), and (iii) changes in crop yields (that is, intensive and extensive farming). These additional elements allow for a more realistic modeling of land use change as sectors only compete for land that is suitable for their use. Box 2 discusses the capabilities and limits of the GTAP-AEZ. Appendices A and B provide a detailed description of the model. To reflect the fact that the economy is embedded in the biosphere, the general equilibrium model is linked to a suite of high-resolution, spatially explicit ecosystem services models that affect the economic parameters in the GTAP-AEZ model. The Integrated Valuation of Ecosystem Services and Tradeoffs (InVEST) tool is a suite of models used to map and value the goods and services from nature that sustain and fulfill human life. InVEST models are based on production functions that define how changes in an ecosystem’s structure and function are likely to affect the flows and values of ecosystem services across a landscape or seascape. The models account for service supply (for example, living habitats as buffers for storm waves) and the location and activities of people who benefit from services (for example, location of people and infrastructure potentially affected by coastal storms). InVEST models are spatially explicit, using maps as information sources and producing maps as outputs. InVEST returns results in biophysical terms (for example, tons of carbon sequestered) or economic terms (for example, the net present value of that sequestered carbon). Appendix B provides more details about InVEST. 16 The Economic Case for Nature Box 2. Overview of the GTAP-AEZ data base The economic model used in this report—the integrated ecosystem-economy model—is based on the Global Trade Analysis Project–Agro-Ecological Zone (GTAP-AEZ) Data Base (Hertel, Rose, and Tol 2009; Baldos and Corong 2020), which was updated extensively for this work. The version of GTAP-AEZ created for this report used 37 aggregated GTAP regions (referred to in this report as “GTAP country units”) and subdivided them further by the 18 agro-ecological zones as defined in Baldos and Corong (2020) (referred to in this report as “agro-ecological zones”), to create 337 regions (referred to in this report as “GTAP-AEZ regions”). Map B2.1 plots the 18 agro-ecological zones against the 37 GTAP country units. The list of countries and territories by GTAP country unit is included in appendix A. Other computable general equilibrium (CGE) models exist with capabilities similar to the GTAP-AEZ model, such as the ENVISAGE model (van der Mensbrugghe 2019), the Modular Applied GeNeral Equilibrium Tool (MAGNET) model (Woltjer and Kuiper 2014) at Wageningen University, and the Economic Projection and Policy Analysis (EPPA) model at the Massachusetts Institute of Technology (Chen et al. 2017). ENVISAGE is a CGE model designed to analyze trade issues and the economics of climate change. MAGNET is a modular extension of the standard GTAP model that can be tailor fitted to analyze various environmental and economic issues. EPPA is a CGE model that has a detailed representation of energy use in the economy, greenhouse gas emissions, and air pollutants. The GTAP-AEZ has been chosen for this analysis because it is tailored to answer environmental questions relevant to this work, in particular with detailed modeling of land as an input to production specific to the endowment within each AEZ. Additionally, the GTAP-AEZ model provides a sufficiently detailed starting point from which the competition for land across cropland, pasture, and managed forest is endogenized. It is also modified to simulate heterogeneous land supply that reflects the availability of land within each AEZ. Finally, the report uses a two-period comparative static model rather than a fully dynamic model, because this allows incorporating very detailed land use change dynamics that would be computationally challenging in a dynamic environment. The downside of this is that while the model can accurately describe the transition from 2021 to 2030 as a whole, it does not provide explicit outputs for the intermediate years. The third building block of the integrated ecosystem-economy model is a simulator that allows converting outputs from the economic model into spatially explicit variables that serve as inputs to the ecosystem models. There are two key spatial variables of interest in this report: the quantity of land that is allocated to different uses, and the locations in which different land uses are found. The first variable is an explicit output of the GTAP-AEZ model.15 A land supply curve for each agro-ecological zone and economic region is defined. Interaction with demand for land determines the amount of land that is put to productive use versus the land that is left in its natural state (Figure 2). The supply curve becomes infinitely inelastic as it approaches the maximum amount of economically exploitable land. 15. The original GTAP-AEZ model includes competition for land among sectors but does not allow land expansion to natural lands. Allowing natural land to be converted into cropland, pastureland, or managed forests is a refinement introduced by this specific application of the model. 2 | Methods: Linking nature and the economy for improved policy making 17 Map B2.1. Agro-ecological zones and GTAP country units Agro-ecological zones (AEZ) TROPICAL 1 2 3 4 5 6 TEMPERATE BOREAL 7 8 9 10 11 12 13 14 15 16 17 18 Arid Shorter Humid Longer growing period Note: The 18 agro-ecological zones are defined in Baldos and Corong (2020). The GTAP database covers 226 countries and territories. These are aggregated into 37 GTAP country units. When overlaid on the agro-ecological zones, this produced the 341 unique AEZ-Region zones shown above. In the figure, each agroecological zone is represented with a unique color. Beyond such limit, no expansion into natural land is economically feasible or possible (for example, because of slope conditions, climatic conditions, or land use restrictions).16 The asymptote is defined based on the MAGNET CGE model (Woltjer et al. 2014). Elasticities and land rental rates are unique for each agro-ecological zone and economic region. To determine the location of production, the Spatial Economic Allocation Landscape Simulator (SEALS) (described in Suh et al. 2020) is used, which allows for empirically-calibrated downscaling of land use and land use change predictions. This step is necessary because the models of biodiversity and ecosystem services, InVEST, require very high resolution to capture local dynamics. 16. This model feature enables a particularly interesting set of policy scenarios, including those that impose a restriction on land use, thus removing land from economic use (for example, through expansion of protected areas). Removing specific grid cells from economic use will shift the asymptote to the left (Figure 2) and affect the land rental rate and elasticity of land supply. Thus, protecting land is not “free” in this model. 18 The Economic Case for Nature Figure 2. Approach used to determine land use and land use change in the integrated ecosystem-economy model Note: (A) In the model, land area under productive use is positively related to the rental rate of land - the higher the rental rate (for example, because demand for crops is higher) the more land is converted. In the flat part of the supply curve, where land is widely available, increasing demand results in much land conversion and modest increases in the cost of land. In the steep part of the curve, as land becomes scarcer, increasing demand results in less land conversion and a higher cost of land. (B) When the amount of land that can be put to productive use is restricted (for example, because of an increase in the size of protected areas) the land supply curve shifts left and the cost of land increases faster as the land used reaches the limit of land available for productive use. The result is a decrease in the land area under productive use and an increase in the rental rate (or cost) of land. Source: This figure is adapted from Woltjer et al. 2014 2 | Methods: Linking nature and the economy for improved policy making 19 The model sequentially runs each of the building blocks. The process starts with projecting an economic BAU scenario to 2030 with detailed consideration of how changing market forces would endogenously drive land use change and conversion of natural land into economically utilized land (Figure 3). This first modeling step is referred to as GTAP-1, which provides inputs into the biophysical models. The results of the GTAP-1 project regional land use for cropland, pastureland, managed forests, and natural land. The next step downscales these results using the SEALS simulator from 37 economic regions to 8.4 billion grid cells (10 arc-second resolution, or roughly 300 meters at the equator). These high-resolution maps, which capture the local dynamic in ecosystems, such as physical proximity of wild pollinators within typical flight range of pollinator-dependent crops (see appendix C for more examples), are then used as inputs for the InVEST model. Finally, the outputs of InVEST are fed back into a second run of the economic model, referred to as the GTAP-2 run, to assess how changes in ecosystem services affect the economy between 2021 and 2030. The outputs of GTAP-2 provide detailed macroeconomic results that are relevant to decision makers. Figure 3. Depiction of the building blocks of the integrated ecosystem-economy model 20 The Economic Case for Nature The model builds on a prior version of the integration between GTAP-AEZ and InVEST used for the World Wildlife Fund’s Global Futures Project (GFP). The GFP assessed the impacts of changes in ecosystem services on the economy using the Shared Socio-Economic Pathways (SSPs) as inputs (see box 3). Analyzing scenarios using the SSPs has the advantage of ensuring consistency with the broad research community, which is centered on assessing the effects of different SSPs. However, the SSPs are “outcome” scenarios, meaning that they summarize the outcome of a combination of policies and other factors that will result in different stylized representations of how the future may unfold (for example, how much land use change may take place). The SSPs do not define explicitly what specific policies could be adopted to arrive at those outcomes (for example, which policies can modify trends in land use change). Additionally, it is not possible to consider how changes in ecosystem services would feed back and change the underlying assumptions on economic performance within the SSPs. The integrated ecosystem-economy model presented here helps address these gaps, and this is its key novelty. Box 3. The global futures project The Global Futures Project (GFP) was launched by the World Wildlife Fund in 2016 to assess how much the environment contributes to economic production and other macroeconomic performance indicators. The first phase of this report, published as Crossman et al. (2018), found that to answer this question accurately, it would be necessary to link a computable general equilibrium model with a high-resolution ecosystem services model. In particular, the authors recommended that the Global Trade Analysis Project (GTAP) model should be linked with the Integrated Valuation of Ecosystem Services and Tradeoffs (InVEST) model. This recommendation was implemented in the second phase of the GFP by the authors of this report (Johnson et al. 2020) and created the original version of GTAPInVEST, on which this report expands. The scenarios used in this report all represent what the world might look like in 2030 if specific policy actions are taken (or not) in 2021. Because the latest GTAP database reference year is 2014, the economy from 2014 to 2021 is projected using an approach set out by a large team of researchers to define baseline projections for economic indicators, as described in appendix C. From this 2021 baseline, a BAU scenario to 2030 is generated. This BAU scenario is then modified with seven different policy options, which are further detailed in section 5. Figure 4 provides a schematic overview of the input-output assumptions and baseline calculations. With the exception of the baseline (economy only) scenario, all the other scenarios incorporate the projected feedback between the economy and ecosystem services. 2 | Methods: Linking nature and the economy for improved policy making 21 Figure 4. Schematic overview of the scenarios analyzed Note: BAU = business-as-usual; BES = biodiversity and ecosystem services; GTAP-AEZ = Global Trade Analysis Project– Agro-Ecological Zone; LUC = land use change; R&D = research and development; RCP = Representative Concentration Pathway; SSP = Share Socioeconomic Pathway. 22 The Economic Case for Nature The accuracy of the integrated ecosystem-economy model is evaluated based on the integrated assessment model (IAM) projections. The projections from the model are compared with existing work to assess how well the baseline scenario captures the broad changes predicted by other models on future cropland and pastureland changes, as well as changes in crop and livestock production over 2014–30. As shown in figure 5, global land cover outcomes with the developed framework are close to the IAM projections. The GTAP-InVEST estimates are nearly within the range of values produced by the IAM projections. Figure 5. Global land cover change projections in this report versus integrated assessment models, 2014–30 Range of projections based on integrated assessment models -4% -2% 0% 2% % Land cover change Cropland Projections used in this report GTAP-INVEST 4% 6% 8% 10% Pasture Forestry Note: The points indicate GTAP-InVEST estimates. The bars indicate the minimum and maximum and estimates from the IAM projections (Popp et al. 2017). GTAP = Global Trade Analysis Project; IAM = Integrated Assessment Model; InVEST = Integrated Valuation of Ecosystem Services and Tradeoffs model. 2 | Methods: Linking nature and the economy for improved policy making 23 2.3 Novel and caveats There have been active efforts to model the interlinkages between the economy and the environment. The Integrated Economic-Environmental Modeling (IEEM) platform (Banerjee et al. 2016, 2019) is an example of a model that integrates the value of natural capital into the economic analysis of public policy and investment. Linking with ecosystem services modeling, the IEEM+ Ecosystem Services Modeling (ESM) platform (Banerjee et al. 2020) was developed to enhance economy-wide decision making by integrating rich information on natural capital stocks and ecosystem services as well as complex public policy goals at the national level. Waldron et al. (2020) provide global estimates of the costs and benefits of protecting 30 percent of the planet for nature based on a financial and economic analysis. However, there are to date no global approaches that combine an economic equilibrium model with ecosystem service models. There is a whole class of sustainability challenges that economics and environmental studies cannot analyze in isolation. Without an integrated model that combines both disciplines, our ability to assess policies aimed at addressing challenges such as ecosystem services loss is limited. Policy options and the global-to-local and local-to-global dynamics can be assessed using an integrated model. The novelty of the integrated ecosystem-economy model is that it simulates the interconnection between protecting land and economic activity at the global level. This is missing in other global models that analyze the costs of conservation (such as Waldron et al. 2020). The closest model to the one presented in this report in terms of land supply responses is the Modular Applied GeNeral Equilibrium Tool (MAGNET). However, MAGNET is not connected to a high-resolution land use change model, which is necessary to estimate ecosystem impacts. GTAP-InVEST includes full biosphere impacts, including the impact of changes in economic activity and policies on the environment as well as the effects of changes in the environment on the economy through ecosystem services and other linkages. Additionally, by connecting these models in a full cycle, it is possible to assess policies that affect different parts of the model in a comparable framework. The methodology used in this analysis has several caveats. The integrated ecosystem-economy model is not yet a comprehensive accounting of impacts of the ecosystem on economies, as it does not incorporate certain feedback loops. For instance, climate change impacts are held constant in the policy scenarios explored, while they are likely to affect and be affected by changes in ecosystem services. There are several ecosystem services with well-quantified economic impacts that could be incorporated to improve the model, including water quality benefits of intact ecosystems and sediment retention. Further ecosystem services also have significant impacts on economies but are not as easily modeled. Examples include perturbations to hydrological cycles and associated impacts on agricultural yields, loss of climate regulation services, and social movements in response to damaged ecosystems. Finally, the analysis of climate regulation from carbon storage and sequestration focuses on forests and does not include emissions from agriculture and livestock. Future work will need to include the full range of effects of policies on net carbon emissions, including those related to agriculture and livestock (as in Laborde et al. 2020) and those related to land use change (the focus of the present report). Se ng the scene: e business-asusual scenario 3 26 The Economic Case for Nature Se ng the scene: e business-as-usual scenario Synthesis The BAU scenario analyzed in this report projects the global economy in 2030 using a standard set of assumptions about economic and demographic growth, but also, crucially, it incorporates ecosystem services dynamics. — The key biophysical effect the integrated ecosystem-economy model projects is the conversion of 46 million hectares of natural land between 2021 and 2030, to managed forests (+17 million hectares), pastureland (+15 million hectares), and cropland (+13 million hectares). — The loss of natural land use in turn adversely impacts the availability of ecosystem services. The model projects a 0.3 percent reduction in global forestry production despite an 3 | Setting the scene: The business-as-usual scenario 27 3 expansion of managed forests, a 2.8 percent reduction in global marine fisheries production, and an additional 791 million metric tons of carbon dioxide emitted. In contrast, global pollinator-dependent agricultural productivity increases by 2.8 percent due to expansion of agricultural land. — The decline in the ecosystem services analyzed in this report results in a loss of global GDP in 2030 of $90 billion to $225 billion, depending on whether the associated costs of climate change are considered. 28 The Economic Case for Nature 3.1 Baseline scenario analyzed: e global economic outlook in 2030 This section describes the world’s economy in 2030 using the integrated ecosystem-economy modeling tool, setting the stage for the analysis of policy reforms. The analysis involves two steps: • The first step solves the GTAP-AEZ model in isolation to estimate what the world economy may look like in 2030 following changes in variables such as population growth and technological change. The major limitation of this scenario, which is referred to as the “baseline scenario,” is that, although changes in the economy increase the demand for natural capital, which in turn affects the provision of ecosystem services, this is not reflected in the economic projections. • The second step solves the GTAP-AEZ model while taking into account changes in ecosystem services to provide a more realistic representation of the economy in 2030. This “enhanced scenario,” referred to as the BAU scenario, then serves as the basis for policy analysis. This section describes the results under BAU, compared with the baseline. It also provides a sensitivity analysis by testing different assumptions about the ability of the economy to adjust to changes in natural capital. Under the baseline scenario, the world in 2030 is characterized by moderate economic and population growth, which is consistent with the middle-of-theroad SSP2 (O’Neill et al. 2014). Economic growth under SSP2 is moderate, with an average annual per capita global GDP growth rate of 2.8 percent, and rates of 1.6, 4.4, and 3.1 percent for high, middle, and low-income countries, respectively (Cuaresma 2017). These are similar to the annual GDP growth rates in the integrated ecosystem-economy model, which are 1.8, 4.2, 4.5, and 3.9 percent for high-, upper-middle-, lower-middle-, and low-income-countries, respectively. Population growth under the SSP2 scenario is also moderate. The global population in 2030 is predicted to be 8.3 billion (Samir and Lutz 2017). 3 | Setting the scene: The business-as-usual scenario 29 3.2 Results: feedback e ects between nature and the global economy under business-as-usual E ects on land use change and ecosys m services Under BAU, the world is projected to lose about 46 million hectares of natural land between 2021 and 2030. This is driven by conversion to land under managed forests (+17 million hectares), pastureland (+15 million hectares), and cropland (+13 million hectares) (Figure 6, panel A). The greatest loss of natural land is in Sub-Saharan Africa (-20 million hectares), followed by Latin America and the Caribbean (-12 million hectares) and East Asia and Pacific (-7 million hectares) (Figure 6, panel B). Furthermore, 26 GTAP country units account for 90 percent of the 2021–30 reduction in natural areas (Figure 6, panel C). Four countries account for 42 percent of this change: Angola and the Democratic Republic of Congo, treated as one region in the model, lose 6.6 million hectares of natural land, mostly to plantation forest. Brazil loses 6.5 million hectares, mostly to pastureland and cropland. Indonesia loses 3.2 million hectares, mostly to plantation forests, but also to cropland. Projections of change in land use and agricultural production in this model are consistent with the literature. Looking at agriculture, the global projection for growth in global crop production over 2021–30 in this report (37.5 percent) is within the range of 25-54 percent reported by Popp et al. (2017). The projected increase in global livestock production of 25 percent over the same period is within the range reported by the authors (19 to 39 percent). In terms of global cropland and pastureland, the GTAP-AEZ model component projects growth rates of 3.1 and 0.97 percent, respectively, which are well within the ranges of the global projections of the IAMs explored in Popp et al. (2017) (4.3 to 8.7 percent and -1.9 to 1.5 percent, respectively). The change in natural land reduces the availability of ecosystem services. Table 1 summarizes the effects of the reduction in natural land on pollination, timber provision, marine fisheries, and carbon sequestration and explains how they are estimated. These results should be interpreted not as simple biophysical outputs of InVEST, but rather the pre-trade shocks to the global economy that result from those biophysical outputs. Some results, such as the increase in pollination, highlight the multiple factors at play and the difficulties of presenting complex global biophysical results in terms of economic value. Overall, the model projects a decline in the ecosystem services analyzed in this report. 30 The Economic Case for Nature Figure 6. Change in managed land use and natural land under the business-as-usual scenario, 2021–30, by: A) Income group Negative change in land (million hectares) Positive change in land -30 -20 -10 0 10 20 Low Natural area Crop Pasture Managed forests Lower middle Upper middle High B) Geographic region -30 -20 -10 0 10 20 Sub-Saharan Africa Latin america & Caribbean East Asia & Pacific North America Europe & Central Asia South Asia Middle East & North Africa 3 | Setting the scene: The business-as-usual scenario 31 C) Global trade analysis project (GTAP) country unit Change in natural land (million hectares) -10 -5 0 5 Sub-Saharan Africa South Asia North America Middle East and North Africa Latin America and Caribbean Europe and Central Asia East Asia and Pacific Rest of SS Africa Angola+DRC South Africa Nigeria Ethiopia Madagascar India Pakistan Bangladesh Rest of S Asia Canada United States Rest of M East N Africa Morocco Egypt, Arab Rep. Brazil Argentina Rest of S America Central America Mexico Colombia European Union Russian Federation Poland Rest of C Asia Turkey Other Europe Indonesia Rest of SE Asia Malaysia China Vietnam Philippines Rest of E Asia Korea, Rep. Japan Oceania Note: The GTAP database covers 226 countries and territories. For analytical purposes and computational tractability, these are aggregated into 37 GTAP country units. For details on grouping and country classification, see appendix A. 32 The Economic Case for Nature Table 1. Summary of the biophysical changes under the business-as-usual scenario and the methods used to estimate them Ecosystem service Pollination Provision of timber Marine fisheries Changes observed under the business-as-usual scenario Methods • Global pollinator-dependent agricultural productivity increases by 2.8 percent. • Although agricultural expansion results in losses of natural land under BAU, triggering losses in pollination services, these effects are offset by total productivity increases from new agricultural land placed under production. • Country examples: In the Republic of Korea, agricultural yields of pollinatordependent crops drop by more than 52 percent; in the Arab Republic of Egypt, yields increase by nearly 40 percent. • Natural landscapes provide high-quality habitat for pollinators; if located close to pollinator-dependent cropsa— within the average flight range of wild pollinators of 1.3 kilometers (Sharp et al. 2018)—the habitat provides ecosystem services by bolstering crop yields. • A reduction in pollinator abundance due to land use change reduces agricultural productivity of nearby pollinator-dependent crops, as modeled using a modified version of the InVEST pollinator abundance model. • Reduced agricultural yields reduce exports; effects propagate through the GTAP model as a global impact of local agricultural productivity shocks (see Johnson et al. 2020). • Global forestry production declines by 0.3 percent despite an expansion of managed forests. • The extent of managed forests increases, yet the economic value of forestry decreases, indicating a reduction in the quality of managed forests (decreased biomass that hampers production). • Country example: Indonesia sees a 3.8 percent reduction in forest productivity, East Asia and Rest of South Asia in its see gains of more than 4.9 percent. • Impact on the forestry sector is estimated based on changes in the carbon stored in forests, modeled as a function of land use change (Johnson et al. 2020) using the InVEST carbon sequestration model. • As timber is extracted from forested areas, it is assumed that any change in the carbon stored constitutes a similar change in forestry productivity; more carbon indicates more timber yield per hectare; forest expansion adds to forest production. • Total change in forestry productivity in each country feeds into GTAP-AEZ that projects changes in supply, demand, and trade of timber goods and related economic indicators. • Global marine fisheries production falls by 2.8 percent. • The decline in fish stocks is driven by: (i) increased fishing activity (fishing continues unabated under BAU), and (ii) climatic shifts (rising sea temperatures shift the range of fish populations). • Country examples: Morocco and Egypt see an increase of 21 percent and a decrease of 17 percent in fisheries productivity, respectively. • Marine fisheries are modeled via an ensemble approach, including multiple fish growth models. • This ensemble model was developed by a project that compared how different fisheries models reported fish stocks, specifically total catch biomass, under different climate and fishing activity scenarios. • The gridded results were aggregated according to the marine economic exclusion zone of each GTAP-AEZ region. 3 | Setting the scene: The business-as-usual scenario 33 Ecosystem service Changes observed under the business-as-usual scenario Methods Carbon sequestration • The BAU scenario generates an additional 791 million metric tons of carbon dioxide emissions. • The climate change impacts of these emissions represent an economic impact of $135 billion. • Climate change impacts are modeled using the InVEST carbon sequestration model and the social cost of carbon (see Johnson et al. 2020). • Ecosystems mitigate climate change by sequestering and storing carbon in living biomass and soil; changes to the landscape can increase carbon pools by adding carbon-sequestering vegetation or release carbon from these pools into the atmosphere via land use change. • The social cost of carbon is set at $171 per metric ton of carbon dioxide (Johnson et al. 2020), which represents the marginal increase in damages to the global economy per ton of carbon emissions in a given year. • The economic damage is calculated post hoc as a direct impact on GDP and not explicitly included in the GTAP model, as the damages covered by the social cost of carbon are not exchangeable on the global market. • Effects on countries are proportional to their population, assuming a uniform distribution of the damages (a simplification of the reality). Biodiversity • Globally, the biodiversity indexb falls by 0.2 percent, reflecting loss in the overall quality of natural habitat supporting biodiversity. The biodiversity index was designed for use in land use protection decisions and the percentage change is hard to interpret, but it remains very useful for understanding the differences in harm to biodiversity across different scenarios. • A biodiversity “index” is used for geographic area; it is calculated by combining data on species richness, threatened and endangered species, rare ecoregions, and key biodiversity areas.b • This index is a holistic approximation of biodiversity, contrasting the number of species and rarity of the biome against the threats it faces. • Change in this biodiversity index is not uniform, falling 0.3 percent in Asian regions, and increasing by 0.22 percent in the Middle East and North Africa. Note: BAU = business-as-usual; GDP = gross domestic product; GTAP = Global Trade Analysis Project; GTAP-AEZ = GTAP–AgroEcological Zone; InVEST = Integrated Valuation of Ecosystem Services and Tradeoffs. a. A wide range of animals can be important pollinators (for example, birds, bats, moths, and flies), but bees are the most important group for most crops (Free 1993). As a result, the InVEST Pollination model used for this report focuses on the resource needs and flight behaviors of wild bees. b. See appendix D for details. 34 The Economic Case for Nature E ects on the economy, accoun ng for changes in ecosys m services The decline in the selected ecosystem services results in a loss of global GDP in 2030 of $90 billion to $225 billion. Incorporated into the CGE model, the decline of ecosystem services results in a loss of GDP in 2030 of $90 billion, compared with the baseline scenario, which does not account for ecosystem services. If climate change damages linked to the loss of ecosystems are also considered, the global cost in 2030 rises to $225 billion (Figure 7). The decline in ecosystem services affects countries to varying degrees, pointing to the added value of this analysis at the country level. For example, the change in 2030 GDP compared with the baseline ranges between -0.4 percent Figure 7. Correction in 2030 GDP after considering the loss of ecosystem services under the business-as-usual scenario, by income group Change in 2030 real GDP (US$, billions) -250 -200 -150 -100 Climate change damages -50 0 GDP loss Global Low income Lower middle income Upper middle income High income Note: The results presented compare the business-as-usual scenario (where changes in ecosystem services are accounted for) with the baseline scenario (which does not account for ecosystem services). GDP = gross domestic product. 3 | Setting the scene: The business-as-usual scenario 35 (10th percentile) and 0.2 percent (90th percentile). Similarly, the change in 2030 welfare compared with the baseline ranges between -5.2 percent (10th percentile) and 4.3 percent (90th percentile). It is also observed that countries experiencing economic losses account for the bulk of the global population. Figure 8 shows the reduction in 2030 GDP once the impact of ecosystem services change on the economy is considered. Nearly all the global population (98 percent) in 2030 will live in countries that lose in terms of GDP if the climate change damages are included (Figure 9). Figure 10 shows that the largest impacts on GDP per capita are found in poor countries. The magnitude of the economic loss hinges on the ability of the economy to adjust to shocks. CGE models often dampen shocks on the economy through price adjustments, substitution, trade, or other offsetting mechanisms. In sectors that are highly dependent on ecosystem services, however, markets may not be able to adjust as easily as would be expected, and there may be much less substitutability. For instance, environmental goods cannot always be replaced with man-made alternatives (Polasky et al. 2015). This could imply an overestimation of the ability of the economy to adapt to environmental shocks. This means that changes in local conditions, for example the increased scarcity of pollinator services, do not easily turn into substitution for pollinator-dependent crops, resulting in scarcity and higher costs for the economy. To reflect the impact of real-world economic rigidities on the economic projections, a sensitivity analysis is conducted that draws on the literature. To account for these rigidities, substitution elasticities that govern industry supply responses and consumer demand sensitivity to price changes are reduced from their initial Figure 8. Correction in 2030 GDP and economic welfare after considering the loss of ecosystem services under the business-as-usual scenario Real GDP variation (%) -2.5 -2 -1.5 -1 -0.5 0 0.5 1 1.5 Economic welfare variation (%) -10 -8 -6 -4 -2 0 2 4 6 36 The Economic Case for Nature Figure 9. Share of the global population gaining or losing GDP in 2030, under the business-as-usual scenario % of 2020 population 25 Gaining 0 Losing -25 -50 -75 -100 GDP 20% will fall to the losing side when climate change damages are considered GDP + Climate damages mean value estimates. To gauge the magnitude of the shock on the global economy better, the substitution elasticities that are part of the GTAP model are replaced; instead of the mean values (based on literature searches and validation studies), the values at the upper limits of the 95th percentile are used. Appendix E provides the details and the sources used for the sensitivity analysis. More conservative assumptions on the economy’s capacity to adapt to the decline in ecosystem services imply that there are greater economic impacts throughout the world. By lowering the range of the relevant elasticity values, the analysis finds that adding rigidities increases economic losses. The losses are calculated as the 2030 difference in GDP between the BAU scenario considering ecosystem services and the baseline scenario without ecosystem services, and they amount to $152 billion. In other words, in the BAU scenario, adding rigidities increases the negative impact of declining ecosystem services on GDP by 72 percent (excluding climate change damages). This is driven in large part by the impacts felt in agriculture, livestock, and forestry output: the 2030 output of these sectors drops by as much as $102 billion, which represents a drop that is 12 times higher compared with the scenario without rigidities. Upper-middle-income countries see the greatest reduction in GDP in absolute dollar terms under the rigid economy (-$110 billion), which is equivalent to a 60 percent increase in economic impacts compared with the scenario without rigidities. Similarly, the East Asia and Pacific region sees the greatest reduction in GDP (-$112 billion). At the country level, China stands out, as the impact of adding rigidities to the baseline without ecosystem services versus the baseline with ecosystem services results in a further loss of $38 billion. This sensitivity analysis with rigidities points at the fact that the economic results of general equilibrium models can be conservative. The effects of loss of ecosystem services could be severe if the economy fails to adapt. These effects are 3 | Setting the scene: The business-as-usual scenario 37 Figure 10. Correction in 2030 GDP per capita after considering the loss of ecosystem services under the business-as-usual scenario, by GTAP country unit Change in 2030 real GDP % per capita -2 -1 0 1 Angola+DRC Pakistan Philippines Morroco Oceania Colombia Rest of SS Africa Russian Federation Rest of M East N Africa Argentina European Union United States Mexico Canada Japan South Africa Rest of E Asia Turkey Brazil Korea Rep. Nigeria Rest of C Asia Other Europe Poland Rest of S America India Vietnam Central America Rest of SE Asia China Malaysia Ethiopia Indonesia Rest of S Asia Egypt, Arab Rep. Madagascar Bangladesh distributed unevenly across countries and regions, implying that appropriate policies need to be considered to help prevent the most vulnerable countries from having huge economic losses. Another reason why these results are conservative is that they do not take into account the risk of ecosystem services collapse, which would result in much greater economic losses (see the next section). Note: The results presented compare the businessas-usual scenario (which accounts for changes in ecosystem services) with the baseline scenario (which does not account for ecosystem services). What happens when nature’s services collapse? 4 40 The Economic Case for Nature What happens when nature’s services collapse? Synthesis Under the current trends in environmental degradation, the risk of ecosystem collapse is ever present and growing, representing systemic risks for economies. — This analysis demonstrates that the collapse of even a limited range of services–wild pollinators, marine fisheries, and timber provision from tropical forests–would have far-reaching effects on the global economy. — Global GDP in 2030 contracts by $2.7 trillion (-2.3 percent), compared with the baseline scenario where no ecological tipping points are reached. This is equivalent to a decline in 20212030 global GDP growth by 10 percent. — Non-extractive primary output contracts by 8 percent, affecting key exporters the most. 4 | What happens when nature’s services collapse? 41 4 — In 2030, 1.6 billion people may live in countries that are projected to experience declines in GDP of 10-20 percent, mostly low-income countries in Sub-Saharan Africa and South Asia. — Low-income and lower-middle-income countries stand to lose the most in relative terms: a greater contraction in GDP (-10 and -7 percent, respectively), compared with upper-middle- and high-income countries (-3.6 and -0.7 percent, respectively); and a relatively larger drop in GDP growth in 2030. — Regional analysis highlights the strong vulnerabilities of economies in Sub-Saharan Africa and South Asia to the abrupt loss of ecosystem services, which pairs with the high dependency of such regions on natural capital. 42 The Economic Case for Nature 4.1 Risk of the collapse of ecosystem services and why it is relevant for economic policymakers Passing ecological thresholds can trigger large, nonlinear, systemic change in the health of entire ecosystems. A growing body of literature suggests that vast part parts of the biosphere are overstretched17 (see also section 1). If goods and services are being drawn from an ecosystem in excess of its regenerative rate (which is what we are observing at present), the ecosystem will eventually tip over and collapse or undergo a “regime shift,” but the state of the ecosystem at which the collapse will occur is not known (Dasgupta 2021). Ecosystem dynamics are nonlinear. Ecosystem productivity declines at an accelerated rate as biodiversity loss (or other forms of environmental degradation) increases, and this can lead to abrupt, irreversible change in the ecosystem state. The degradation thresholds beyond which this change occurs are poorly understood, making these events inherently difficult to predict, especially at large spatial scales (Lenton 2013). Recent analyses of the impacts of continued deforestation in the Amazon suggest that loss of 20 to 25 percent of the remaining biome could trigger its dieback, illustrating the profound uncertainties and nonlinearities associated with nature loss (box 4). The understanding of the risks stemming from environmental degradation is more advanced in the context of climate change, and important parallels can be drawn from that body of work. The risks of abrupt, major shifts in the climate system as a result of the buildup of greenhouse gasses in the atmosphere are well documented (see IPCC 2018). Climate tipping points, ranging from loss of permafrost to the collapse of the Greenland and Antarctic ice sheets, can form a “cascade” and trigger self-amplifying global warming, bringing with it nonlinear, unpredictable, and potentially irreversible environmental, social, economic, and geopolitical dynamics. Based on current global warming trends, the prospects for avoiding any nearby climate tipping points are low (Lenton 2013; Kriegler et al. 2009). Of key importance for this report is also the fact that the interaction between climate change and nature loss amplifies the risk of reaching planetary tipping points. Notwithstanding the deep uncertainties surrounding these risks, their magnitude and complexity have provided the rationale for early global action to 17. The Stockholm Resilience Center research on “planetary boundaries” provides an approach that seeks to define a “safe operating space” in which human societies can develop and thrive (Rockström et al. 2009; Steffen et al. 2015). The boundaries are defined as a “safe” distance from a dangerous level (for processes without known thresholds at the continental to global scale) or a global threshold. Of the nine planetary boundaries analyzed, which include climate change, ocean acidification, biochemical flows (nitrogen and phosphorus), land system change, and biosphere integrity, among others, four have already been crossed. The Dasgupta Review on the Economics of Nature formally describes and analyzes the “Impact Inequality”—a framework that looks at the overshoot in humanity’s demands on the biosphere versus the supply of nature services—and suggests that this difference has been widening (Dasgupta 2021). 4 | What happens when nature’s services collapse? 43 mitigate them. The “precautionary principle”18 is widely accepted in international environmental treaties as a rationale for action to prevent events that can cause serious or irreversible damage, even in the absence of full scientific evidence on the probability of that event occurring. Literature on the economics of climate change also recognizes that the benefits of strong, early action considerably outweigh the costs (Stern et al. 2006; Nordhaus 2007). One recent study shows that the social cost of carbon in the default Dynamic Integrated Climate-Economy model increases eightfold when large-scale singularities or “tipping elements” are incorporated (Cai, Lenton, and Lontzek 2016). The growing risk of ecosystem services collapse underscores the high cost of inaction when it comes to nature loss. Like climate change, ecological regime shifts can result in catastrophic disruption of the provision of the ecosystem services on which economies and communities rely. In the financial sector, for example, there is growing awareness of the risks of “green swans”19—low-probability but large and potentially highly disruptive events that can permeate the economy. Risks associated with nature loss are financially systemic because of the complexity, interdependence, and interconnectedness within the financial system (Bolton et al. 2020; Dasgupta 2021). There is also a growing recognition of the need to better account for such risks. The chances of their occurrence are not reflected in past data and therefore traditional approaches to risk management consisting of extrapolating historical data and assumptions of normal distributions are largely irrelevant for assessing future risks (Bolton et al. 2020). COVID-19 serves as an example, demonstrating the effects of systemic risks associated with degradation of nature for the entire global economy. According to new analysis (Dobson et al. 2020), the financial damage from the economic and health crisis linked to the COVID-19 pandemic dwarfs, by several orders of magnitude, the cost to mitigate and prevent the risks of the pandemic emerging in the first place.20 Macroeconomic models typically do not account for the systemic risks associated with ecosystem services collapse. Macroeconomic models mostly represent the global economy as external to—rather than embedded in—the biosphere (see section 2). The nonlinear dynamics of ecosystems and uncertain degradation thresholds beyond which regime shifts occur also make modeling the impact of nature loss on economies challenging. As a result, macroeconomic models and, more broadly, deterministic projections of the effects of continued degradation 18. The 1992 UNFCCC Article 3 states that “The Parties should take precautionary measures to anticipate, prevent or minimize the causes of climate change and mitigate its adverse effects. Where there are threats of serious or irreversible damage, lack of full scientific certainty shall not be used as a reason for postponing cost-effective measures to prevent environmental degradation.” Source: UNFCCC, May 9, 1992. https://unfccc.int/resource/docs/convkp/conveng.pdf. 19. The “green swan” concept used in this report finds its inspiration in the now famous concept of the “black swan” developed by Nassim Nicholas Taleb in 2007. Black swan events have three characteristics: (i) they are unexpected and rare, thereby lying outside the realm of regular expectations; (ii) their impacts are wide ranging or extreme; and (iii) they can only be explained after the fact. These events typically fit fat-tailed probability distributions (they exhibit a large skewness relative to that of the normal distribution, but also relative to the exponential distribution). Source: Bolton et al. (2020). 20. Net costs of actions to prevent future zoonotic pandemics have been estimated at $18 billion to $27 billion per year (Dobson et al. 2020). In comparison, COVID-19 may reduce GDP by at least $5 trillion in 2020, and the willingness to pay for avoided loss of lives constitutes many additional trillions. These costs exclude the rising tally of deaths, deaths from other causes due to disrupted medical systems, and loss of forgone activities due to social distancing. 44 The Economic Case for Nature of nature on the global economy often fail to reflect systemic risks—the chance of catastrophic impacts of nature loss and the true cost of inaction. Several local analyses of ecosystem collapse exist, such as the assessment of the socioeconomic damage of a potential Amazon forest dieback (Lapola et al. 2018), but global analyses are lacking. Moreover, in traditional CGE models, many negative impacts are ameliorated to some degree by a built-in assumption that economies are able to substitute away from affected sectors, although, in reality, substitution may be limited in the case of environmental goods (Polasky et al. 2015). Box 4. Risk of ecosystem collapse: The example of the Amazon The Amazon biome is an example of the symbiosis of the biotic (living) organisms and abiotic (nonliving) environment that supports Earth systems and gives rise to vital ecosystem services. One of them is carbon sequestration—the Amazon contains an amount of carbon equivalent to a decade of global human emissions (Lovejoy and Hannah 2019). Another is evapotranspiration, which controls atmospheric moisture and weather patterns in the basin and far beyond. The Amazon generates half of its own rainfall by recycling moisture five to six times as airmasses move from the Atlantic across the basin (Salati et al. 1979). The vapor generated by the Amazon also gets transported to different regions. For example, it contributes to winter rainfall in southern Paraguay, southern Brazil, Uruguay, and central-eastern Argentina (Lovejoy and Nobre 2018). Observational studies carried out in the Amazon over the past 30 years show evidence that the forest and regional climate are changing (Lapola et al. 2018). Forest loss reduces rainfall and results in warmer, drier weather, threatening to disrupt the positive feedback loop that keeps the tropical forest alive. Nearly 17 percent of the Amazon basin and 20 percent of the Brazilian Amazon have already been deforested (Lovejoy and Nobre 2019). Some estimates suggest that loss of 20-25 percent of the remaining biome could trigger an ecosystem regime shift; widespread deforestation in the southern Amazon has already reduced rainfall significantly (Lovejoy and Nobre 2018). Regional climate scenario projections also envisage severe alterations of forest structure, biodiversity, and function (Lapola et al. 2018), adding to these pressures. The Amazon forest dieback hypothesis projects an abrupt, basin-wide, climate-driven shift of the region’s rainforest to a drought-prone vegetation with lower biomass, savannah, or even degraded vegetation (Lapola et al. 2018). Uncertainties remain not only around the biophysical processes in these scenarios, but also the socioeconomic impact. Some 30 million people depend directly or indirectly on the forest for their well-being. Some studies show that extreme deforestation in the Amazon may reduce rainfall in key growing seasons as far as in the Midwest, Northeast, and South in the United States (Dasgupta 2021). Estimates also suggest that the costs of acting now to curtail the impacts or mitigate the risk of this dieback are lower than the socioeconomic losses involved in a “no action or action later” scenario (Lapola et al. 2018). 4 | What happens when nature’s services collapse? 45 4.2 Par al ecosystem collapse scenarios analyzed To help address this gap, the integrated ecosystem-economy model analyzes the potential impacts of three major ecological regime shifts on the global economy to 2030. Drawing on the work of the Stockholm Resilience Centre,21 the model assesses the impact of the collapse—a 90 percent reduction in the flow of ecosystem services value—of wild pollination, marine fisheries, and timber provision (as a result of widespread conversion of tropical forests to savannah) on real economic activity between 2021 and 2030 (see appendix C). The model estimates the magnitudes of ecosystem services feedbacks on global economic projections and should be seen as the equivalent of a stress test of the global economy. These selected ecosystem services collapse scenarios are used as inputs into the integrated ecosystem-economy model and shock different components of the economy (Table 2). For instance, the collapse of wild pollinators reduces the productivity of agriculture; the severity of this effect at the country level depends on the extent to which crop production is reliant on wild pollination. The fisheries collapse impacts the model by lowering the total catch biomass, which translates into a technology-neutral productivity change in the fisheries sector. The collapse of native forests reduces the availability of timber by 90 percent in agro-ecological zones 5 and 6 (where the evidence suggests there is potential for a regime shift to savannah/shrubland), which in turn affects many productive sectors, such as manufacturing, construction, pulp and paper, energy, and others. The modeled effect signifies a permanent reduction of the productive potential of the economy as a result of the substantial loss of global natural capital, which is difficult or impossible to substitute with human and produced capital. The results are considered conservative for several reasons. First, the analysis only considers selected ecosystem services. Second, the model does not include the direct negative effects of climate change on ecosystem services. Third, the CGE model likely overestimates the ability of economies to adjust to shocks resulting from the partial collapse of ecosystem services. Fourth, some of the losses in GDP growth—for example, 12 percent of the global population experiencing a drop in GDP growth of 45 percent—would likely be associated with societal conflict (see the next section). Finally, there are significant nonquantifiable socioeconomic impacts associated with losing vital ecosystem services at the global scale, such as loss of food security and cultural heritage. 21. This analysis used the Regime Changes database of the Stockholm Resilience Centre to survey potential tipping points and selected three that have connections to the ecosystem services modeled in this report. 46 The Economic Case for Nature Table 2. Overview of the three ecosystem services collapse scenarios Scenario Wild pollination collapse Marine fisheries collapse Widespread conversion of tropical forests to savannah Overview of the methods used The model looks at the effect of a 90 percent reduction in wild pollination sufficiency on agricultural yields, focusing on crops that are dependent on wild pollination. Crops that are only partially dependent on these services will not see yield reductions as large as the pollinator collapse. The model builds on Bauer and Wing (2016), making the scenarios spatially explicit through the Integrated Valuation of Ecosystem Services and Tradeoffs tool. The model relies on the Fisheries and Marine Ecosystem Model Intercomparison Project data (Lotze et al. 2019). To simulate the regime shift, the model assumes a severe climate change scenario (Representative Concentration Pathway (RCP) 8.5 instead of RCP4.5) and further takes the worst-case outcome in terms of climate change impact reported in the uncertainty bounds and sensitivity analysis. The model simulates severe disruptions of fish migration that lead to a reduced total catch biomass, which in turn impacts the economic model. The model modifies two elements of the Spatial Economic Allocation Landscape Simulator model to simulate widespread collapse of tropical forests that results in forests converting into grasslands and shrubs: (i) assuming 88 percent less forest cover for all tropical regions; and (ii) lowering expansion suitability for forestry in the Amazon basin. This scenario impacts the economy through reduced provision of timber from native forests in agro-ecological zones 5 and 6 by 90 percent. 4 | What happens when nature’s services collapse? 47 4.3 Results: Es mated e ects of par al ecosystem collapse on the economy in 2030 E ect on GDP As a result of the collapse of wild pollinators, marine fisheries, and timber provision from tropical forests, global GDP in 2030 declines by 2.3 percent (-$2.7 trillion), affecting lower-income countries disproportionately (Figure 11). The global aggregate conceals a relatively larger contraction of GDP in lower-income countries, which account for nearly half of the world’s population. Figure 11. Change in 2030 real GDP under the partial ecosystem collapse scenario compared with the no-tipping-point scenario A) By income group (the bars are proportional to the population in 2030) 48 The Economic Case for Nature Figure 11. (con nued) B) By geographic region (the bars are proportional to the population in 2030) Note: The population data are based on the United Nations’ 2019 World Population Prospects for 2030. The analysis considers 226 countries and territories (see appendix A). “Other drivers” accounts for general economic growth, population size, and sectoral efficiencies. B = billions; BES = biodiversity and ecosystem services; GDP = gross domestic product. Low-income countries see a 10 percent drop in GDP in 2030 (equivalent to $81 billion), whereas lower-middle-income countries see a 7.3 percent drop (equivalent to $734 billion). In contrast, GDP contracts by 3.6 percent (-$1.5 trillion) in upper-middle-income countries and by 0.7 percent (-$471 billion) in high-income countries. The heterogeneity of the effects is due, in part, to the high dependency of the output of low-income and lower-middle-income countries on forestry production and pollinated crops, along with their limited ability to switch to other production and consumption options (Box 5 provides an illustration of how the tipping points generate changes in the economy). At the regional level, Sub-Saharan Africa and South Asia are hit particularly hard. The two regions experience the greatest contraction of GDP in percentage terms—losses of 9.7 percent (-$358 billion) and 6.5 percent (-$320 billion), respectively. East Asia and Pacific and Latin America and the Caribbean see losses on the order of 3.4 percent (-$1.3 trillion) and 3.3 percent (-$305 billion), respectively. Fifty-one countries, with a population of 1.6 billion people in 2030, are projected to experience a 10 to 20 percent decline in GDP (Figure 12). These include Bangladesh (-20.4 percent), Madagascar (-19.9 percent), Angola and the Democratic Republic of Congo (-19.3 percent), Pakistan (-15 percent), Ethiopia (-14.7 percent), Indonesia (-11 percent), and 44 countries in the Rest of Sub-Saharan Africa (-10.4 percent). 4 | What happens when nature’s services collapse? 49 Box 5. Tipping points and the resulting general equilibrium effects Given its ability to track adjustments through the fabric of the economic system, the integrated ecosystem-economy model can provide insights on the pathways of impact of any given exogenous shock. In general, a shock such as a collapse of ecosystem services will result in productivity losses in the crops, forestry, and fisheries sectors. Lower productivity, in turn, will result in less output and higher output prices. Next, higher output prices will lead to greater demand for inputs by the affected sectors, and this will drive up input costs for the whole economy, with eventual effects on export and import flows. Following this causation chain can help in understanding the way in which partial ecosystem collapse produces effects in different economies, as exemplified below. Bangladesh. Partial ecosystem collapse in Bangladesh results in substantial decline in fishery sector productivity—falling by around 8 percent compared with the baseline scenario where no ecological tipping points are reached. Fisheries gross output declines by $5.4 billion relative to the baseline. Labor and capital demands by fisheries grow roughly by 1.7 and 1.5 times compared with the baseline, respectively. Total gross output for tradable commodities and GDP decrease by around $20.5 billion and $58.9 billion, respectively. Ethiopia. The forestry sector in Ethiopia faces significant losses under the partial ecosystem collapse scenario. Forestry sector productivity decreases by 16 percent relative to the baseline, while gross output shrinks to $4.7 billion in 2030 (compared with $5.9 billion in 2030 under the baseline scenario). Labor and capital demand in this sector grow by roughly 2.5 times compared with the baseline. Total gross output for tradable commodities and GDP shrink by around $34.0 billion and $13.7 billion, respectively. Indonesia. Compared with the baseline without ecosystem collapse, forest sector productivity in Indonesia decreases significantly by 33 percent, while gross output shrinks by $6.3 billion under the partial collapse scenario. Rising output prices result in greater demand for labor and capital by the forestry sector—growing by roughly 5.6 and 5.5 times, respectively, compared with the baseline. Total gross output for tradable commodities and GDP shrink by around $303.3 billion and $146.9 billion, respectively. Brazil. Brazil’s oilseeds sector is adversely affected by the pollination loss from partial ecosystem collapse. Oilseed sector productivity falls by 6 percent relative to the baseline, resulting in gross output loss of around $11.3 billion. Demand for labor and capital in this sector grows by roughly 9.7 and 8.4 times, respectively, compared with the baseline. Total gross output for tradable commodities and GDP shrink by around $220.5 billion and $149.5 billion, respectively. In absolute terms, the greatest losses of GDP are expected in middle-income countries. The worst affected country is China, which sees its GDP drop by $943 billion in 2030, followed by India (-$193 billion), Brazil (-$150 billion), Indonesia (-$144 billion), and Nigeria (-$139 billion) (Figure 12). The projected loss in GDP signifies a permanent reduction of the productive potential of the economy, with potentially long-lasting effects on incomes and employment. 50 The Economic Case for Nature Figure 12. Change in 2030 GDP under the partial ecosystem collapse scenario compared with the no–tipping point scenario, by GTAP country unit, in (A) monetary terms and (B) percentage terms A) Change in monetary terms (US$ billions) -1000 -500 0 Sub-Saharan Africa B) Change in percentage terms -25 -20 -15 -10 -5 South Asia North America Middle East and North Africa Latin America and Caribbean Europe and Central Asia East Asia and Pacific 0 Madagascar Angola+DRC Ethiopia Rest of SS Africa Nigeria South Africa Bangladesh Pakistan Rest of S Asia India Canada United States Egypt, Arab Rep. Morocco Rest of M East N Africa Rest of S America Brazil Central America Argentina Colombia Mexico Rest of C Asia Turkey Other Europe Poland Russian Federation European Union Indonesia Vietnam Philippines Malaysia Rest of SE Asia China Korea, Rep. Rest of E Asia Oceania Japan Note: The GTAP database covers 226 countries and territories. For analytical purposes and computational tractability, these are aggregated into 37 GTAP country units. For details on grouping and country classification, see appendix A. 4 | What happens when nature’s services collapse? 51 E ect on economic growth Presented in terms of the implications for economic growth, the analysis reveals that reaching ecological tipping points could jeopardize some of the poorest countries’ prospects for growing out of poverty. Figure 13 illustrates these impacts. A 90 percent reduction in wild pollinators, timber provision from tropical forests, and marine fisheries could result in nearly 10 percent lower global GDP growth from 2021 to 2030 (9.5 percent) than under a no–tipping point scenario. The distributional effects across country income groups are again regressive. Low-income countries may experience one-third lower GDP growth (31 percent), whereas lower-middle-income countries may forgo 20 percent of growth over the next decade. Impacts at the regional level would be most severe in SubSaharan Africa, which could see a 26 percent drop in GDP growth, South Asia may see a 18 percent drop, and Latin America and the Caribbean a 16 percent drop. Seven countries—Madagascar, Bangladesh, Pakistan, Ethiopia, Indonesia, Angola and the Democratic Republic of Congo—could see their GDP growth drop by more than 45 percent. Approximately 12 percent of the population could experience a drop in GDP growth by more than 45 percent. The greatest losses in real GDP growth are observed in Madagascar (-103 percent), Bangladesh (-73 percent), Pakistan (-55 percent), Ethiopia (-53 percent), Indonesia (-47 percent), Angola and the Democratic Republic of Congo (-47 percent), South Africa (-30 percent), and Vietnam (-29 percent). These results highlight the relevance of the risks associated with nature loss for development efforts. Sustained economic growth is recognized as the most powerful tool for reducing poverty and increasing shared prosperity. The literature finds that the pace of growth is the main determinant of poverty reduction (Nallari and Griffith 2011). Although poverty responds to growth differently across countries due to factors such as productivity gains, the extent of inequality, as well as policies and institutions, it is generally accepted that growth tends to raise incomes for all members of society, including the poor, and, if sustained, it can generate virtuous cycles of prosperity and opportunity and support progress against multiple dimensions of poverty. The opposite is also true: low or declining economic growth—such as that projected under the partial ecosystem collapse scenarios—leads to increases in the incidence of poverty (examples from the literature include Chen and Ravallion (2000)). 52 The Economic Case for Nature Figure 13. Change in GDP growth over 2021–30 under the partial ecosystem collapse scenario compared with the no–tipping point scenario, by (A) income group, (B) geographic region, and (C) GTAP country unit A) Income group Change in real GDP growth (%) -40 -30 -20 Total change Forestry -10 Pollination Fisheries Others 0 Low income Lower middle income Upper middle income B) Geographic region -40 -30 Total change High income -20 -10 0 Forestry Pollination Fisheries Others Sub-Saharan Africa South Asia Latin America and Caribbean Middle East and North Africa East Asia and Pacific Europe and Central Asia North America 4 | What happens when nature’s services collapse? 53 C) Global trade analysis project (GTAP) country unit Change in real GDP growth (%) -100 -75 -50 -25 Sub-Saharan Africa South Asia North America Middle East and North Africa Latin America and Caribbean Europe and Central Asia East Asia and Pacific 0 Madagascar Ethiopia Angola+DRC Rest of SS Africa Nigeria South Africa Bangladesh Pakistan Rest of S Asia India Canada United States Egypt, Arab Rep. Morocco Rest of M East N Africa Rest of S America Brazil Colombia Argentina Central America Mexico Turkey Rest of C Asia Other Europe Poland European Union Russian Federation Indonesia Vietnam Philippines Malaysia Rest of SE Asia China Japan Korea, Rep. Oceania Rest of E Asia Note: The GTAP database covers 226 countries and territories. For analytical purposes and computational tractability, these are aggregated into 37 GTAP country units. For details on grouping and country classification, see appendix A. BES = biodiversity and ecosystem services; GDP = gross domestic product; GTAP = Global Trade Analysis Project. 54 The Economic Case for Nature E ects on sector output The output of sectors that rely directly on ecosystem services could drop by 8 percent (-$602 billion) by 2030. The model analyzed potential disruptions in the output of the agriculture, livestock, forestry production, and fisheries sectors. Under the partial ecosystem collapse scenario, the world’s non-extractive primary output—across this selected range of sectors—contracts by 8 percent or $602 billion (Figure 14). Some of the greatest losses in relative terms occur in Angola and the Democratic Republic of Congo (-26 percent or $17.3 billion), Madagascar (-23 percent or $1.6 billion), Philippines (-18 percent or $12.2 billion), Bangladesh (-17 percent or $14.0 billion) and Vietnam (-16 percent or $10.9 billion). Some of the key exporters of wood and wood products,22 as well as major exporters of beef23 and crops24 are also affected. In absolute terms, the greatest losses are in China (-9 percent or $194 billion), India (- 6 percent or $46 billion), the United States (-8 percent or $41 billion), Brazil (-15 percent or $36 billion), and the European Union (-5 percent or $28 billion). In terms of distributional effects across country income groups, low-income countries are hit the most (-11 percent or $18 billion), followed by lower-middle income countries (-10 percent or $207 billion) and uppermiddle-income (-9 percent or $301 billion). In particular, the world’s crop output contracts by 9 percent (-$400 billion) by 2030. The geographic distribution of the decline in crop output in absolute terms follows a pattern that is similar to that of the agriculture, livestock, forestry and fishery output. In percentage terms, the greatest losses of crop output occur in Madagascar (-33 percent or $800 million), followed by Brazil (-21 percent or $31 billion), the Philippines (-17 percent or $5 billion), Argentina (-16 percent or $7 billion), Turkey (-14 percent or $7 billion), Angola and the Democratic Republic of Congo (-14 percent or $3 billion), and China (-12 percent or $142 billion). Uppermiddle-income countries see the greatest losses (-11 percent or $203 billion), followed by lower-middle-income and low -income countries (-8.4 percent each or $118 and $9 billion, respectively) and the high-income group (-7 percent or $70 billion). Global fisheries output in 2030 contracts by 15.4 percent (-$94 billion) under the partial ecosystem collapse scenario. In relative terms, the fisheries output declines the most in Bangladesh (-29 percent or $5.4 billion), Angola and the Democratic Republic of Congo (-27 percent or $4.4 billion), Ethiopia (-26 percent or $0.6 billion), Philippines (-22 percent or $3.5 billion) and Nigeria (-21 percent or $4.6 billion). These are among the countries with greatest decline in fisheries output in absolute terms, along with China (-16 percent or $42 billion), India (-16 percent or $4.9 billion) and Indonesia (-17 percent or $3.8 billion). 22. FAOSTAT Forestry Production and Trade data, http://www.fao.org/faostat/en/#data/FO. 23. U.S. Department of Agriculture, Foreign Agricultural Service, Global Market Analysis, https://apps.fas.usda.gov/psdonline/circulars/livestock_poultry.pdf. 24. FAOSTAT Countries by Commodity, http://www.fao.org/faostat/en/#rankings/countries_ by_commodity. 4 | What happens when nature’s services collapse? 55 Figure 14. Change in 2030 output for agriculture, livestock, forestry, and fishery under the partial ecosystem collapse scenario compared with the no–tipping point scenario, by GTAP country unit Total change ($ USD billions) -200 -100 0 Sub-Saharan Africa South Asia North America Middle East and North Africa Latin America and Caribbean Europe and Central Asia East Asia and Pacific Total change in percentage terms -30 -20 -10 0 10 Angola+DRC Madagascar Rest of SS Africa Nigeria South Africa Ethiopia Bangladesh Pakistan Rest of S Asia India United States Canada Rest of M East N Africa Egypt, Arab Rep. Morocco Brazil Argentina Rest of S America Central America Mexico Colombia Turkey Rest of C Asia Poland Other Europe Russian Federation European Union Philippines Vietnam Malaysia China Indonesia Korea, Rep. Rest of SE Asia Japan Oceania Rest of E Asia Note: The GTAP database covers 226 countries and territories. For analytical purposes and computational tractability, these are aggregated into 37 GTAP country units. For details on grouping and country classification, see appendix A. 56 The Economic Case for Nature The impacts of ecosystem regime shifts go beyond the primary sector, with negative impacts on manufacturing, particularly in low-income and lower-middle-income countries. As shown above, when tipping points cause ecosystem regime shifts, the model shows a generalized reduction in primary (excluding minerals) output measured at market prices. Table 3 summarizes the results by income group and geographic region. The reduction of output in agriculture, livestock, forestry, and fisheries at the global level is around 8 percent, compared with the BAU scenario with no tipping points. Importantly, the impacts are also felt in manufacturing and to a much lesser extent in the service sector. Manufacturing is hit particularly hard in low-income countries, especially in SubSaharan Africa and South Asia, with industries highly dependent on the transformation of agricultural products. Latin American and the Caribbean also faces a substantial blow. Table 3. Change in 2030 output at market prices under the partial ecosystem collapse scenario, by region and income group (%) Low-income Lower-middle income Upper-middle income High-income East Asia & Pacific Europe & Central Asia Latin America & Caribbean Middle East & North Africa North America South Asia Sub-Saharan Africa World Agriculture, livestock, forestry, and fisheries -11 -10 -9 -4 -9 0 -12 -12 -8 -8 -11 -8 Manufacturing -19 -10 -3 0 -1 1 -10 -3 -2 -11 -15 -2 Services -2 0 -1 0 -1 0 1 0 0 0 1 0 4 | What happens when nature’s services collapse? 57 Economy-wide impacts are high in resource-dependent developing countries. As shown in table 3, the cross-sectoral impacts are high in Sub-Saharan Africa (driven mainly by the drop in the forestry and fisheries sectors), South Asia (fisheries), and Latin America and the Caribbean (forestry and crop sectors). Madagascar’s manufacturing sector output in 2030 is projected to decline as much as 55 percent in the tipping point scenario, and the country’s service sector output declines by 19 percent. This is linked to the drop in crop, forestry, and livestock outputs. Manufacturing and services outputs are also severely affected in Ethiopia (-42 and -26 percent, respectively). Manufacturing also suffers in Pakistan (-32 percent) and Indonesia (-23 percent). In Bangladesh, the fisheries sector is hit particularly hard (-29 percent of output). Policy response and the poli cal economy 5 60 The Economic Case for Nature Policy response and the poli cal economy Synthesis This analysis demonstrates that nature-smart policies can be win-win—good for ecosystems and good for economies—with the best outcomes achieved if a combination of policies is implemented. — All the policy scenarios analyzed avoid conversion of natural land: decoupled agricultural support to farmers avoids 8 percent of BAU conversion; domestic and global forest carbon payment systems avoid 26 and 35 percent, respectively; and a comprehensive policy package where decoupled support to farmers is combined with global forest carbon payments and investment in agricultural research and development (R&D) avoids 50 percent. — All the scenarios analyzed are win-win policies that generate economic gains alongside conserving natural ecosystems: basic policies—decoupled agricultural support and local and global forest carbon mechanisms increase global GDP by $50 billion to $56 billion in 2030; a more comprehensive policy approach that includes investment in R&D results in an increase in output of all economic sectors, including manufacturing and services. The results stress that no single policy response can deliver all the desired outcomes, and a combination of policies goes much further in delivering both environmental and economic benefits. — R&D investments benefit developing countries disproportionately. Fifty-five percent of the economic benefits from comprehensive policy action combining decoupled support to farmers, global 5 | Policy response and the political economy 61 5 forest carbon payments, and R&D expenditure accrue to China, Nigeria, India, Indonesia, and the European Union. The share of benefits accruing to low-income, lower-middle-income, and uppermiddle-income countries increases from roughly 70 to 80 percent if investment in R&D is incorporated into the policy response. — The political economy between countries poses a challenge. Seventy-eight percent of the global population and 90 percent of the world’s GDP in 2030 will be in regions that see net economic gains. The remaining regions see a decline in GDP and could resist a push for coordinated reforms. — The more countries cooperate, the better the outcomes: if all countries cooperate and simultaneously adopt nature-smart policies, the world as a whole stands to gain. This means that with appropriate compensatory payments, all countries stand to gain. Accounting for climate change mitigation services considerably increases the number of countries benefiting under all the policy scenarios. — Within-country analysis of the distributional effects shows that policy reforms tend to have a positive impact on labor wages and a negative impact on returns to land. — The analysis demonstrates that nature-smart policies are also climate-smart policies. 62 The Economic Case for Nature 5.1 Depar ng om business-asusual: Policy ins uments for sustainable land use The draft post-2020 global biodiversity framework, which is expected to be adopted in Kunming, China, at the COP-15 of the CBD, calls for urgent, transformative action on nature loss. The framework recognizes that urgent policy action globally, regionally, and nationally is required to transform economic, social, and financial models so that the trends that have exacerbated biodiversity loss stabilize by 2030 and allow for the recovery of natural ecosystems in the following 20 years, with net improvements by 2050, to achieve the CBD’s vision of “living in harmony with nature by 2050” (CBD Secretariat 2020a). The draft of the post-2020 global biodiversity framework stresses that a whole-of-government and whole-of-society approach is necessary to implement the systematic changes that are needed over the next 10 years. Countries need to internalize the value of nature in decisions at all levels and recognize the cost of inaction. The transformative actions that are required include putting in place tools and solutions for implementation and mainstreaming, reducing threats to biodiversity, and ensuring that biodiversity is used sustainably to meet people’s needs. Enabling conditions and adequate means of implementation, including financial resources, capacity, and technology, are needed to support these actions. Internalizing the value of nature in decisions at all levels is a pre-condition for transformative change. As discussed in section 1, market, policy, and institutional failures are enabling the proximate drivers of nature loss. They misalign the private and social costs and benefits of the use of nature, often to the point that a negative price tag is placed on nature’s goods and services. This in turn promotes unsustainable production and consumption practices, depleting and degrading nature. Examples of the latter include extensive expansion of the agricultural frontier and overexploitation of fish stocks. An immediate opportunity for countries to align policy with sustainable management of biodiversity and ecosystem services is to reform the subsidies that are currently provided to agriculture and other sectors, so that support is decoupled from the production or consumption of specific goods or services and instead transferred as a lump sum to economic agents.25 A recent analysis of agricultural policy found that policies that are potentially most economically distorting and most environmentally harmful provide the majority of agricultural 25. The literature suggests that the most distorting forms of support—market price support, payments based on output, and payments based on unconstrained variable inputs—generally have a negative impact on farm technical efficiency and productivity, providing incentives to maintain marginal land in production (OECD 2020b). In contrast, payments that are decoupled from production allow price signals to reach farmers and keep efficiency incentives; they do not generally affect incentives at the intensive or extensive margin, although they may affect incentives at the entry-exit margin and impact production and trade through risk-related effects. 5 | Policy response and the political economy 63 support (OECD 2020b).26 It is also estimated that governments spend at least $500 billion annually on fiscal support to agricultural producers, forestry and fisheries, and fossil fuels that is potentially harmful to biodiversity (OECD 2020c). For example, in 2017, 76 predominantly Organisation for Economic Co-operation and Development (OECD) and G-20 economies spent $340 billion on fossil fuel support (OECD and IEA 2019). In the same year, OECD countries provided $228 billion in support to farmers, of which $116 billion could be considered harmful to biodiversity (OECD 2020a). More than half of the global subsidies to fisheries, estimated at $35 billion per year, is for fuel support and results in overfishing (Sumaila et al. 2016). The experiences of countries such as Mexico, Indonesia, India, and Brazil demonstrate that such policies encourage unsustainable production practices (OECD 2020a) because they amplify market failures, further encouraging underpricing of biodiversity risks and value in private investment, production, and consumption decisions. Another priority area is the creation of incentives for the conservation of nature, for example by leveraging the climate change mitigation services of forests. Maintaining and managing forests for carbon sequestration, for example through initiatives such as Reducing Emissions from Deforestation and Forest Degradation (REDD+),27 represents an increasingly relevant opportunity to harness some of the public good services of forests and incentivize their protection through the sale of carbon credits and offsets in voluntary or regulated carbon markets. These efforts can also be effective in protecting biodiversity. Research demonstrates the existence of spatial trade-offs in allocating funds to protect forests for carbon and biodiversity (Venter et al. 2009). It also shows that while cost-effective spending under REDD+ would protect relatively few species of forest vertebrates, because the trade-offs are nonlinear, minor adjustments to the allocation of funds could double the biodiversity protected, while reducing carbon outcomes only marginally. The World Bank supports the implementation of the REDD+ mechanism in client countries through the Forest Carbon Partnership Facility Readiness Fund, which currently targets 36 countries, and the Carbon Fund, which targets 18 countries.28 Increasing public agricultural R&D investments is also one of the key levers that policymakers can use to alter the trajectory of land use change. Growing demand for agricultural commodities is one of the major drivers of land use change, and a key to dampening agricultural land use expansion is productivity growth. Burney, Davis, and Lobell (2010) illustrate that meeting the world’s needs in the absence of yield growth between 1961 and 2005 would have required an additional 1,761 million hectares of cropland. Using the GTAP model, Stevenson et al. (2013) calculate that an additional 17.9 million to 26.7 million hectares of cropland would have been needed in 2004 if the crop yield gains from the Green 26. The report estimated that market price support, together with payments based on production quantities or the unconstrained use of variable inputs such as fertilizers, accounts for more than half of all transfers to and from the sector, or 70 percent of transfers to and from individual producers. 27. Reducing Emissions from Deforestation and Forest Degradation, plus the sustainable management of forests, and the conservation and enhancement of forest carbon stocks (REDD+), https://redd.unfccc.int/. 28. As of June 30, 2020, there were 47 countries in the REDD+ Readiness Fund (of which 36 have programs that the World Bank has implemented and 10 that other development partners have implemented) and 18 countries in the Carbon Fund (FCPF 2020). 64 The Economic Case for Nature Revolution had not occurred. Agricultural productivity relies heavily on sustained growth in R&D investments (Fuglie 2017), which could even mitigate the adverse impact of climate change on yields (Baldos, Fuglie, and Hertel 2020). In addition to promoting conservation and sustainable use of nature’s services at the extensive margin (by avoiding expansion of the agricultural frontier), agricultural R&D may also promote sustainable practices at the intensive margin (by avoiding the negative effects of intensification through nature-based solutions or reduced use of chemical fertilizers or pesticides, for example), especially if it is coupled with training and knowledge sharing of mainstream best practices (Pretty and Bharucha 2014). R&D also generates spillovers, favoring technology adoption beyond the locality for which it was originally intended (Fuglie 2017), and this provides additional justification for public spending on R&D. The fifth edition of the Global Biodiversity Outlook (GBO5) also points at eight key transitions. The GBO5 points to the relevance of the policy reforms identified above. Among the five essential areas of action needed to reduce the rate of nature’s decline (to “bend the curve”29 of biodiversity loss), the GBO5 identifies enhanced conservation and restoration of ecosystems and more sustainable production of goods and services, especially food. In addition, the GBO5 looks at eight key transitions across a range of human activities that need to shift away from BAU. They are (i) the land and forests transition (which includes conserving intact ecosystems and employing landscape-level planning to avoid, reduce, and mitigate land use change); (ii) the sustainable freshwater transition; (iii) the sustainable fisheries and oceans transition; (iv) the sustainable agriculture transition (which includes enhancing productivity through agro-ecological and other innovative approaches); (v) the sustainable food systems transition; (vi) the cities and infrastructure transition; (vii) the sustainable climate action transition (which includes employing nature-based solutions); and (viii) the biodiversity transition, including the OneHealth transition (CBD Secretariat 2020b). Some of these actions are reflected in the policy scenarios used in this analysis. 29. “Bending the curve” refers to reversing the downward-sloping trends in biodiversity indices observed since the 1970s—the declining species abundance over time (Mace et al. 2018; IPBES 2019; CBD Secretariat 2020b). 5 | Policy response and the political economy 65 5.2 Policy reform scenarios analyzed Three basic policies and a set of policy combinations are analyzed in this report (Figure 15). The policy experiments considered focus on terrestrial ecosystems.30 The basic policies considered are the provision of decoupled support to farmers (by substituting agricultural subsidies linked to production with lump sum transfers to landowners), the establishment of domestic forest carbon payment schemes, and the establishment of a global forest carbon payment scheme. The following is an overview of these policies (see also table 4): • P1 | Decoupled support to farmers.31 The reform of agricultural subsidies is often advocated as a crucial intervention to mitigate land use change—one of the key drivers of nature loss (OECD 2020a, 2020b; Dasgupta 2021). The draft post-2020 global biodiversity framework includes a target on redirecting, repurposing, reforming, or eliminating incentives that are harmful for biodiversity (proposed Target 17). In 2010–19, according to OECD estimates, government support to agricultural producers averaged some $417 billion per year for OECD and selected non-OCED countries. This included market price support, coupled budget transfers, and decoupled budget transfers. Focusing on coupled budget transfers (and in particular on the intermediate input and output subsidies paid to the agriculture sector), this analysis models policy reform by zeroing in on all the corresponding subsidy rates (as defined in the GTAP 10 database, also based on the OECD) and by reallocating the “savings” as lump sum transfers to landowners.32 The budget for subsidy repurposing used in this report is based on the intermediate input and output subsidies paid to the agriculture sector. Appendix G contains further information on the country coverage of the GTAP subsidy data and how the cumulative value of the transfers to be decoupled is estimated. • P2 | Domestic forest carbon payments. Global carbon payments require international transfers. These are globally optimal but politically challenging. Under domestic forest carbon payments, each country funds its own payment scheme. Domestic forest carbon payments are implemented within each model region by limiting land supply while compensating landowners via increases in land subsidies. • P3 | Global forest carbon payments. There is a growing global consensus on the need for a price on carbon. Nature-based solutions, which include sustainable management of forests, could provide an estimated 37 percent of cost-effective climate change mitigation opportunities through 2030 (Griscom et al. 2017). In the model, a global forest carbon payment is implemented using a global pool 30. The analysis presented in this report does not include policies specifically targeted at improving the management of fisheries. This is a key area for follow-up work. 31. Decoupled support to farmers does not depend on current or future production. For a policy measure to be deemed decoupled, that production (or trade) should not differ from the level that would have occurred in the absence of the measure (OECD 2020a). Reforming agricultural policies in this way can reduce interference with production decisions (OECD 2006). 32. It is important to note that the true scale of subsidies is difficult to ascertain. Some subsidies can be observed directly, and others are hidden within budgets and make the task of accounting for them very difficult. 66 The Economic Case for Nature approach. Contributions from developed countries, based on historical greenhouse gas emissions, are pooled and allocated to developing countries, which, in turn, constrain land use change by an amount equivalent to the carbon payment. The scenarios also include combinations of policies involving a change in the agricultural subsidy regime and forest carbon payments. These include the case in which the decoupled support to farmers is coupled with a domestic forest carbon payment scheme (P4 in figure 15). That is, the financial resources freed up by the subsidy are returned to landowners in exchange for the protection of forest carbon sinks. Another option considered is the simultaneous application of decoupled support to farmers and a global forest carbon payment scheme (P5), in which international financial transfers from developed to developing countries are provided against verified forest carbon emission reductions. The final set of scenarios includes an increase in public spending on agricultural R&D. Publicly funded R&D is combined with decoupled support to farmers to obtain a new policy scenario (P6 in figure 15), and the latter is further combined with the global forest carbon payment scheme (P7). In the model, the R&D policy is implemented by zeroing the output and input subsidy rates in agriculture and reallocating the “savings” as follows: (i) an amount equivalent to 20 percent of the savings is invested in R&D;33 and (ii) an amount equivalent to the remaining 80 percent of the savings is reallocated as a lump sum transfer to landowners. Change in agricultural total factor productivity (TFP) is computed using key elasticities and R&D TFP models (Baldos, Fuglie, and Hertel 2020). Appendix F provides more details. The policy scenarios considered all assume a cooperative approach, through domestic policies or via global transfers. The policy experiments considered here are designed to assess the costs and benefits of multilateral cooperation. Hence, it is assumed that all countries implement the policy scenarios in a coordinated way. As the model results show, the whole world stands to gain if all countries cooperate. However, in the absence of a well-defined enforcement mechanism, cooperation will be made difficult by the incentive countries may have to free ride and not comply with the agreement (Barrett 1994). This type of challenge is not analyzed in this report. In terms of financial transfers, most of the policy scenarios considered do not require transfers between countries (for example, decoupled support to agriculture is provided by each country based on its own baseline support to agriculture). Only a limited number of policy scenarios (P3, P5, and P7) consider a global forest carbon results-based payment from developed to developing countries. The policies included represent a subset of a wide range of policy pathways that could be used to reconcile nature and economic development. Examples of such policies that are not analyzed here include food demand-side management, to reduce consumption of animal-based foods, increase plant-based foods, reduce overconsumption, and disincentivize extensification of agriculture production; reduction of loss and waste along the food supply chain and promotion of circular economy approaches; as well as border adjustment taxes to disincentivize consumption of 33. In the current modeling setup, it is assumed that R&D investments are costless. This is due to the fact that the GTAP-AEZ model does not have a specific R&D sector. To mitigate an overestimate of the economic benefits of the policy, GDP in the relevant policy scenarios is adjusted ex post by an amount equivalent to the annualized estimate of R&D expenditure in 2030. This likely leads to a conservative estimate of the policy’s benefits as it does not take into account the multiplier effects of the increased public spending on R&D. 5 | Policy response and the political economy 67 goods with a heavy footprint on nature. Agricultural subsidy reform can also take different forms, such as simple removal of subsidies that are harmful to biodiversity.34 Likewise, the “savings” from agricultural subsidy reform could be channeled not only into agricultural R&D, but also into broader provision of sectoral public goods and services. Potential areas for investment include general R&D, infrastructure (for example, transport, information, and communication technologies), and education, which may also yield high economic returns while supporting sustainable productivity growth in agriculture (OECD 2020b). While by no means exhaustive, the interventions analyzed in this report can be considered representative of a broader range of instruments aimed at addressing policy failures (for example, decoupling subsidies to eliminate perverse incentives) and market failures (for example, payment for ecosystem services to internalize the value of ecosystem services, and investment in R&D to remedy the inability of markets to provide broad access to higher-yield agricultural technology). Figure 15. Schematic overview of the policy scenarios 34. This analysis focuses on decoupling of agricultural support, which tends to be associated with fewer trade-offs and more favorable political economy than alternative policies such as removal of subsidies (OECD 2017, 2020b). These considerations are important because agricultural support can be granted on the basis of valid policy goals, such as poverty reduction, and it is important to ensure that any reform does not carry negative distributional impacts on the poor or lead to unintended outcomes. 68 The Economic Case for Nature Table 4. Description of the policy scenarios # Policy intervention Rationale/hypothesis Policy scenario analyzed Expected insights for policymakers P1 Decoupled P4 fiscal support to farmers P5 P6 P7 Agricultural subsidies (such as support to prices, input, or output) can be distortionary— they can encourage land use change and thus be harmful to biodiversity and ecosystem services. Existing input and output subsidy rates in the GTAP model are brought to zero; the “savings” are then reallocated to owners of agricultural land in all countries as lump sum transfers. Can agricultural policy reform be welfareenhancing in a way that reduces deforestation and the associated loss of biodiversity and ecosystem services? P2 Forest carbon P3 payment mechanisms P4 P5 P7 Land use change and degradation often results from the public good nature of ecosystems and the absence of markets for the (positive) externalities they generate and, as a result, these ecosystems are underprovided. Payment for ecosystem services mechanisms, voluntary or regulated carbon markets, can mimic a market for these services and internalize the positive impacts of conservation. Forest carbon mechanisms are analyzed at two levels: a) Global—where payment is made by high-income countries based on historical emissions and payment is received by countries according to the avoided deforestation. b) Domestic—where payments are made within each model region by limiting land supply while compensating landowners via increases in land subsidies. To what extent can forest carbon payments disincentivize change in land use/loss of natural ecosystems? P6 Public Land use change is R&D policy is How cost-effective is it P7 spending on driven in some countries implemented by agricultural R&D by low agricultural zeroing the subsidy to invest in agricultural intensification (for productivity. If access rates in agriculture example, $ per hectare to agricultural inputs is and reallocating the of deforestation avoided, difficult or too expensive, “savings”: (i) 20 percent compared with business- expanding the areas of of the savings is invested as-usual)? production is likely the in R&D, and (ii) 80 best path to increase percent of the savings is output (particularly reallocated as lump sum when forested land transfers to landowners. is abundant). Note: This report also analyzes the scenario where protected areas are extended to 30 percent of global terrestrial surface (see section 6). GTAP = Global Trade Analysis Project; R&D = research and development. 5 | Policy response and the political economy 69 5.3 Results: Win-win policy solu ons that enhance ecosystem services provision and economic outcomes in 2030 E ect on land use change All the policies analyzed have a positive effect on natural land conservation. Providing decoupled support to farmers decreases natural land loss by 8 percent over 2021–30, corresponding to sparing nearly 4 million hectares, mostly in Oceania, Brazil, the Russian Federation, and Indonesia, which together account for 55 percent of the avoided conversion. Other policies are substantially more impactful in terms of avoiding land conversion. Domestic and global forest carbon payments reduce natural land loss by 26 percent (12 million hectares) and 35 percent (16 million hectares), respectively. Combining policies provides even greater benefits, as shown in figure 16. Combining the agricultural subsidy reform with forest carbon payments enhances the impacts of such policies implemented in isolation. As would be expected, however, the combined result is lower than the arithmetic sum of the individual policies, owing to some redundancies in the incentives. In the scenario where the global forest carbon payment scheme is combined with the domestic reform to decouple support to farmers, 28 percent of natural land loss is avoided (P4 in figure 16), and eight countries, including the United States, Indonesia, the Russian Federation, Angola/the Democratic Republic of Congo, Mexico, Brazil, and Malaysia, account for 63 percent of the avoided loss. Investing in R&D substantially improves environmental outcomes, particularly if it is part of a comprehensive and coordinated policy action. The most impactful policy, among those analyzed, is the combination of decoupled support to farmers, redirecting part of the savings toward R&D with the global forest carbon payment scheme (P7 in figure 16). The policy halves the projected loss of natural land, corresponding to sparing 23 million hectares. Eight model regions (the United States, Indonesia, the Russian Federation, Oceania, Brazil, Angola and the Democratic Republic of Congo, Mexico, and South Africa) account for 65 percent of the land saving. Even without the global forest carbon payment scheme, the decoupled support to farmers that redirects part of the savings toward R&D produces a major improvement relative to the case in which savings are not redirected to R&D but provided as a lump sum to landowners. Adding R&D improves the environmental impact of the fiscal reform by 12 percentage points, corresponding to 5.8 million hectares. 70 The Economic Case for Nature Figure 16. Change in global GDP and avoided conversion of natural land compared with business-as-usual, by policy Note: BAU = business-as-usual; GDP = gross domestic product; R&D = research and development. 5 | Policy response and the political economy 71 The policies considered here lead to a net increase in biodiversity at the global level relative to the BAU scenario. The index developed for this work (see Appendix D), which combines information on species richness, available habitat for endemic species, available habitat for red list species, and habitat in key biodiversity areas, shows a net increase globally. The biodiversity outcomes show considerable heterogeneity among regions. For example, the biodiversity index decreases in lower-middle-income countries for scenarios without the global forest carbon payment program. High-income countries experience a very slight decrease in the biodiversity index under the global forest carbon payment scheme and a slight increase in the biodiversity index under the domestic forest protection scheme. Economic e ects The policies considered not only help reduce the conversion of natural land, but also result in improved global economic outcomes. Compared with BAU, the policies increase GDP in the range of $20 billion to more than $100 billion; and, when including the economic benefits from the enhanced carbon sequestration of forests (valued using the social cost of carbon according to Tol (2008)), the range of increase is between $50 billion and $150 billion (Table 5). Table 5. Economic effects of the policies (change in 2030 GDP relative to BAU, US$, billions) Scenario P1: Decoupled support to farmers P2: Domestic forest carbon payment P3: Global forest carbon payment P4: Decoupled support + Domestic forest carbon payment P5: Decoupled support to farmers + Global forest carbon payment P6: Decoupled support to farmers + R&D P7: Decoupled support to farmers + R&D + Global forest carbon payment INCREASE IN 2030 GDP COMPARED WITH BAU ($, BILLIONS) Excluding carbon emission reduction benefits Adding carbon emission reduction benefits 29.4 56.5 24.3 49.6 19.0 53.4 26.0 53.1 21.7 57.8 109.1 100.7 141.7 147.9 Note: BAU = business-as-usual; GDP = gross domestic product; R&D = research and development. 72 The Economic Case for Nature Focusing on the economic benefits including carbon emission reduction, figure 16 plots, for each policy, the increase in GDP against the avoided land conversion, relative to the BAU scenario. The three basic policies considered increase GDP by $50 billion to $56 billion, with the decoupled support to farmers having the greatest economic impact (+$56 billion), owing to the increased allocative efficiency made possible by decoupling economic support from production. In this case, economic benefits accrue in particular to China, India, the Rest of Middle East and North Africa, the United States, and the European Union. The resultsbased forest carbon payments are much more effective in protecting land, but they provide slightly lower GDP benefits. Combining the decoupled support to farmers with the carbon payment schemes enhances the effect of the policy, in terms of conservation and GDP increases. Investing in R&D results in substantial economic benefits, particularly in developing countries, in addition to the conservation benefits noted above.35 The policy scenario that combines the global forest carbon payment scheme with the decoupled support to farmers with partial repurposing to R&D generates $148 billion in net gains globally. As much as 55 percent of the economic benefits accrue to China, Nigeria, India, the European Union, and Indonesia. Adding R&D investment to the policy mix not only enhances the economic benefits globally, but also ensures that a good share of the economic benefits accrue to low- and middle-income countries. It is important to stress that these impacts reflect the synergistic effect of combining the benefits of R&D (via an increase in TFP) and the benefits of decoupling agricultural farmer support (via the elimination of a distortion). However, comparing the pairs of policies with and without R&D shows the substantial incremental effect of investing in R&D, particularly in developing countries. Low-income, lower-middle-income, and upper-middle-income countries see net GDP increases of $41 billion in the policy scenario without R&D investment (P5), and $119 billion in the scenario with R&D investment (P7). The share of the total benefits accruing to these countries increases from around 70 percent (under P5) to 80 percent (under P7). This is shown in figure 17. The figure also demonstrates that the gain from R&D investment is high especially in the poorer countries, which has important implications for reducing poverty as well as promoting food security. The reason for the relative success of increasing public investment in R&D in economic outcomes is that the benefits spill over to other economic sectors of production, an effect that is not systematically noticed in other policy reforms.36 Greater investment in R&D contributes to an increase in the outputs of all economic sectors, including manufacturing and services, in most countries. 35. The modeling framework used does not prevent economic agents from responding to increases in agricultural productivity to clear more land (except when specific payment for ecosystem services schemes are modeled). What explains the fact that a rebounding effect on deforestation (or Jevons paradox, as it is often referred to) is not observed in the results is that in the modeled scenario, all countries implement the policy (for example, an increase in R&D). This allows a global increase in agricultural productivity, shifting the supply curve downward and boosting output. If countries were to increase production further via expanding cultivated area, this would end up depressing prices and, at some point, the gain on the quantity side would be offset by a loss on the price side. If only a subset of countries were to invest in R&D, relative prices for crops would remain close to their pre-policy level and hence maintain the incentive to clear land. 36. Another policy to consider would be gradual phasing out the subsidies rather than just a decoupling, under which higher returns to capital may indeed compensate landowners for the phasing out of the subsidy. 5 | Policy response and the political economy 73 Returns to the factors of production also increase across the board. The same is not true for other policy reforms (see Box 6 for a decomposition of the drivers of GDP change across policies). In general terms, alternative policies tend to increase the outputs for cotton, sugar crops, forestry, manufacturing, and services, while they may cause a decrease in output for one or more of the following: livestock, oilseed, paddy rice, and wheat. Decoupled support to farmers in the form of lump sum subsidies decreases output for livestock and paddy rice. Adding global forest carbon payments to this policy also decreases the output of oilseed. Adding the domestic forest carbon payment instead also decreases wheat, in addition to livestock, paddy rice, and oilseed. Figure 17. Economic effect of including R&D investments in policy scenarios, by country income group High income countries Low and middle income countries $90B could be added in real GDP change with R&D 16.5 41.3 No R&D +$12B 16.5 +$78B in real GDP change for low- and middle-income countries with R&D 41.3 With R&D Note: The scenario “No R&D” presented here corresponds to P5—decoupled support to farmers combined with global forest carbon payments; the scenario “With R&D” corresponds to P7—decoupled support to farmers combined with global forest carbon payments and R&D investment. Middle-income countries include the lower-middle-income and upper-middle-income brackets. BAU = business-as-usual; R&D = research and development. 74 The Economic Case for Nature Box 6. Drivers of change in the integrated ecosystem-economy model Changes in sector outputs, gross domestic product (GDP), welfare, and other economic variables are the result of the evolution of exogenous parameters, such as population growth and climate change, policy reforms, and endogenously determined changes in ecosystem services. These interact with each other in complex ways through adjustments in market prices and the quantities of goods and services produced, consumed, and internationally traded. A decomposition analysis looking at the relative contributions of key factors, namely, changes in ecosystem services and policy changes, was conducted. By comparing the relative importance of ecosystem services and policy actions to specific economic indicators, the analysis is able to identify trends in terms of where and to whom the benefits of nature (and of human intervention) accrue. At a global scale, changes in GDP across the policy scenarios are driven in varying degrees by the policy impacts on economic productivity and the impacts such policies have on ecosystem services (Figure B6.1). The policy impacts on underlying economic productivity are strongest in the case of increased investments in research and development, accounting for over 40 percent of the total impact. The forest carbon payment schemes result in a negative economic impact on underlying economic productivity. As expected, all the policy changes result in positive impacts through the boost in ecosystem services. The impacts in this case are comparable across the various policy scenarios, with higher contributions to GDP under all the global forest carbon payment schemes (via the enhanced carbon sequestration benefits). Failure to adopt nature-smart policies exposes the world to the risk of a double loss. It means not only forgoing the net GDP gains that could be reached in 2030 if the current levels of ecosystem services provision are maintained or enhanced, but also exposing the planet to the risk of ecosystem collapse and the associated economic losses (see section 4). If the latter materializes, the same policies that today offer the double dividend of nature conservation and GDP gains would end up being largely ineffective: the estimated loss in 2021–30 GDP growth prevailing under the tipping point scenario is on the order of 9.5 percent or $2.8 trillion (compared with BAU), both with and without adoption of the policies. The COVID-19 pandemic is a striking example of the potential trade-offs involved in delaying action on risks related to environmental degradation. According to new analysis, the net costs of actions to prevent future zoonotic pandemics range from $18 billion to $27 billion per year (Dobson et al. 2020). This contrasts sharply with the economic damages caused by the COVID-19 pandemic. In 2021 alone, the pandemic is projected to reduce global GDP by 5.3 percent or $4.7 trillion compared with pre-pandemic scenarios (World Bank 2021). 5 | Policy response and the political economy 75 Figure B6.1. Change in 2030 global GDP, by policy and contributing drivers Change in 2030 real GDP (US$, billions) P1| Decoupled farmer support 57 P2| Domestic forest carbon payment 50 P3| Global forest carbon payment 53 P4 (P1 + P2) 53 P5 (P1 + P3) 58 P6 (P1 + R&D) P7 (P1 + P3 + R&D) Ecological drivers Policy drivers Other drivers 142 148 Poli cal economy of a global deal for nature As CBD Parties around the world strive to implement more ambitious policy reform for the next decade, they may encounter political economy barriers that could hamper progress. Resistance is often driven by concerns about the impact of policy reform on the competitiveness of a sector or country. For example, policy reforms that aim at enhancing protected areas may be perceived to increase the cost of land and hence make a country’s agricultural products less competitive on international markets. Often, the resistance to reform conceals vested interest in the status quo and may be at odds with the interests of other segments of the population, for example local communities and urban dwellers. OECD (2017) points at the importance of using a robust evidence base to build broad support for reform, identifying synergies between economic and environmental interests, and devising measures to compensate the “losers” of policy reform. This section illustrates qualitatively where the key tension points may be. The distributional effects of the policy scenarios are analyzed by focusing on two sets of comparators. First, the section looks at differences in GDP impacts across countries and regions. As the results below show, most countries stand 76 The Economic Case for Nature to gain, relative to BAU, from the coordinated adoption of the policy reforms analyzed. Second, the analysis looks at the differences in impacts on factor returns to unskilled labor, skilled labor, capital, and land within countries. By doing so, it is possible to analyze the prospects for policy adoption and the source of resistance at the country level and provide a measure of the extent of compensation that may be required to make progress on the nature agenda. Between-coun y comparison Although at the global level the case for adopting nature-smart policies is clear, some countries may see a decline in real income. To evaluate the attractiveness of any given policy scenario to any given country, the analysis in this report compares what happens to each GTAP country unit’s GDP in 2030 relative to the counterfactual without the policy scenario (that is, the BAU scenario). The vast majority of country units appear to gain from all seven policies analyzed (Table 6). The policies that produce the largest shares of “losers” are those that introduce a global forest carbon payment (P3 and P5).37 In such cases, the policies result in a decline of GDP for 10 GTAP country units. In 2030, these regions will account for 22 percent of the world’s population, but only 10 percent of the world’s GDP. The countries that benefit from the adoption of a global forest carbon payment scheme include the largest economies in the world: China, the United States, the European Union, Japan, India, the Russian Federation, and Brazil. Other policies have much-improved prospects for being adopted widely. Globally coordinated decoupled support to farmers increases GDP in 31 of the 37 GTAP country units, representing 82 percent of the world’s population and 94 percent of global GDP in 2030. Decoupled support restores competitive balance in the global agricultural market, thereby benefitting developing countries the most, except in the Middle East and North Africa region, which initially subsidizes agriculture sectors. The policies with the highest number of winners are those involving an increase in public investment in R&D. Under such policy scenarios, 99 percent of the world’s population and 97 percent of the world’s GDP are in countries that gain from the adoption of the policy. Access to new technologies boosts agricultural productivity and lowers agricultural commodity prices across the world, with virtually all developing countries gaining from the reform. If the carbon sequestration services of nature are considered, the policy reforms substantially boost the number of countries that are likely to be in favor. The analysis above is done based on GDP changes before the benefits of avoided damages from land-based greenhouse gas emissions are considered. Protecting natural land cover results in an enhanced ability of forests to absorb greenhouse gas emissions. The consequential climate change mitigation benefits 37. To explain why some country units lose with certain policies, it is useful to note that the limits imposed on land use by the forest carbon payment scheme may negatively affect output by reducing the amount of land available for agriculture. Eliminating subsidy distortions can have the opposite effect by reducing an inefficiency, but this is not strong enough to counter the impact on land restrictions. When designing a policy, these effects may be difficult to predict ex ante and require the type of modeling presented here, to design incentive compatible reforms. 5 | Policy response and the political economy 77 considerably increase the number of regions that benefit from the reform. The gains are highest when a global forest carbon payment scheme is established. For example, when the global forest carbon payment is combined with the policy that decouples support to farmers, the number of country units that benefit from the policy reform, after considering the carbon sequestration benefits, increases from 27 to 34 (of 37). Weighted by GDP in 2030, this represents an increase in the GDP produced in countries that gain from the reform from 90 to 96 percent of the world’s total. Like other findings of this report, this shows that nature-smart policies have significant climate co-benefits, underscoring the fact that the two agendas are complementary and there are significant synergies to be exploited. Table 6. Share of the world’s economy benefitting from adoption of policies, compared with business-as-usual, in terms of (A) GDP, (B) population, and (C) number of GTAP country units Policy P1 P2 P3 P4 P5 P6 P7 A. Relative to 2030 GDP under business-as-usual (%) Excluding carbon sequestration benefits 94 92 90 94 90 97 97 Including carbon sequestration benefits 98 97 96 97 96 100 98 B. Relative to population in 2030 (%) Excluding carbon sequestration benefits 82 80 78 82 78 99 99 Including carbon sequestration benefits 99 99 96 99 96 100 99 C. Number of GTAP country units Excluding carbon sequestration benefits 31 29 27 30 27 35 35 Including carbon sequestration benefits 36 35 34 35 34 37 36 Note: P1:Decoupled Support to Farmers; P2: Domestic Forest Carbon (FC) payment; P3: Global FC payment; P4: Subsidy reform + Domestic FC payment; P5: Decoupled Support to Farmers + Global FC payment; P6: Decoupled Support to Farmers + Agricultural R&D; P7: Decoupled Support to Farmers + Agricultural R&D + Global FC payment 78 The Economic Case for Nature Within-coun y comparison Distributional impacts are another common issue in introducing environmental reform. The distributive elements of policies to control externalities are of great interest in a world in which poverty reduction and inequality are still an unfinished agenda (Baumol and Oates 1988). Protecting nature may hinder the ability of natural resource owners to capture the rents from the exploitation and transformation of the asset, but because the policies boost the demand for more efficient use of the resource, they may benefit returns to capital and even returns to labor. Concerns about the inclusive and equity aspects of policies can affect the political feasibility of reforms and cause these policies to be supported or challenged by public resistance. Mackie and Haščič (2019) argue that well-designed environmental policy reforms incorporating appropriately targeted compensation schemes (for example, within existing social welfare systems) can neutralize direct negative distributional outcomes and generate progressive outcomes overall. Similarly, Liu and Kontoleon (2018), through a meta-analysis, show that payment for ecosystem services programs are likely to have positive but modest livelihood impacts on ecosystem services suppliers; however, several institutional characteristics of payment for ecosystem services are found to be correlated with more favorable livelihood impacts. The literature on climate change yields a wide range of estimates of net benefits of carbon mitigation policies. Fullerton (2008) shows that if emission abatement technologies are capital intensive, then any mandate to abate pollution may induce firms to use more capital. If demand for capital is raised relative to labor, then a lower relative wage may hurt low-income households. Equity considerations can also be an enabler of ambition for accelerated mitigation (HoeghGuldberg et al. 2019) and enhance social acceptance of mitigation efforts (Klinsky and Winkler 2018). By using an impact decomposition technique, this report analyzes how the different policies considered affect returns to different factors of production. The returns to factors of production analyzed are interest payments to capital, land rents, wages to skilled labor, and wages to unskilled labor (see appendix H for details). The model allows for estimating the percentage change in factor returns between 2021 and 2030, by GTAP country unit. Under BAU, the return to land increases by 87 percent on average (with 90 percent of regions seeing an increase in returns and 75 percent of the regions having an increase in land rents of at least 44 percent between 2021 and 2030). This highlights the world’s increasing reliance on land over the period considered. The highest increase is 348 percent in the Angola and the Democratic Republic of Congo. Returns to labor increase on average by 9.0 percent (skilled labor wages, with changes ranging between -31 and +40 percent) and 9.7 percent (unskilled labor wages, ranging between -26 and +38 percent). Returns to capital decrease in the BAU scenario. On average, there is a decline of 11 percent in the return to capital over 2021–30. This is quite generalized, with 90 percent of the model regions seeing a decline in returns to capital. The policy reforms tend to have a positive impact on labor wages and a negative impact on returns to land. When the policies are implemented, the general evidence is that unskilled labor wages increase under most of the policy scenarios (Figure 18). It is important to note that the real price of land actually increases in most of the policy scenarios, thereby benefitting landowners in absolute terms. However, the real returns to land increase less fast under the 5 | Policy response and the political economy 79 policy scenarios than under BAU. In the case of decoupled support to farmers, there are two effects at play: the reduction in input and output fiscal transfers, which reduces demand for land (lowering its price), and the lump sum transfer to landowners, which increases the demand for land and other inputs (increasing the price of land). The former is dominant, and the net effect is a decline in the price of land. With respect to unskilled labor, the policy reforms tend to reward employment in the primary sectors, thus boosting wages. This effect is more pronounced when decoupled support to farmers, which shifts resources away from agricultural inputs (other than labor), is combined with payment for ecosystem services schemes and R&D. Figure 18. Effects of policy scenarios on returns to factors of production, relative to BAU factors of production for each country unit land capital skilled labor % difference in 2021 - 2030 growth, relative to BAU 20 P1 P2 P3 P4 (P1 + P2) P5 (P1 + P3) P6 (P1 + R&D) unskilled labor P7 (P1 + P3 + R&D) 0 -20 -40 -60 Note: P1: Decoupled Support to Farmers; P2: Domestic Forest Carbon (FC) payment; P3: Global FC payment; P4: Subsidy reform + Domestic FC payment; P5: Decoupled Support to Farmers + Global FC payment; P6: Decoupled Support to Farmers + Agricultural R&D; P7: Decoupled Support to Farmers + Agricultural R&D + Global FC payment 80 The Economic Case for Nature Figure 19. Political economy challenge measured as: (A) Likelihood of each factor of production benefitting from policy scenarios, and (B) Likelihood of a policy scenario benefitting factors of production A more systematic look at the impacts on different factors of production shows that, in addition to unskilled labor, capital and skilled labor also tend to benefit from the policies considered. Figure 19, panel A, ranks the factors of production relative to how many policies benefit them and relative to the country units in which the benefits materialize. The results show a rather generalized decrease in the growth rate of land returns, with landowners being net losers in the various policy scenarios. In as many as 25 of the 37 regions landowners lose under each of the policy reforms. The policies that in some instances provide net gains to landowners are those that implement decoupled support and repurpose the subsidy into lump sum payments or domestic forest carbon payments. Unskilled labor benefits from a broad range of policy reforms in a large number of country units. For 34 of the 37 model regions, there are at least three policies that benefit unskilled workers. These are the policy that implements a domestic forest carbon payment and the two policies that repurpose part of the decoupled support to farmers to increase public investment in R&D. Capital also stands to gain from a large number of the policies in a larger number of countries. In 13 of the 37 regions, the returns to capital are higher under all the policy scenarios. 5 | Policy response and the political economy 81 The polices that will likely face the least political economy tensions are the increase in public R&D investment and the domestic forest carbon payment scheme. Figure 19, panel B, ranks the policy reforms according to the number of country units and the country distribution of factors of production that benefit from the reform. The policy that redirects part of the agricultural subsidies to public investment in R&D (P6) benefits at least three factors of production in 33 of the 37 country units. Adding the global forest carbon payment (P3) benefits at least three factors in 29 country units. For the policy that establishes domestic forest carbon payment schemes financed by general taxation, 32 regions see at least three factors of production benefitting. There are very few instances in which all the factors of production benefit, giving rise to potential political economy challenges. The policies that combine decoupled support to farmers (P1) with the forest carbon payment scheme, whether local (P4) or global (P5), are the ones with the most challenging situation as no factor of production benefits in a relatively large share of the countries (Figure 19, panel B). P1: Decoupled Support to Farmers; P2: Domestic Forest Carbon (FC) payment; P3: Global FC payment; P4: Subsidy reform + Domestic FC payment; P5: Decoupled Support to Farmers + Global FC payment; P6: Decoupled Support to Farmers + Agricultural R&D; P7: Decoupled Support to Farmers + Agricultural R&D + Global FC payment Implica ons for the 30x30 global target 6 84 The Economic Case for Nature Synthesis This report evaluates the opportunity costs of achieving the “30x30” goal (30 percent of the planet protected by 2030) in terms of the GDP that would be forgone in 2030 as a result of protecting additional natural land, rather than letting market forces develop it where profitable. — The cost is estimated at $115 billion (-0.1 percent of global GDP in 2030); if the analysis accounts for the economic benefits of the avoided carbon dioxide emissions, the cost becomes only $13 billion. — Although achieving the goal implies the need to mobilize resources in developing countries to cover the net cost they would 6 | Implications for the 30x30 global target 85 Implica ons for the 30x30 global rget 6 incur (some 1.3 percent of their GDP in 2030), the analysis points to several reasons for optimism—opportunities for broadening the range of nature-smart policies and accounting for a wider range of ecosystem services, the protection of which could result in further GDP benefits that are not quantified in this analysis. 86 The Economic Case for Nature 6.1 What is the 30x30 goal? Negotiation efforts in the lead-up to COP-15 of the CBD have built momentum around the need to protect 30 percent of the planet for nature by 2030, which is often referred to as the “30x30” goal. Although the specific numerical target of the conservation goal is still under intense debate, it is clear that some version of a large-scale land protection plan will need to be discussed even beyond CBD COP-15 and debated for inclusion in future global conservation strategies. Importantly, it will be necessary to define the opportunity cost of such large-scale conservation efforts. One important challenge with implementing this type of policy is the high likelihood of negatively affecting the population of certain countries or areas of special priority for conservation. Existing approaches that seek to quantify the associated opportunity costs, such as Waldron et al. (2020), calculate the direct costs of protecting land but do not include a full general equilibrium assessment at the national or subnational level. Modeling the implemen on of the 30x30 goal The integrated ecosystem-economy model developed for this report is uniquely suited to assess the cost of achieving a large-scale objective such as the 30x30 goal. To complement the policy analysis described in section 5, an additional scenario is analyzed here to assess the opportunity cost of protecting 30 percent of the land on Earth by 2030. The methodology builds on the work by Waldron et al. (2020), which analyzes a similar question but does not capture general equilibrium effects—in particular, the study was not able to track down, across supply chains and countries, the GDP and welfare effects due to removal of land from production. The integrated ecosystem-economy model presented in this report achieves this by directly incorporating a land supply curve that is parameterized uniquely for each GTAP country unit, producing a sophisticated tool to assess this question in a whole-of-economy framework. In a nutshell, the opportunity cost is estimated by: identifying in a spatially explicit manner the priority areas to be protected to achieve the 30x30 goal, evaluating the change in GDP in 2030 relative to the BAU scenario as a result of taking land area out of production, and controlling for variations in land scarcity across the world (see box 7 for more details). Although this initial analysis considers conservation of land only, it should eventually be expanded to include marine ecosystems. 6 | Implications for the 30x30 global target 87 Box 7. Approach taken to model the implementation of the 30x30 goal This analysis assumes that every Global Trade Analysis Project (GTAP) country unit increases the land area under protection by 15.3 percent of the total land area in that GTAP country unit. This means that when it is added to the land already under protection (14.7 percent of the global terrestrial surface area), the 30x30 goal is achieved at the global level. This approach is a midway point between two more extreme solutions, namely: to protect the most valuable land in terms of biodiversity and ecosystem services (which would confer much more protection responsibility to tropical and high–conservation value countries), and to require protection of 30 percent of the land within each country unit (which would mean zero expansion in country units that are already conserving 30 percent of their land). This spectrum is discussed in more depth in Waldron et al. (2020) and is reflected by the range of scenarios defined therein. A minor adjustment of the approach described above was necessary, since the unit of analysis for the computable general equilibrium model includes groups of countries clustered in GTAP “country units.” Some of the GTAP country units have less than 15.3 percent of their land area available for extra protection (this is the case for countries with large conservation efforts already underway). In those cases, the scenario was built by increasing the area under protection by whatever is left to protect in that GTAP country unit, and then slightly increasing the protected area in all the other GTAP country units, to ensure that the global conservation goal is achieved. Appendix I provides details on the resulting area increases for each GTAP country unit. An additional assumption that must be specified is which grid cells within a GTAP country unit should be protected. The Waldron report specifies many different scenarios that address how to prioritize among competing conservation goals. The analysis uses the best performance scenario, the Global Deal for Nature, using biodiversity and carbon storage indicators to identify the highest-value conservation grid cells (appendix I provides details on the geospatial computation steps). However, the Waldron report does not provide spatially explicit maps (or even exact regional totals) of where each scenario protects land, and no such maps are available from other sources. In contrast, the analysis carried out for this report creates spatially explicit maps that implement the Global Deal for Nature scenario with a new level of spatial and regional explicitness. This is described in detail in appendix I. The opportunity cost of achieving the 30x30 goal is assessed by comparing the gross domestic product (GDP) and welfare generated in 2030 with the business-as-usual scenario. The model estimates them as follows: • The 30x30 scenario “shocks” each GTAP country unit by shifting the land supply curve asymptote according to the number of hectares removed from current or potential economic use (see appendix I). • The GTAP-1 run calculates the endogenous change in land use. • The model downscales the endogenous land use change using the Spatial Economic Allocation Landscape Simulator, estimating the changes in the provision of different ecosystem services through the Integrated Valuation of Ecosystem Services and Tradeoffs. • The GTAP-2 run projects the economic impact of the changes in ecosystem services. A key advantage of this modeling approach is that it accurately reflects the physical scarcity (or lack thereof) of land, which varies among regions. For example, if there is land protection in a region that has a large amount of land still unutilized by the economy, this will have little impact on GDP because the elasticity is still very low (that is, producers would easily find other land that is not protected). However, in regions where there is not much land left, constraining the economy not to use newly protected land would have relatively large impacts (because there will be a high elasticity of land as an input factor). This is a level of realism that is not included in methods that apply a fixed price of land as the opportunity cost. Additionally, this is an improvement over earlier efforts that did not consider how economies might be constrained by lack of land inputs (although they may include the increased price of land as a benefit to landowners). 88 The Economic Case for Nature This analysis identified in a spatially explicit way the areas where protection efforts to achieve the 30x30 goal may focus. Map 1 shows currently protected hectares in green and new areas proposed for protection in purple and orange (see appendix I for a detailed presentation of the methods and results). The report identified which areas should be protected by calculating a weighted sum of carbon sequestration potential and biodiversity. The new protected areas in purple show areas that are not on potentially cultivated agricultural lands, and the areas in orange show where there is conflict (that is, areas that in BAU would be converted to production). Map 1. Spatially explicit definition of the 30x30 goal Displaces cropland Newly protected (no conflict) Currently protected 8605 hectares per 5-arc-minute grid cell Most 0 Least land 6 | Implications for the 30x30 global target 89 Insights for CBD nego ators As expected, the 30x30 scenario improves biodiversity outcomes relative to BAU and all the other policies considered. Globally, the increase in the biodiversity index is 29 percent, compared with BAU (see Table D.1 for details). This represents a reversal of biodiversity lost in the BAU scenario (relative to the “baseline” scenario) and a 50 percent improvement beyond that. Moreover, the change in biodiversity outcomes in the 30x30 scenario show very large improvements over the policies calculated without implementation of this goal. For example, 30x30 achieves a global biodiversity improvement over BAU that is almost six times higher than the next most ambitious scenario (subsidies repurposed to R&D with global forest carbon payment). Biodiversity outcomes (as measured by the biodiversity index) improve across all regions and income groups. The 30x30 goal is within reach at a moderate economic cost. When combined with the most conservation-effective of the policy scenarios presented in section 5 (namely P7, decoupled support to farmers with R&D and global forest conservation payments), achievement of the 30x30 goal results in a 0.1 percent decline in global GDP in 2030, compared with BAU (Table 7). Table 7. Economic impacts of achieving the 30x30 goal in terms of GDP, by income group Country group A. Low-income Share of extra protection effort (%) 9 Change in GDP US$, billions -15.7 Percent -1.93 Change in GDP corrected with climate mitigation benefits US$, billions Percent -10.3 -1.30 B. Lower-middle income C. Upper-middle income D. High-income 34 -84.2 -0.83 -46,0 -0.46 38 0.3 0.00 37.7 0.00 19 -15.9 -0.02 -5.1 0.02 Total 100 -115.4 -0.10 -13.4 -0.01 90 The Economic Case for Nature Accounting for the carbon sequestration services of newly protected areas further improves the prospects for a 30x30 deal. The global loss is even smaller when GDP is adjusted for the climate change mitigation benefits of the lower carbon emissions made possible by the extra conservation of natural areas (estimated using the social cost of carbon emissions, from Tol (2008)). From the global perspective, the economic loss caused by restrictions on land use (red arrows in figure 20) is almost entirely offset by the economic gains resulting from improved provision of ecosystem services (green arrows in figure 20). Figure 20. Change in 2030 real GDP under the 30x30 scenario, by income group and driver of change 6 | Implications for the 30x30 global target 91 Since the economic costs of implementing the 30x30 goal vary across countries and income groups, global cooperation is paramount to its successful implementation, and appropriate incentives for such cooperation need to be put in place. To achieve the goal, developing countries would need to be assisted in mobilizing financial resources, as they account for some 80 percent of the new conservation efforts in this scenario (Table 7, first column) and would forgo close to 1 percent of their GDP in 2030 (among low-income, lower-middleincome, and upper-middle-income countries). Conversely, high-income countries account for less than 20 percent of the extra conservation, while incurring a small GDP gain (some 0.02 percent of GDP in 2030). Once again, these findings suggest that the more countries cooperate, the better the outcomes are, and incentives for such cooperation need to be put in place. There are reasons for optimism: this analysis shows that the right mix of incentives and supporting policies could achieve the 30x30 goal. First, pursuing the 30x30 goal in isolation would entail a global GDP loss in excess of $200 billion in 2030, but conversely, if combined with subsidy decoupling and R&D investment, the GDP cost drops by almost a half. Ramping up the scale of the policies accompanying the 30x30 goal (for example, channeling more than 20 percent of the savings obtained from decoupling agricultural support toward agricultural R&D) could further reduce its net GDP impacts. Second, there is a wider range of policies that could be added to the policy response, such as border adjustment taxes to disincentivize consumption of goods with a heavy footprint on nature or incentives to reduce food loss or waste. A broader array of policies can provide countries extra opportunities for identifying win-win options that are better suited to their circumstances, and ultimately getting the world closer to an ambitious conservation target such as 30x30. Third, this analysis only includes a relatively limited set of ecosystem services (pollination, timber provision, fisheries, and carbon sequestration by forests). Quite conceivably, accounting for further services that nature provides to societies (for example, watershed regulation and coastal protection) would demonstrate higher economic benefits from protecting those services. Conclusions and the way forward 7 94 The Economic Case for Nature This report provides new evidence that protecting nature makes economic and environmental sense. The cost of inaction is very high. In what resembles a stress test of the global economy, the model developed for this report analyzes the impact of reaching a number of ecological regime shifts in pollination, forests, and fisheries. If these were to happen, the world’s economy would shrink by 2.3 percent by 2030, with low-income countries (-10 percent in GDP) being hit the hardest and having to give up in some cases as much as 50 percent of their GDP growth rate between now and 2030. This is a low-probability scenario, but the COVID-19 pandemic is an important reminder of the impacts that low-probability yet largescale events can have on economic development. By contrast, it is possible to take action through what this report refers to as “nature-smart” policies. These policies have a double virtue: they protect nature and make the economy more efficient as they enhance the services nature provides to economic activities. The nature-smart policies analyzed in this report are also climate-smart policies. COP-15 of the Convention on Biological Diversity provides a once-in-a-generation opportunity to put the world on a more sustainable and more efficient development path. The preparation of the post-2020 global biodiversity framework is, at the time of writing, well underway. The framework will be designed to address the drivers of biodiversity loss and boost the benefits to people by establishing targets for urgent and transformative action by governments and all of society. The framework will be implemented primarily through activities at the national level, with supporting action at the subnational, regional, and global levels. The framework’s theory of change proposes actions to (i) put in place tools and solutions for implementation and mainstreaming, (ii) reduce the threats to biodiversity, and (iii) ensure that biodiversity is used sustainably to meet people’s needs. The model and insights developed for this report can inform the tools and solutions based on which the framework is designed and be used to maximize synergies and manage the trade-offs between biodiversity and the climate change commitments. The work presented here provides relevant insight for nine of the 20 targets in the draft of the post-2020 global biodiversity framework (Figure 21). Ambition in Kunming means ensuring that political economy issues are tackled with determination. Most policies, in most countries, may face important adoption challenges. Yet, despite net GDP gains for the economy as a whole, in particular for developing countries and less wealthy people, in most countries the policies are likely to result in losses for one or more factors of production (land, capital, and labor). Landowners stand to lose from the adoption of most of the policies, potentially representing strong resistance to reform. Boosting R&D investment and technology adoption can help reduce the gap while increasing the size of the pie to be shared among different parts of society. This is an important lesson to be taken up by negotiators of the post-2020 global biodiversity framework and during implementation of the framework over the next 10 years. Beyond COP-15, there is an important role for economics. Economics is inherently the science that studies the administration of scarce resources to achieve societal goals. Biodiversity and the services nature provides are becoming ever scarcer and so is the capacity of nature to regenerate. There are three important actions for the economics profession to undertake moving forward. The first is to contribute to the development and implementation of natural capital and ecosystem valuation. Building on the advances that the System of Environmental and Economic Accounts has made and the United Nations Statistical Division 7 | Conclusions and the way forward 95 has championed, it is important to develop practical and actionable guidance for integrating ecosystem services into national accounting and estimating the economic value of a wide range of ecosystem services. This should include naturebased solutions such as carbon sequestration, flood and erosion protection, and food security (for example, fisheries and agroforestry). The second is to contribute to assessing policy and investment options. Economic information would provide the basis for development of the economic analyses that are needed to compare investment options such as roads, seawalls, and policy reforms, including land and marine use planning policies at the country level. The availability of such data would also greatly benefit the private sector, helping to inform the decisions of firms and financial institutions at the project and portfolio levels and helping them to engage with sovereigns in an effort to manage natural resources more sustainably. The third action is to support the integration of an economic and finance lens into National Biodiversity Strategies and Action Plans to ensure that they become a tool for national planning to be adopted and harnessed by economic decision makers. A key opportunity for follow-up work would thus be to apply this analysis to country-specific contexts. Figure 21. Post-2020 global biodiversity framework targets for which the model provides relevant insights 96 The Economic Case for Nature Appendices 97 Appendices A B C D E F G H I 98 The Economic Case for Nature Appendix A Coun y and region classi ca on GTAP GTAP GTAP37 226 226 (ISO Index 3 Code) 3 AGO Angola+DRC 41 COD Angola+DRC 9 ARG Argentina 20 BGD Bangladesh 29 BRA Brazil 5 ALB Rest of C Asia 6 AND Rest of C Asia 10 ARM Rest of C Asia 15 AZE Rest of C Asia 24 BIH Rest of C Asia 25 BLR Rest of C Asia 68 FRO Rest of C Asia 72 GEO Rest of C Asia 74 GIB Rest of C Asia 89 HRV Rest of C Asia 103 KAZ Rest of C Asia 105 KGZ Rest of C Asia 124 MCO Rest of C Asia 125 MDA Rest of C Asia 130 MKD Rest of C Asia 176 SCG Rest of C Asia 184 SMR Rest of C Asia 199 TJK Rest of C Asia 201 TKM Rest of C Asia 211 UKR Rest of C Asia 214 UZB Rest of C Asia 35 CAN Canada 1 ABW Central Amer 4 AIA Central Amer 7 ANT Central Amer 12 ATG Central Amer 23 BHS Central Amer GTAP 226 Long Name - ISO 3 Code Angola Congo, the Democratic Republic of the Argentina Bangladesh Brazil Albania Andorra Armenia Azerbaijan Bosnia and Herzegovina Belarus Faroe Islands Georgia Gibraltar Croatia Kazakhstan Kyrgyz Republic Monaco Moldova, Republic of Macedonia, the former Yugoslav Republic of Serbia and Montenegro San Marino Tajikistan Turkmenistan Ukraine Uzbekistan Canada Aruba Anguilla Netherlands Antilles Antigua and Barbuda Bahamas World Bank region Sub-Saharan Africa Sub-Saharan Africa World Bank income group (June 2020) Lower-middle income Low income Latin America & Caribbean South Asia Latin America & Caribbean Europe & Central Asia Europe & Central Asia Europe & Central Asia Europe & Central Asia Europe & Central Asia Europe & Central Asia Europe & Central Asia Europe & Central Asia Europe & Central Asia Europe & Central Asia Europe & Central Asia Europe & Central Asia Europe & Central Asia Europe & Central Asia Europe & Central Asia Upper-middle income Lower-middle income Upper-middle income Upper-middle income High income Upper-middle income Upper-middle income Upper-middle income Upper-middle income High income Upper-middle income High income High income Upper-middle income Lower-middle income High income Lower-middle income Upper-middle income .. Europe & Central Asia Europe & Central Asia Europe & Central Asia Europe & Central Asia Europe & Central Asia North America Latin America & Caribbean .. .. Latin America & Caribbean Latin America & Caribbean .. High income Low income Upper-middle income Lower-middle income Lower-middle income High income High income .. .. High income High income Appendices 99 GTAP GTAP GTAP37 226 226 (ISO Index 3 Code) 26 BLZ Central Amer 27 BMU Central Amer 30 BRB Central Amer 47 CRI Central Amer 48 CUB Central Amer 49 CYM Central Amer 54 DMA Central Amer 56 DOM Central Amer 81 GRD Central Amer 82 GRL Central Amer 83 GTM Central Amer 88 HND Central Amer 90 HTI Central Amer 100 JAM Central Amer 108 KNA Central Amer 115 LCA Central Amer 138 MSR Central Amer 149 NIC Central Amer 158 PAN Central Amer 164 PRI Central Amer 183 SLV Central Amer 186 SPM Central Amer 195 TCA Central Amer 204 TTO Central Amer 215 VCT Central Amer 217 VGB Central Amer 218 VIR Central Amer 38 CHN China 87 HKG China 208 TWN China 44 COL Colombia 59 EGY Egypt 63 ETH Ethiopia 14 AUT EU 17 BEL EU 21 BGR EU 50 CYP EU 51 CZE EU 52 DEU EU 55 DNK EU GTAP 226 Long Name - ISO 3 Code Belize Bermuda Barbados Costa Rica Cuba Cayman Islands Dominica Dominican Republic Grenada Greenland Guatemala Honduras Haiti Jamaica Saint Kitts and Nevis Saint Lucia Montserrat Nicaragua Panama Puerto Rico El Salvador Saint Pierre and Miquelon Turks and Caicos Islands Trinidad and Tobago Saint Vincent and the Grenadines Virgin Islands, British Virgin Islands, U.S. China Hong Kong SAR, China Taiwan, Province of China Colombia Egypt Ethiopia Austria Belgium Bulgaria Cyprus Czech Republic Germany Denmark World Bank region Latin America & Caribbean North America Latin America & Caribbean Latin America & Caribbean Latin America & Caribbean Latin America & Caribbean Latin America & Caribbean Latin America & Caribbean Latin America & Caribbean Europe & Central Asia Latin America & Caribbean Latin America & Caribbean Latin America & Caribbean Latin America & Caribbean Latin America & Caribbean Latin America & Caribbean .. Latin America & Caribbean Latin America & Caribbean Latin America & Caribbean Latin America & Caribbean .. Latin America & Caribbean Latin America & Caribbean Latin America & Caribbean Latin America & Caribbean Latin America & Caribbean East Asia & Pacific East Asia & Pacific East Asia & Pacific Latin America & Caribbean Middle East & North Africa Sub-Saharan Africa Europe & Central Asia Europe & Central Asia Europe & Central Asia Europe & Central Asia Europe & Central Asia Europe & Central Asia Europe & Central Asia World Bank income group (June 2020) Upper-middle income High income High income Upper-middle income Upper-middle income High income Upper-middle income Upper-middle income Upper-middle income High income Upper-middle income Lower-middle income Low income Upper-middle income High income Upper-middle income .. Lower-middle income High income High income Lower-middle income .. High income High income Upper-middle income High income High income Upper-middle income High income High income Upper-middle income Lower-middle income Low income High income High income Upper-middle income High income High income High income High income 100 The Economic Case for Nature GTAP GTAP GTAP37 226 226 (ISO Index 3 Code) GTAP 226 Long Name - ISO 3 Code 61 ESP EU Spain 62 EST EU Estonia 64 FIN EU Finland 67 FRA EU France 71 GBR EU United Kingdom 76 GLP EU Guadeloupe 80 GRC EU Greece 91 HUN EU Hungary 94 IRL EU Ireland 99 ITA EU Italy 119 LTU EU Lithuania 120 LUX EU Luxembourg 121 LVA EU Latvia 132 MLT EU Malta 139 MTQ EU Martinique 151 NLD EU Netherlands 166 PRT EU Portugal 171 REU EU Réunion 172 ROM EU Romania 189 SVK EU Slovakia 190 SVN EU Slovenia 191 SWE EU Sweden 93 IND India India 92 IDN Indonesia Indonesia 102 JPN Japan Japan 109 KOR Korea Korea, Republic of 8 ARE Rest of M East N Africa United Arab Emirates 22 BHR Rest of M East N Africa Bahrain 57 DZA Rest of M East N Africa Algeria 95 IRN Rest of M East N Africa Iran, Islamic Republic of 96 IRQ Rest of M East N Africa Iraq 98 ISR Rest of M East N Africa Israel 101 JOR Rest of M East N Africa Jordan 110 KWT Rest of M East N Africa Kuwait 112 LBN Rest of M East N Africa Lebanon 114 LBY Rest of M East N Africa Libyan Arab Jamahiriya 156 OMN Rest of M East N Africa Oman 168 PSE Rest of M East N Africa Palestinian Territory, Occupied 170 QAT Rest of M East N Africa Qatar 175 SAU Rest of M East N Africa Saudi Arabia World Bank region Europe & Central Asia Europe & Central Asia Europe & Central Asia Europe & Central Asia Europe & Central Asia .. Europe & Central Asia Europe & Central Asia Europe & Central Asia Europe & Central Asia Europe & Central Asia Europe & Central Asia Europe & Central Asia Middle East & North Africa .. Europe & Central Asia Europe & Central Asia .. .. Europe & Central Asia Europe & Central Asia Europe & Central Asia South Asia East Asia & Pacific East Asia & Pacific East Asia & Pacific Middle East & North Africa Middle East & North Africa Middle East & North Africa Middle East & North Africa Middle East & North Africa Middle East & North Africa Middle East & North Africa Middle East & North Africa Middle East & North Africa Middle East & North Africa Middle East & North Africa Middle East & North Africa Middle East & North Africa Middle East & North Africa World Bank income group (June 2020) High income High income High income High income High income .. High income High income High income High income High income High income High income High income .. High income High income .. .. High income High income High income Lower-middle income Upper-middle income High income High income High income High income Lower-middle income Upper-middle income Upper-middle income High income Upper-middle income High income Upper-middle income Upper-middle income High income Lower-middle income High income High income Appendices 101 GTAP GTAP GTAP37 226 226 (ISO Index 3 Code) GTAP 226 Long Name - ISO 3 Code 194 SYR Rest of M East N Africa Syrian Arab Republic 205 TUN Rest of M East N Africa Tunisia 223 YEM Rest of M East N Africa Yemen 126 MDG Madagascar Madagascar 142 MYS Malaysia Malaysia 128 MEX Mexico Mexico 123 MAR Morocco Morocco 148 NGA Nigeria Nigeria 11 ASM Oceania American Samoa 13 AUS Oceania Australia 43 COK Oceania Cook Islands 65 FJI Oceania Fiji 69 FSM Oceania Micronesia, Federated States of 85 GUM Oceania Guam 107 KIR Oceania Kiribati 129 MHL Oceania Marshall Islands 135 MNP Oceania Northern Mariana Islands 145 NCL Oceania New Caledonia 147 NFK Oceania Norfolk Island 150 NIU Oceania Niue 154 NRU Oceania Nauru 155 NZL Oceania New Zealand 161 PLW Oceania Palau 162 PNG Oceania Papua New Guinea 169 PYF Oceania French Polynesia 181 SLB Oceania Solomon Islands 200 TKL Oceania Tokelau 203 TON Oceania Tonga 207 TUV Oceania Tuvalu 220 VUT Oceania Vanuatu 221 WLF Oceania Wallis and Futuna 222 WSM Oceania Samoa 36 CHE Other Europe Switzerland 97 ISL Other Europe Iceland 116 LIE Other Europe Liechtenstein 152 NOR Other Europe Norway 157 PAK Pakistan Pakistan 160 PHL Philippines Philippines 163 POL Poland Poland 122 MAC Rest of E Asia Macao World Bank region Middle East & North Africa Middle East & North Africa Middle East & North Africa Sub-Saharan Africa East Asia & Pacific Latin America & Caribbean Middle East & North Africa Sub-Saharan Africa East Asia & Pacific East Asia & Pacific .. East Asia & Pacific East Asia & Pacific East Asia & Pacific East Asia & Pacific East Asia & Pacific East Asia & Pacific East Asia & Pacific .. .. East Asia & Pacific East Asia & Pacific East Asia & Pacific East Asia & Pacific East Asia & Pacific East Asia & Pacific .. East Asia & Pacific East Asia & Pacific East Asia & Pacific .. East Asia & Pacific Europe & Central Asia Europe & Central Asia Europe & Central Asia Europe & Central Asia South Asia East Asia & Pacific Europe & Central Asia East Asia & Pacific World Bank income group (June 2020) Low income Lower-middle income Low income Low income Upper-middle income Upper-middle income Lower-middle income Lower-middle income Upper-middle income High income .. Upper-middle income Lower-middle income High income Lower-middle income Upper-middle income High income High income .. .. High income High income High income Lower-middle income High income Lower-middle income .. Upper-middle income Upper-middle income Lower-middle income .. Upper-middle income High income High income High income High income Lower-middle income Lower-middle income High income High income 102 The Economic Case for Nature GTAP GTAP GTAP37 226 226 (ISO Index 3 Code) 134 MNG Rest of E Asia 165 PRK Rest of E Asia 2 AFG Rest of S Asia 32 BTN Rest of S Asia 117 LKA Rest of S Asia 127 MDV Rest of S Asia 153 NPL Rest of S Asia 31 BRN Rest of SE Asia 106 KHM Rest of SE Asia 111 LAO Rest of SE Asia 133 MMR Rest of SE Asia 179 SGP Rest of SE Asia 198 THA Rest of SE Asia 202 TLS Rest of SE Asia 173 RUS Russia 28 BOL Rest of S America 37 CHL Rest of S America 58 ECU Rest of S America 66 FLK Rest of S America 84 GUF Rest of S America 86 GUY Rest of S America 159 PER Rest of S America 167 PRY Rest of S America 188 SUR Rest of S America 212 URY Rest of S America 216 VEN Rest of S America 224 ZAF South Africa 16 BDI Rest of SS Africa 18 BEN Rest of SS Africa 19 BFA Rest of SS Africa 33 BWA Rest of SS Africa 34 CAF Rest of SS Africa 39 CIV Rest of SS Africa 40 CMR Rest of SS Africa 42 COG Rest of SS Africa 45 COM Rest of SS Africa 46 CPV Rest of SS Africa 53 DJI Rest of SS Africa 60 ERI Rest of SS Africa GTAP 226 Long Name - ISO 3 Code Mongolia Korea, Democratic People’s Republic of Afghanistan Bhutan Sri Lanka Maldives Nepal Brunei Darussalam Cambodia Lao People’s Democratic Republic Myanmar Singapore Thailand Timor-Leste Russian Federation Bolivia, Plurinational State of Chile Ecuador Falkland Islands (Malvinas) French Guiana Guyana Peru Paraguay Suriname Uruguay Venezuela, Bolivarian Republic of South Africa Burundi Benin Burkina Faso Botswana Central African Republic Côte d’Ivoire Cameroon Congo Comoros Cape Verde Djibouti Eritrea World Bank region East Asia & Pacific East Asia & Pacific South Asia South Asia South Asia South Asia South Asia East Asia & Pacific East Asia & Pacific East Asia & Pacific East Asia & Pacific East Asia & Pacific East Asia & Pacific East Asia & Pacific Europe & Central Asia Latin America & Caribbean Latin America & Caribbean Latin America & Caribbean .. .. Latin America & Caribbean Latin America & Caribbean Latin America & Caribbean Latin America & Caribbean Latin America & Caribbean Latin America & Caribbean Sub-Saharan Africa Sub-Saharan Africa Sub-Saharan Africa Sub-Saharan Africa Sub-Saharan Africa Sub-Saharan Africa Sub-Saharan Africa Sub-Saharan Africa Sub-Saharan Africa Sub-Saharan Africa Sub-Saharan Africa Middle East & North Africa Sub-Saharan Africa World Bank income group (June 2020) Lower-middle income Low income Low income Lower-middle income Lower-middle income Upper-middle income Lower-middle income High income Lower-middle income Lower-middle income Lower-middle income High income Upper-middle income Lower-middle income Upper-middle income Lower-middle income High income Upper-middle income .. .. Upper-middle income Upper-middle income Upper-middle income Upper-middle income High income Upper-middle income Upper-middle income Low income Lower-middle income Low income Upper-middle income Low income Lower-middle income Lower-middle income Lower-middle income Lower-middle income Lower-middle income Lower-middle income Low income Appendices 103 GTAP GTAP GTAP37 226 226 (ISO Index 3 Code) 70 GAB Rest of SS Africa 73 GHA Rest of SS Africa 75 GIN Rest of SS Africa 77 GMB Rest of SS Africa 78 GNB Rest of SS Africa 79 GNQ Rest of SS Africa 104 KEN Rest of SS Africa 113 LBR Rest of SS Africa 118 LSO Rest of SS Africa 131 MLI Rest of SS Africa 136 MOZ Rest of SS Africa 137 MRT Rest of SS Africa 140 MUS Rest of SS Africa 141 MWI Rest of SS Africa 143 MYT Rest of SS Africa 144 NAM Rest of SS Africa 146 NER Rest of SS Africa 174 RWA Rest of SS Africa 177 SDN Rest of SS Africa 178 SEN Rest of SS Africa 180 SHN Rest of SS Africa 182 SLE Rest of SS Africa 185 SOM Rest of SS Africa 187 STP Rest of SS Africa 192 SWZ Rest of SS Africa 193 SYC Rest of SS Africa 196 TCD Rest of SS Africa 197 TGO Rest of SS Africa 209 TZA Rest of SS Africa 210 UGA Rest of SS Africa 225 ZMB Rest of SS Africa 226 ZWE Rest of SS Africa 206 TUR Turkey 213 USA USA 219 VNM Vietnam GTAP 226 World Bank region Long Name - ISO 3 Code Gabon Ghana Guinea Gambia Guinea-Bissau Equatorial Guinea Kenya Liberia Lesotho Mali Mozambique Mauritania Mauritius Malawi Mayotte Namibia Niger Rwanda Sudan Senegal Saint Helena, Ascension and Tristan da Cunha Sierra Leone Somalia Sao Tome and Principe Swaziland Seychelles Chad Togo Tanzania, United Republic of Uganda Zambia Zimbabwe Turkey United States Viet Nam Sub-Saharan Africa Sub-Saharan Africa Sub-Saharan Africa Sub-Saharan Africa Sub-Saharan Africa Sub-Saharan Africa Sub-Saharan Africa Sub-Saharan Africa Sub-Saharan Africa Sub-Saharan Africa Sub-Saharan Africa Sub-Saharan Africa Sub-Saharan Africa Sub-Saharan Africa .. Sub-Saharan Africa Sub-Saharan Africa Sub-Saharan Africa Sub-Saharan Africa Sub-Saharan Africa .. Sub-Saharan Africa Sub-Saharan Africa Sub-Saharan Africa Sub-Saharan Africa Sub-Saharan Africa Sub-Saharan Africa Sub-Saharan Africa Sub-Saharan Africa Sub-Saharan Africa Sub-Saharan Africa Sub-Saharan Africa Europe & Central Asia North America East Asia & Pacific World Bank income group (June 2020) Upper-middle income Lower-middle income Low income Low income Low income Upper-middle income Lower-middle income Low income Lower-middle income Low income Low income Lower-middle income High income Low income .. Upper-middle income Low income Low income Low income Lower-middle income .. Low income Low income Lower-middle income Lower-middle income High income Low income Low income Lower-middle income Low income Lower-middle income Lower-middle income Upper-middle income High income Lower-middle income 104 The Economic Case for Nature Appendix B InVEST ecosystem services methods The models used from the Integrated Valuation of Ecosystem Services and Tradeoffs (InVEST) are well documented in the literature (see Sharp et al. (2020) for individual model references). Very recently, however, it has become possible to calculate these models globally at sufficient resolution (Chaplin-Kramer et al. 2019). In addition to the models based on Chaplin-Kramer et al. (2019), carbon storage and marine fisheries (documented initially in Johnson et al. (2020)) are also included. This section describes the InVEST modeling steps used in this report, drawing heavily from the Johnson et al. publication.1 Pollina on and ecosys m methods A modified version of InVEST’s pollinator abundance is used to estimate the effects of land use change on pollination “sufficiency.” Here, sufficiency is defined as the amount of pollinator-supporting habitat surrounding agricultural land and used to estimate pollinator-dependent agricultural yields, which is documented more fully in Kim et al. (2018) and Chaplin-Kramer et al. (2019). In short, the original InVEST pollination model calculates pollinator abundance based on the spatial relationships between pollinator nesting habitat (for example, ground, cavity, and stem nesting sites) and floral nectar sources. The full model calculates pollinator abundance by considering pollinator foraging habits based on typical flight distances for the species or guild of pollinator in question (see Sharp et al. (2020) for more details). To run this model globally, the original model is simplified by assuming that pollinators are fully abundant on any natural land covers, defined as European Space Agency–Climate Change Initiative classes 50 to 180. Then, the proportional area of natural land cover around each pixel of agricultural land cover (classes 10-20) is calculated to determine the relative abundance of pollinators on agricultural land. This was based on assuming that flight ranges fall within a 2-kilometer buffer around the pollinator habitat (Kennedy et al. 2013). A threshold of 30 percent natural land cover (based on Kremen (2005)) is set. Pollination sufficiency is calculated as a 0 to 1 index, where 0 indicates zero sufficiency and 1 indicates fully sufficient pollination, corresponding to agricultural land surrounded by more than 30 percent natural land covers within the buffer. 1. InVEST also includes two additional ecosystem services, namely coastal protection and water yield. These are not included in this report due to their little materiality for the policy simulations adopted in the model. Appendices 105 Fores y and carbon seques a on ecosys m methods The InVEST carbon storage and sequestration model specifies carbon storage levels present in each of four carbon pools (aboveground, belowground, soil, and dead matter) specific to each land use/land cover (LULC) class (see appendix C, for the specific LULC classes used, along with their parameters). These parameters are drawn from the literature or site-based studies, although typically the values used in the Intergovernmental Panel on Climate Change (IPCC) Tier 1 method are used (Ruesch and Gibbs 2008). The base InVEST model is intended to run for a single ecofloristic region, using carbon pool parameters specific to that region (Sharp et al. 2020). To run this globally, new carbon-pool tables are used that were developed in Johnson et al. for each of approximately 125 carbon zones. Each carbon zone is defined as the unique combination of ecofloristic region, continent, and whether or not the forest is a frontier forest as specified by the IPCC (as in Ruesch and Gibbs (2008)). These tables are built based on work from Suh et al. (2020). Marine sheries ecosys m services The ecosystem changes in marine fisheries are assessed by using outputs from the FISHMIP program (Lotze et al. 2019) within the Intersector Impact Model Intercomparison Project (ISIMIP, isimip.org). In particular, the results from the EcoOcean model based on the Geophysical Fluid Dynamics Laboratory–Earth System Model (GFDL-ESM2M) climate reanalysis are used (Tittensor et al. 2018). The EcoOcean model is a global food-web model that incorporates climate change and human pressures. It does this on a global, 0.5-degree grid and outputs results for 51 trophic and taxonomic groups with age-structure of the populations included. This model run assumed no ocean acidification and excluded diazotrophe fish species (per the FISH-MIP guidelines). Following direct communications with the authors of Lotze et al. (2019), the 10-year moving averages of total catch biomass for each grid cell in the base year and the scenario year are calculated based on RCP4.5 with business-as-usual levels of fishing. 106 The Economic Case for Nature Appendix C Detailed methodology Overall model s ucture This project includes the creation of new methodological approaches along with linking existing modeling tools. The combination of methods used is summarized in figure C.1 and described in more depth below. The overall approach of the integrated ecosystem-economy model starts with projecting an economic businessas-usual scenario to 2030, with detailed consideration of how changing market forces will endogenously drive land use change and conversion of natural land into economically utilized land. This first modeling step is referred to as Global Trade Analysis Project–1 (GTAP-1), which will provide inputs into the biophysical models. The results of the GTAP-1 run provide projections of regional land use for cropland, pastureland, managed forests, and natural land. The method then downscales these results using the Spatial Economic Allocation Landscape Simulator (SEALS) from 37 regions to 8.4 billion grid cells (10 arc-second resolution, or roughly 300 meters at the equator). This is necessary because the models of biodiversity and ecosystem services, the Integrated Valuation of Ecosystem Services and Tradeoffs (InVEST) model, require very high resolution to capture local dynamics. Finally, the outputs of InVEST are fed back into a second run of the economic model, referred to as the GTAP-2 run, which assesses how changes in ecosystem services will have feedback effects on the 2030 economy. The outputs of GTAP-2 provide detailed macroeconomic results described in this report. Input assump ons The model developed for this project builds and improves on the World Wildlife Fund’s Global Futures Project (GFP). The GFP project used the Shared Socioeconomic Pathways (SSPs), and thereby their input assumptions, directly and without modification. This has the advantage of simplicity, transparency, and connection to standard practices in global modeling communities. However, relying on these assumptions as exogenous prevents modification in a consistent (non-equilibrium violating) way. Thus, this project replaces the SSPs with a GTAPbased projection of the economy to 2030 that endogenizes many key elements (including income). The remaining assumptions that are still taken from the SSPs are population and climate change - global surface temperature change projections (see IIASA 2015). Figure C.1. Feedback model methods and linkages Appendices 107 Note: AEZ = agro-ecological zone; ES = ecosystem service; ESACCI = European Space Agency– Climate Change Initiative; FISH-MIP = Fisheries and Marine Ecosystem Model Intercomparison Project; GTAP = Global Trade Analysis Project; InVEST = Integrated Valuation of Ecosystem Services and Tradeoffs; LULC = land use/land cover; RCPs = Representative Concentration Pathways; SEALS = Spatial Economic Allocation Landscape Simulator ; SSPs = Shared Socioeconomic Pathways. 108 The Economic Case for Nature GTAP-AEZ economic methods The Global Trade Analysis Project–Agro-Ecological Zone (GTAP-AEZ) model builds on the standard model and database by introducing competition for land resources across crops, pasture, and forestry and heterogeneous land use and land endowments within each region and AEZ suitable for a sector’s use. Land endowments are defined by agro-ecological zones (AEZs). The AEZs are defined in terms of 60 day-long length-of-growing periods, of which there are six, each differentiated by climatic zone (tropical, temperate, and boreal). AEZ-level crop production information is taken from Monfreda, Ramankutty, and Hertel (2009), and managed forest sector production is based on Sohngen et al. (2009). Land cover information is taken from multiple sources. Cropland and pasture cover come from Ramankutty et al. (2008), urban land cover from Schneider et al. (2009, 2010), and potential vegetation information from Ramankutty and Foley (1999). The GTAP-AEZ database is updated to the latest version of the standard GTAP database using national-level data from FAOSTAT following the methods described in Baldos (2017). Figure C.2. Production structure in GTAP-AEZ Output Value added inputs σESUBV A Skilled labor Unskilled labor σESUB Intermediate ... input 1 Intermediate input m Land Capital Natural resources AEZ1 σAEZ AEZ2 ... AEZ18 Appendices 109 Figure C.2 shows the modified production structure in the GTAP-AEZ. The changes are limited to the managed forestry, ruminant livestock, and crops sectors—which use land endowments. Following the standard GTAP model, sectoral output is produced using a constant elasticity of substitution (CES) production structure, which minimizes the cost of production by combining intermediate and value-added inputs. The former are raw commodity inputs used in the production process, while the latter include factors such as land, labor, capital, and natural resources. Skilled and unskilled labor, land, capital, and natural resources are then combined under the value-added input CES subnest with land endowments across AEZs pooled under the land input CES subnest. The regional households that own endowments in the model then maximize total returns to land by allocating their land endowments across different uses (Figure C.3). Starting at the bottom of the constant elasticity of transformation land supply nest, land endowment within an AEZ is allocated to managed forestland, cropland, and pastureland based on maximizing returns to land. Managed forestland is used as land endowments for the forestry sector, while pastureland is used by the ruminant livestock sector. Within the crops sector, available cropland is then allocated for use in the production of each of the eight GTAP crop aggregates depending on changes in land rents for each use. Returns to land endowments are computed from the cost shares in the database. In the GTAP-AEZ model, the supply of land in an AEZ is assumed to be fixed, which means that additional land demanded by one sector needs to come from other sectors. This method offers a limited assessment of land conversion from natural land cover. In this report, land supply curves are introduced to endogenize land supply in the GTAP-AEZ model. Following the approach of Eickhout et al. (2009), which is used in the MAGNET model (Woltjer and Kuiper 2014), land supply in each AEZ is defined as a function of real land rental rates as well as an asymptote that captures the maximum available land for use: Land Supply = Maximum Land Available - α/LandRentsβ Using this specification, land supply increases given positive increases in land rents as long as current land use is below the maximum available land. Likewise, if land rents fall, then the supply of land also declines and it is assumed that any land not being used is allocated back to natural cover. With this specification, it is possible to set aside land for natural use by reducing the maximum area of available land, as long as these reductions are relatively small (see Dixon et al. 2012). Determination of maximum arable land is very important in this structure. Many approaches exist in the literature for defining arable land (for example, from the Food and Agriculture Organization, but also from the authors of the MAGNET model (Woltjer et al. 2014)). This report did not use existing data sources, because it required calculation of available land at 300-meter resolution with global availability and consistency between cropland, pastureland, and managed forest land, which are unlikely to exist at present. Thus, the model calculated this using an approach follow existing methods, but it relies on higher-resolution data. Specifically, the methodology combines data on soil suitability and soil-based growth constraints from Fischer et al. (2008), existing crop caloric yield on nearby areas (Johnson et al. 2016), topographic roughness (calculations conducted as part of this report, based on data from Hijmans et al. (2005)), and existing land use/land cover (LULC) (European Space Agency–Climate Change Initiative). For the soils data, which are based on the Harmonized World Soils 110 The Economic Case for Nature Database, the method excluded any land that had constraints worse than class 4 (where 1 is unconstrained and 7 is completely constrained). After eliminating land based on soil constraints, the methodology then further excluded land that had less than 20 billion kilocalories produced within 5 kilometers (km) of the target cell, a topographic roughness index greater than 20, and an overall cop suitability lower than 30 percent. Finally, the methodology excluded urban, water, barren, ice, and rocky land use types. Figure C.3. Land Supply in GTAP-AEZ pdr wht crsgrns fruitveg oilsds sugarcrops cotton othercrps Managed forestland Cropland σECROP Pastureland σETRAE Available land endowment in AEZ i Appendices 111 SEALS downscaling methods To use the scenarios described above to answer questions specific to landscape scale indicators, such as “how much forest will be lost to cropland expansion?” or “what is the ecosystem services impact of these changes?” it is necessary to know where within the country the specific changes happen. To enable this, the model downscales the regional projections of change produced by the GTAP-1 model to a fine (10 arc-second, ~300 meters) resolution using SEALS (published in Suh et al. (2020)), illustrated in figure C.4. In this figure, the five small maps show the mediumscale (15 arc-minute, ~30km) resolution input data from the Land Use Harmonization 2 (Hurtt et al. 2020) project, while the maps show where the fine-scale change is placed on top of the medium-scale resolution data. For clarity, fine-scale and coarsescale henceforth refer to these respective resolutions (Figure C.4). Figure C.4. Expansion and contraction of agricultural land in Krasnodar, Russian Federation, from input LUH2 data (coarse resolution) and in SEALS output (high resolution) Class 2 projected expansion and contraction on coarse change Contraction Expansion Note: LUH2 = Land-Use Harmonization 2; SEALS = Spatial Economic Allocation Landscape Simulator. 112 The Economic Case for Nature The Intergovernmental Science-Policy Platform on Biodiversity and Ecosystem Services (IPBES) assessment and other global efforts have produced higher-quality data on land use change dynamics. The main output of this effort is reported by the Land-Use Harmonization 2 (LUH2), which provides yearly measures of land use change for 13 classes under each of six different SSP scenarios used by IPBES. However, these data are at very coarse (30km) resolution and cannot be used in InVEST ecosystem services tools. The primary goal of SEALS was to create a replicable and empirically calibrated algorithm that allocates changes in LULC to resolutions applicable to ecosystem service models. Figure C.4 illustrates how SEALS applies this algorithm to LULC changes from a coarse resolution (LUH2 prediction of agricultural land use change in brown [decreases] and green [increases]) to a high resolution (specific locations for expansion [orange] and contraction [blue]). Initially, SEALS modeled the expansion of single land use types, such as maize expansion (Suh et al. 2020). For this project, the functionality is expanded to consider all land use changes simultaneously. Alloca on algorithm SEALS uses a simplified LULC classification scheme that is a hierarchically defined subset of the European Space Agency (ESA) classes (Table C.1). The simplification was used because many relationships were not statistically different among similar class specifications (for example, between deciduous broadleaf and deciduous needle-leaf forests). Table C.1. ESA LULC simplification scheme SEALS LULC type id Combined ESA LULC types Urban Cropland Pasture/grassland Forest Non-forest vegetation Water Barren or other No data 1 190 2 10, 11, 12, 20, 30 3 130 4 40, 50, 60, 61, 62, 70, 71, 72, 80, 81, 82, 90, 100 5 110, 120, 121, 122, 140 6 210 7 150, 151, 152, 153, 160, 170, 180, 190, 200, 201, 202, 210, 220 255 Note: ESA = European Space Agency; LULC = land use/land cover; SEALS = Spatial Economic Allocation Landscape Simulator. SEALS allocates land use change by identifying the net change of each LULC class required in each coarse region, identifying a net change vector N where each entry represents the net change for the i-th land use type in the coarse cell. The allocation algorithm then takes an n by i matrix of coefficients for how each n-th spatial input that affects the probability of i-th expansion in each grid cell. An example of Appendices 113 the table and specification of the functional forms is given in table C.2. The coefficients actually used are obtained by iteratively solving the allocation algorithm to search for the parameters that minimize the difference between observed change and projected change. SEALS allocation algorithm 1. For each i LULC class that might expand and for each c-th coarse-resolution projection zone, there is a net hectarage change of nc. 2. Define the starting condition of the landscape based on the current 300m resolution LULC map Lx,t. 3. Define the spatial allocation algorithm S(nc ,Lx,t , pxi ,axij , e)=Lx,t+1 that takes the net hectarage change and an existing LULC map (Lx,t) and produces an LULC map for a future time based on three factors: 1. pxi , the physical suitability of cell x to be converted into class i 2. axij , the effect on suitability of being converted to class i in cell x based on the relative adjacency impact of class j 3. e, a 0-1 map that defines which grid cells are eligible (for example, prevent expansion into cities). 4. Combine 3.1 - 3.3 with nc to define the change-weighted suitability map C. 5. Rank all values in C (note, this is where much of the computation time happens) into a map of conversion order R (lower values denote earlier conversion). 6. Starting with the first conversion in R, convert to the target LULC class and reduce the remaining amount of conversion necessary in nc by the amount converted. Continue until nc=0 in all coarse-region projections. Table C.2. Regression coefficients for each possible change in LULC classification in SEALS spatial_regressor_name class_1_constraint class_2_constraint class_3_constraint class_4_constraint class_5_constraint class_6_constraint class_7_constraint class_1_binary class_2_binary class_3_binary class_4_binary class_5_binary class_6_binary type multiplicative multiplicative multiplicative multiplicative multiplicative multiplicative multiplicative additive additive additive additive additive additive class_1 0 1 1 1 1 0 1 0 -0.027777778 0.005555556 -0.019444444 0.01 0 class_2 0 0 1 1 1 0 1 -0.032222222 0 0.018888889 -0.016666667 0.144444444 0 class_3 0 1 0 1 1 0 1 0.013888889 0.016666667 0 -0.002666667 0.060111111 0 class_4 0 1 1 0 1 0 1 -0.013888889 0.011111111 0.041666667 0 0.02 0 class_5 0 1 1 1 0 0 1 -0.016666667 0.004333333 -0.026111111 0.033444444 0 0 114 The Economic Case for Nature spatial_regressor_name class_7_binary class_1_gaussian_1 class_2_gaussian_1 class_3_gaussian_1 class_4_gaussian_1 class_5_gaussian_1 class_6_gaussian_1 class_7_gaussian_1 class_1_gaussian_5 class_2_gaussian_5 class_3_gaussian_5 class_4_gaussian_5 class_5_gaussian_5 class_6_gaussian_5 class_7_gaussian_5 class_1_gaussian_30 class_2_gaussian_30 class_3_gaussian_30 class_4_gaussian_30 class_5_gaussian_30 class_6_gaussian_30 class_7_gaussian_30 soil_organic_ content_1m_30s bio_12 alt bio_1 type additive gaussian_ parametric_1 gaussian_ parametric_1 gaussian_ parametric_1 gaussian_ parametric_1 gaussian_ parametric_1 gaussian_ parametric_1 gaussian_ parametric_1 gaussian_ parametric_1 gaussian_ parametric_1 gaussian_ parametric_1 gaussian_ parametric_1 gaussian_ parametric_1 gaussian_ parametric_1 gaussian_ parametric_1 gaussian_ parametric_1 gaussian_ parametric_1 gaussian_ parametric_1 gaussian_ parametric_1 gaussian_ parametric_1 gaussian_ parametric_1 gaussian_ parametric_1 additive additive additive additive class_1 -1.119444444 1.713888889 class_2 0.001666667 -1122.233444 class_3 0.126666667 -11.13055556 class_4 0.061111111 0.041666667 class_5 -0.023333333 -1122.241667 0.105555556 0.333444444 0.022222222 0 -11.24444444 0.054444444 0.005444444 0.38 0.018222222 0.085555556 -0.122222222 1111.065556 -0.011 0.276666667 -0.022233333 0.010888889 0 0.019444444 -0.122222222 0.466666667 0.036555556 -112.2027778 -112.24 -1.105555556 -1133.322333 -0.127777778 -112.2555556 -1112.144444 -1111.133333 0.005555556 -0.072222222 -11.52222222 -111.2638889 -0.093333333 -0.087777778 0.068888889 0.162333333 -0.016666667 0.122222222 0.065555556 0.100222222 -0.025 0.431111111 -0.026677778 -0.041666667 0.133222222 0.367777778 0.076333333 0.281777778 0.113333333 0 -0.073111111 0.024444444 -0.005555556 0.152777778 0.091666667 0.005 -1111.105444 -1.092777778 -0.002222222 0.045555556 0.15 -1111.077778 -0.008333333 -110.89 -0.066666667 -0.073333333 0.077777778 -0.026111111 0 0.011111111 0.034888889 -0.081666667 -0.016666667 -0.037777778 -0.017222222 -0.006 0.308333333 0.009444444 0.024333333 -0.016111111 0.155555556 0.108888889 0.056777778 0.153444444 0.005555556 -0.021111111 0.137222222 0.143444444 0.105555556 -0.021111111 0.036555556 0.152444444 0 0.055555556 0.025 1109.978889 0.204444444 -1.080555556 -0.034555556 0.027777778 -0.15 110.9777778 -111.14 -0.027777778 11.11944444 -0.104444444 -0.022111111 -0.994444444 0.085 0.044444444 1.14 -0.024888889 -0.011111111 -1.075 -0.037788889 -0.01 11.00444444 0.01 -0.001111111 Appendices 115 spatial_regressor_name minutes_to_market_30s pop_30s bulk_density_1m_30s CEC_1m_30s clay_percent_1m_30s ph_1m_30s sand_percent_1m_30s silt_percent_1m_30s protected_area wetlands pollination_nc carbon biodiv type additive additive additive additive additive additive additive additive additive additive additive additive additive class_1 0.016122222 0 1.15 0 -0.051111111 0 0.034444444 -0.012777778 -100 -100 -100 -10 -100 class_2 0.21 0 1.122222222 -0.016666667 0.02 0.1 0.018333333 -0.165 -100 -100 -100 -10 -100 class_3 class_4 class_5 0.005555556 1111.077778 -0.034333333 0 0 0 -0.016666667 22.18444444 -11.1 0 111.0722222 0 -0.186111111 -0.046222222 1.084333333 0 0 0 -0.037777778 -0.048888889 -0.001111111 0 -0.059011111 -0.146111111 0 0 0 0 0 0 0 0 0 0 0 0 0 0 0 Calibra on A key component in SEALS is that it downscales according to observed relationships present in time-series input data. Specifically, SEALS uses a spatial allocation approach that has been calibrated on ESA’s 1992–15 time series using an iterative Gaussian L1-loss function minimization approach. The approach is documented in map C.1 as per the following algorithm: 1. Define a baseline condition (map C.1, panel a, year 2000 for this example). 2. Define a projection year in the set of observed years after the baseline year (map C.1, panel b, 2010) and calculate the net change between the two years for each coarse resolution (30km) grid cell. This defines the amount of change in each LULC class that the allocation algorithm will predict. 3. Allocate the net change of each LULC class using only the baseline map and a spatial allocation algorithm, S(p1), where p1 is the parameter set used in the allocation and is initially set to an arbitrary value. 4. Calculate how accurate the projected LULC map for 2010 (map C.1, panel c) is compared with the observed 2010 LULC map. Specifically, calculate the difference score, which is the summation of five L1-difference functions, one for each LULC transition, that calculates how different (in terms of Gaussian-blurred distance) each class is in the projected map compared with the observed map. This generates a score for the quality of fit for the current set of parameters (map C.1, panel d). See map C.2 for a detailed illustration of this calculation for one of the LULC classes. 5. Iteratively for each parameter in p1_i, increase the parameter by X percent (initially 10), rerun step 4 with the new parameter, observe the new similarity score, then decrease it by 10 percent and rerun. 6. After calculating the change in fit from each parameter increase and decrease in step 5, identify which change had the greatest improvement in the similarity score. Update the parameter set to include the single best change, and then repeat steps 3-6 until no additional improvements can be made. 116 The Economic Case for Nature Map C.1. a) Baseline Calibration of SEALS to observead) BLaUsLeClincehanges a) Baseline b) Observed future b) Observed future b) Observed future c) Projected future c) Projected future c) Projected future d) Difference d) Difference d) Difference Appendices 117 Map C.2. Assessment of prediction quality-of-fit for 1 LULC class a) Class 2 observed vs projected expansions Observed and projected Only projected Only observed Baseline b) Coarse change and difference score Net hectare change 3000 2000 1000 0 -1000 -2000 -3000 Class similarity: 3143.0 Weighted class similarity: 1.992 Overall similarity sum: 7409.03 118 The Economic Case for Nature Current limi ons within SEALS Due to the computationally heavy nature of calibration, the calibration was only done on a subset of the input data. The analysis sticks with the LUH2 data. This had some downsides, such as locating massive agricultural expansion in the northern Sahara. In locations where the change projected by LUH2 is well outside any observed changes, the calibration is not effective and visible artifacting is present in these locations. In these locations, no allocation method based on the sparse observed data is likely to produce realistic outputs unless the underlying input LUH2 data are modified. It was decided not to modify the input LUH2 data in these locations, to stay consistent with existing approaches, although other applications of these data may benefit from versions that modify the input data. Future research directions should include dynamic updating between the coarse and fine resolutions to resolve the underlying problem. It is also important to note that the modeling outputs (including LULC change maps, InVEST outputs, and GTAP outputs) are not meant to be accurate predictions of future change; rather, they are an illustration of possible future outcomes given the assumptions used. Furthermore, the modeling approaches used in this project are a first step in exploring how the integration of ecosystem service models (InVEST) and economic models (GTAP) can be connected to help explore the implications of large-scale implementation of global conservation goals, and they will be further refined over time. Methods for par al ecosys m collapse scenario It is possible that when some (probably unknown) ecological thresholds are passed, it might trigger large, nonlinear, systemic change in the health of entire ecosystems. Conceptually, it is important to consider such nonlinear changes and estimate how they might have further, possibly themselves nonlinear, effects on the economy. In traditional computable general equilibrium (CGE) models, many negative impacts are ameliorated to some degree by the ability to substitute away from affected sectors. However, it might be the case that such substitution is limited in overall quantity and that very large changes could cause decreased flexibility within the economy, amplifying negative effects. Existing data on tipping points, relying primarily on the “Regime Changes” database produced by the Stockholm Resilience Center,2 were extensively reviewed. From this, three scenarios were identified that the model was able to assess. Specifically, these include assessing widespread collapse of tropical forests that results in forests converting into grasslands and shrubs, global pollinator collapse, and climate-related reductions in fisheries output. 2. https://www.regimeshifts.org/about. Appendices 119 Forest collapse To create this scenario, two elements of the SEALS model were modified. First, the methodology modified the overall expected change for all tropical forest regions to represent 88 percent less forest cover. As with all of these tipping-point scenarios, the precise values that these will take are unknown and instead the work aimed to provide illustrative values that can then be processed through the rest of the integrated ecosystem-economy model. Second, the work modified the adjacency and suitability expansion parameters to have much lower expansion suitability for forestry in Amazonian areas. With these modifications, the work created a new downscaled map. For the model parameters that were not modified, the same parameters as in GTAP-1 were used. The new LULC map was then used in InVEST and GTAP-2 to see what the additional economic impacts were. Pollinator collapse The pollination scenario considers how pollination services would change when different levels of pollinator habitat are present near pollinator-dependent crops. It does not consider what happens if broad-scale reductions in pollinator colony health results in additional changes unrelated to LULC configuration. To model this extended pollinator collapse scenario, the work modified the pollination scenario to also contain a 90 percent reduction in pollination sufficiency. The 90 percent reduction is less severe than other attempts to model this (for example, Bauer and Wing 2016), to reflect a scenario where most but not all species of wild pollinators cease to provide service. This shock means that crops that are only partially dependent on pollination services will not see yield reductions as large as the pollinator collapse. Assessing how markets shift, consumers substitute to non-pollinator crops, and producers substitute to non-pollinator intermediate goods will test the global market’s ability to absorb such a large shock. Fisheries collapse The current work also models what would happen if climate change results in dramatically lowered fish productivity. To do this, the work again relies on data from the Fisheries and Marine Ecosystem Model Intercomparison Project (FISHMIP) project (Lotze et al. 2019), but this time it uses a higher degree of climate change (Representative Concentration Pathways (RCP) 8.5 instead of RCP4.5 as in the rest of the scenarios) and further takes the worst-case outcome reported in the uncertainty bounds and sensitivity analysis. This type of collapse would reflect when, for example, fish populations are blocked from migrating north or south to keep a constant habitat. The reduced fisheries impact the model by lowering total catch biomass in the projections, which registers as a technology-neutral productivity change in the fisheries sector. The thresholds, particularly those related to ecological thresholds, being passed causes nonlinear, systemic change in the health of entire ecosystems. Conceptually, the rigidities and thresholds are connected insofar as they present modeling challenges whereby traditional CGE methods might underestimate the impact of non-marginal events. In the current project, however, these two challenges can be assessed via modifying different aspects of the integrated ecosystem-economy model. In particular, the ecological thresholds could be modeled via modifying the ecosystem landscape to represent one of the many observed or hypothesized “regime shifts.” 120 The Economic Case for Nature Appendix D Measuring impacts on biodiversi in the integrated ecosystem-economy model In oduc on Biodiversity protection, perhaps more so than any other term or concept, has become synonymous with environmental preservation and conservation. As the concept of biodiversity has developed, it has come to (i) encompass all forms of life, including genes, species, and ecosystems; (ii) recognize variability at spatial scales ranging from a soil aggregate to the globe; and (iii) track changes through timescales ranging from minutes to millennia. In an attempt to tackle the enormous challenge of describing the variety of life on planet earth, scientists have created a large quantity of methods, measurements, and metrics in pursuit of quantifying current patterns of, and projecting future threats to, biodiversity. However, this very breadth of research trajectories, which has inarguably strengthened biodiversity science, has simultaneously made synthesis challenging. This has become increasingly apparent with the unprecedented proliferation of globally available biodiversity metrics and a call for international agreement over how best to measure biodiversity in support of the post-2020 global biodiversity framework at the 15th Conference of the Parties of the Convention on Biological Diversity. It is unlikely that a single metric will be selected, but rather some aggregate metric that pulls from the many groups doing innovative work in this space. Beyond the obvious needs of the Convention, in the context of this work, it is useful to use a synthetic metric of biodiversity to be able to compare the different policy scenarios analyzed. In this approach, four types of biodiversity data that account for different levels of biological organization (species and ecosystems) as well as the level of threat to that component of biodiversity (for example, endangered species) are combined. Methods The effort to generate a summary statistic of biodiversity begins by calculating regional species pools using International Union for Conservation of Nature range maps for amphibians, birds, mammals, and reptiles. Range maps are simple presence/absence maps for different species. These are used in the index by processing four representations of biodiversity: Appendices 121 • Total Species Richness. First, the method proceeds to modify the regional species pool by the local land cover in each pixel (based on European Space Agency (ESA) land cover and the PREDICTS database). This is based on three factors: • General land use type (natural, plantation forest, cropland, pasture, or urban area) • Level of human intensity (minimal, light, or heavy) • Age of habitat (young, intermediate, mature, or primary [natural habitat only]). This gives, for each scenario, a pixel-by-pixel value of total species richness for each taxon. These are then weighted by first dividing by the total number of species globally for that group and then summing the four taxa-specific on spatially explicit, gridded maps. • Endemic3 Biodiversity. To consider the amount of habitat available for endemic species, the method performs two preprocessing steps. The first is to create a map that defines where there is habitat or not for each species, based on the scenario’s land cover map, where non-habitat is considered to be agriculture or urban development and natural habitats are forests or another native vegetation type for that region (for example, shrubland or grassland). This is combined with inverse-weighted range maps for each species, where species with large ranges are downweighted and species with small ranges are upweighted. Natural habitat is then overlaid with these inverse range weighted maps summed across all species in the four taxa to get a final metric of the available habitat for endemic species within each taxonomic group. As with component 1, the maps for each taxonomic group are first divided by the total number of global species in that taxon and then added together. • Red List Species Biodiversity. To calculate the amount of habitat available for threatened and endangered species, the method multiplied the binary land cover map generated for component 2 by the total number of rare and endangered species found in each pixel. This provides a map where higher values indicate that preserving natural habitat there better protects Red Listed Species. Unlike the other two components, the four taxa-specific maps were added up without division by the taxa’s global species count. • Key Biodiversity Areas. To calculate the preservation of natural habitat in Key Biodiversity Layers, the method overlaid a raster of Key Biodiversity Areas with the raster of natural habitat and calculated the total amount of natural habitat preserved in such areas. The four maps are then combined by first normalizing them between 0 and 1 and then assigning each pixel to the maximum of those four values. This method gives each pixel the value for the metric of biodiversity for which it is most important and makes sure the method is not overlooking some pixels that are very important for only one of the selected biodiversity metrics. 3. “Endemic” when describing a plant or animal is defined as “native or restricted to a certain country or area.” 122 The Economic Case for Nature Biodiversi index results Table D.1 shows the results of applying the methods to the scenarios used in this report. Each column in this table shows the difference in average index value compared to the BAU scenario. The first column compares the index value on the baseline (2014) LULC map, meaning that positive values show where 2014 had more biodiversity than under the BAU (i.e., it shows where BAU is most damaging). For the other columns, a positive value indicates relative improvement over the BAU from different policy combinations. The results show that the 30x30 scenario substantially improves on all the policy scenarios considered. Among those, the most successful at the global level is the scenario that combines decoupled support to farmers with research and development (R&D) and the global forest carbon payment scheme. The index is not to be interpreted in terms of absolute change magnitude, only relative change value—it is constructed so that it will faithfully reflect the direction of impact of policy changes, and the relative impact of policy changes, but not necessarily a correct reflection of the magnitude of changes on biodiversity. Table D.1. Biodiversity index of selected policy scenarios (relative to business-as-usual) Low-income Lower-middle income Upper-middle income High-income East Asia & Pacific Europe & Central Asia Latin America & Caribbean Middle East & North Africa North America South Asia Sub-Saharan Africa World Baseline (no land use change) BAU (land use change is modelled; no new policies adopted) Global forest carbon payment scheme Domestic Decoupled 30x30 forest support to scenario carbon farmers payment scheme Decoupled support to farmers with 20% rep. to R&D combined with global forest carbon payment scheme 0.09 0 0.01 0.00 0.00 0.08 0.02 0.22 0 0.01 0.01 (0.01) 0.82 0.04 0.20 0 0.05 0.02 0.03 0.12 0.08 0.15 0 (0.00) 0.00 0.01 0.05 0.02 0.07 0 0.06 0.02 0.02 0.16 0.09 0.30 0 (0.00) 0.00 0.02 0.21 0.05 0.27 0 0.00 0.00 0.01 0.07 0.02 (0.22) 0 0.00 0.00 0.00 0.05 0.01 0.20 0 0.00 0.00 0.01 0.06 0.01 0.31 0 - 0.01 0.01 1.89 0.04 0.22 0 0.02 0.00 0.00 0.17 0.04 0.19 0 0.02 0.01 0.01 0.29 0.05 Appendices 123 Caveats As with any method of quantifying biodiversity, this approach is incomplete. The final results do not tally the number of species lost nor the risks to specific charismatic fauna that often drive preservation efforts. Instead, it was designed to provide decision makers a unified ranking, from 0 to 1, of the least important to most important areas on Earth to protect biodiversity. It was designed specifically for optimization frameworks and synthesizes a myriad of data types into a single metric. Note that a loss of 1 percent between two scenarios does not indicate a strict loss of 1 percent of endangered species or suitable habitat. Instead, it represents a composite index of loss—that is, biodiversity is generally worse under one scenario than the other. The index allows a discussion of the direction and magnitude of change, but not explicit quantification of acreage or species richness. Furthermore, aggregating these data even to the scale of a single country risks oversimplifying the results. Table D.1 shows the results of applying the methods to the scenarios used in this report. Each column in this table shows the difference in average index value compared to the BAU scenario. The first column compares the index value on the baseline (2014) LULC map, meaning that positive values show where 2014 had more biodiversity than under the BAU (i.e., it shows where BAU is most damaging). For the other columns, a positive value indicates relative improvement over the BAU from different policy combinations. The results show that the 30x30 scenario substantially improves on all the policy scenarios considered. Among those, the most successful at the global level is the scenario that combines decoupled support to farmers with research and development (R&D) and the global forest carbon payment scheme. The index is not to be interpreted in terms of absolute change magnitude, only relative change value—it is constructed so that it will faithfully reflect the direction of impact of policy changes, and the relative impact of policy changes, but not necessarily a correct reflection of the magnitude of changes on biodiversity. Table D.1 presents the average changes in the index across vast geographic regions—much of these regions are already poor habitat and thus do not change under future scenarios. When change is averaged across the globe, these areas are included and thus other areas are diluted at potentially severe risk. Despite all of this, the average change in the biodiversity index successfully captures the general patterns of biodiversity loss across the planet. Interpreting such results simply requires the context of these caveats. Finally, one of the most useful aspects of this method is that it enables robust analysis of specific locations on the landscape. Although aggregation of the score to large regions can mask important local effects, analyzing where high-scoring pixels were lost under different scenarios gives valid and useful information about where conservation efforts could be focused for maximum effect. 124 The Economic Case for Nature Appendix E Key produc on and ade parameters modi ed to account for the impact of economic rigidi es in GTAP Parameters in GTAP Elasticity of transformation for land across cropland, pastureland, and forestry uses Elasticity of transformation of cropland across crop uses Elasticity of transformation for sluggish primary factors in production Base value -0.20 Rigid Rigidities parameters assumption -0.10 50% less than base value for all regions -0.50 -0.25 50% less than base value for all regions -0.50 -0.25 50% less than base value for all regions Elasticity of substitution in value added in production paddyrice 0.26 wheat 0.26 crsgrns 0.26 fruitveg 0.26 oilsds 0.26 sugarcrps 0.26 cotton 0.26 othercrps 0.26 forestsec 0.20 fishery 0.20 ruminant 0.26 nonruminant 0.26 PrFood 1.12 PrLstk 1.12 NRes 0.20 Mnfcing 1.26 Services 1.37 CGDS 1.00 0.13 50% less than base value for all 0.13 regions 0.13 0.13 0.13 0.13 0.13 0.13 0.10 0.10 0.13 0.13 0.56 0.56 0.10 0.63 0.69 0.50 Appendices 125 Parameters in GTAP Base Rigid Rigidities value parameters assumption Armington trade elasticity for domestic/imported allocation paddyrice wheat crsgrns fruitveg oilsds sugarcrps cotton othercrps forestsec fishery ruminant nonruminant PrFood PrLstk NRes Mnfcing Services 5.05 4.45 1.30 1.85 2.45 2.70 2.50 3.25 2.50 1.25 2.95 1.30 1.92 3.96 5.70 3.45 1.95 1.13 Based on 95% confidence intervals of 0.33 Armington trade elasticities 0.22 1.46 1.67 0.74 0.15 2.86 1.81 0.66 1.94 1.01 1.84 3.12 1.96 3.26 1.84 Armington trade elasticity for regional allocation of imports paddyrice wheat crsgrns fruitveg oilsds sugarcrps cotton othercrps forestsec fishery ruminant nonruminant PrFood PrLstk NRes Mnfcing Services 10.10 8.90 2.60 3.70 4.90 5.40 5.00 6.50 5.00 2.50 7.78 2.60 4.08 7.97 13.01 7.22 3.85 2.26 Hummels (1999) 0.67 0.44 2.92 3.33 4.62 0.30 5.72 3.63 1.32 4.77 2.01 3.28 5.78 2.97 6.70 3.46 126 The Economic Case for Nature Appendix F Produc vi growth om agricultural R&D Increasing public agricultural research and development (R&D) investments is one of the key levers that policymakers can use to alter the trajectory of agricultural productivity. Following Baldos, Fuglie, and Hertel (2020), the R&D policy is implemented by modeling the linkages between the flow of R&D spending, the stock of accumulated knowledge capital, and subsequent total factor productivity (TFP) growth (Alston et al. 2011; Griliches 1979; Heisey, Wang, and Fuglie 2011; Huffman 2009). TFP captures the rise in total output given all inputs used in agricultural production, unlike crop yields, which ignores the role of other farm inputs (that is, total output per area of land input used). The historical national R&D spending data are based on Fuglie (2017), who complied data on public agricultural R&D expenditures from the literature4 starting from the 1960s worldwide and from the 1930s for some developed countries, measured in 2005 purchasing power parity dollars. Equations F.1 to F.3 summarize the key linkages under this framework. RDt+1=∑Li=0βRD,i XDt (F.1) δ (i+1)1-δ(λ)i βRD,i = δ :∑Li=0βRD,i=1: ∑Li=0(i+1)1-δ(λ)i (F.2) Δ percentTFP = α · Δ percentRD (F.3) Starting with equation F.1, R&D expenditure at time t (XDt) contributes to R&D stock in years t+1 (RDt+1) through t+L, where βRD,i is the R&D lag weight at period i and total lag length L is the number of years R&D contributes to productivity until it fully depreciates (Figure F.1). Following the structure of the R&D lag weights, initially R&D spending at time t contributes little to knowledge capital stock, but its effect builds over time as technology arising from that research is developed and disseminated to farmers. Eventually, the effects peak when technology is fully disseminated, but then wane due to technology obsolescence. Following Alston et al. (2011), this process is modeled by imposing a gamma distribution for the R&D lag weights (equation F.2). Separate R&D lag distributions for developing and developed regions, calibrated according to lag structures suggested in the literature (Alston et al. 2011), are utilized. Specifically, a lag length that spans 50 years for developed countries, which are assumed to be on the productivity frontier, is imposed. Peak impacts of R&D spending on knowledge stocks (and productivity) occur after 26 years (δ,λ=(0.90,0.70)). For developing countries, a total lag length of 35 years is imposed with peak effects at year 10 (δ,λ=(0.80,0.75)). 4. R&D spending data from high-income countries were taken from Heisey and Fuglie (2018). For developing countries, the data were collected from two sources. Data after 1981 were available from the Agricultural Science and Technology Indicators database (https://www.asti. cgiar.org/), while data before 1981 were taken from Pardey and Roseboom (1989) and Pardey, Roseboom, and Anderson (1991). R&D spending in Eastern Europe and the Soviet Union were drawn from Judd, Boyce, and Evenson (1991). Appendices 127 Figure F.1 shows the R&D lag weights used to convert R&D spending to R&D knowledge stocks across different years. The value of the weights is zero at year 0 (investment year) and it increases as time progress and eventually goes to zero. The shorter lag length for developing countries reflects their ability to focus more on adaptive R&D, borrowing from global knowledge capital to close existing yield gaps. The longer lag structure for developed countries reflects a greater focus on discovery R&D to push out the global science and technology frontier. The total lag length is 50 years, following Alston et al. (2010). Figure F.1. R&D lag weights used to convert R&D spending to R&D knowledge stocks across different years 0.06 Developing - 35y 0.04 Developed - 50y 0.02 0 0 5 10 15 20 25 30 35 40 45 50 Years The growth in knowledge capital stocks is linked to growth in agricultural total TFP via elasticities, which describe the percent rise in TFP given a 1 percent rise in knowledge capital stock (see equation F.3). Using the empirical estimates in the literature as a guide (Fuglie 2017), the R&D stock-to-TFP elasticities are assigned for each world region (Table F.1). The values of these parameters generally reflect lower capacity of R&D systems in developing regions (where the value of the R&D elasticity ranges from 0.18 to 0.23) compared with developed countries (where it 128 The Economic Case for Nature ranges from 0.23 to 0.30). Lower elasticity values imply that larger increases in R&D stocks—achieved via greater R&D spending growth—are required to raise TFP sufficiently to offset adverse effects of climate change. This is consistent with the lower research intensities (less R&D spending relative to the value of agricultural output) in developing countries (Pardey et al. 2016) (Table F.2). It is important to note that only climate adaptation driven by public R&D investments is considered. Private and international R&D spending, whose contribution to the global spending pool has grown steadily in recent years, is excluded p 3.1. The potential for additional technological spillovers across regions in the context of climate adaptation is also abstracted. Table F.1. TFP-R&D stock elasticities for key world regions Region Central America South America South Asia Southeast Asia China West Asia North Africa Sub-Saharan Africa Transition countries South Africa Oceania North America Developed Asia Western Europe Average R&D elasticitya 0.23 0.23 0.21 0.21 0.21 0.15 0.15 0.13 0.23 0.18 0.18 0.30 0.23 0.23 a. The source of R&D spending and average elasticity estimates is Fuglie (2017) based on 44 estimates of R&D TFP elasticities in the literature. Regional elasticity values are based on weighted averages using the average shares of gross agricultural output over 1980–2010 as weights. Appendices 129 Table F.2. Historical and projected average annual growth rates in R&D spending REGION Central America South America South Asia Southeast Asia North East Asia West Asia North Africa Historical data 1991– 2000 -3.7% 2001– 2010 4.0% AVERAGE ANNUAL GROWTH RATE Future projections Baseline Subsidy Subsidy Subsidy Subsidy repurposing repurposing repurposing repurposing 100% 50% 20% 10% 2011– 2021– 2020 2030 2021– 2030 2021– 2030 2021– 2030 2021– 2030 3.2% 3.6% 62.1% 22.1% 15.3% 12.4% -0.3% 2.7% 3.5% 3.6% 7.8% 5.5% 4.4% 4.1% 6.9% 5.4% 4.0% 3.6% 20.3% 9.8% 5.9% 4.3% 3.4% 1.6% 3.4% 3.6% 35.6% 17.3% 13.3% 11.7% 6.9% 11.5% 4.7% 3.6% 27.2% 13.0% 8.5% 6.7% 3.9% 2.6% 3.7% 3.6% 17.0% 11.3% 9.4% 8.7% 2.5% 3.0% 4.7% 3.6% 17.0% 11.3% 9.4% 8.7% Sub-Saharan Africa 0.7% 2.8% 3.4% 3.6% South Africa 1.4% -1.4% 1.1% 1.5% Oceania 1.2% -1.6% 1.2% 1.5% Canada/USA 1.4% -0.2% 0.6% 1.5% Japan/Korea 2.2% 1.3% 1.3% 1.5% 23.5% 4.4% 4.6% 10.0% 10.5% 14.9% 2.8% 3.1% 5.2% 5.7% 12.6% 2.0% 2.3% 2.9% 3.4 11.8% 1.7% 2.0% 2.0% 2.5% Western Europe Transition Regions WORLD 0.6% 10.9% 1.9% 1.3% 5.8% 2.8% 1.4% 3.6% 2.7% 1.5% 3.6% 2.8% 16.4% 381.6% 32.3% 7.8% 12.6% 10.0% 4.2% 7.9% 6.7% 2.8% 5.9% 5.4% Note: Subsidy repurposing includes R&D spending under the baseline for 2021–30 in addition to savings from subsidy removal. 130 The Economic Case for Nature Appendix G Decoupling of agricultural subsidies The budget for subsidy repurposing used in this report is based on the intermediate input and output subsidies paid to the agriculture sector (Table G.1). These are computed directly from the ad valorem subsidy rates in the Global Trade Analysis Project (GTAP) database. The GTAP 10 Data Base (Aguiar, Narayanan, and McDougall 2016) accounts for several agricultural domestic supports and subsidies for reference year 2014. The main data sources on agricultural domestic support include producer support estimates from OECD (2019) and the European Commission’s Joint Research Centre for EU member countries (Boulanger, Philippidis, and Jensen 2019). For other countries/regions, data on agricultural support are based on the most recent national input-output data provided by GTAP contributors. In general, agricultural support in the GTAP Data Base incorporates direct transfers to producers, which are based on actual budgetary transfers as well as forgone revenue by government and other agents (Huang 2013). It excludes the market price support component of the producer support estimate, which directly changes the domestic market prices of agricultural commodities compared with their border prices. The ad valorem subsidy rates are fixed in all future projections except when subsidies are explicitly removed and repurposed as investments in agricultural research and development (R&D) and as payments to ecosystem services. The GTAP–AgroEcological Zone model used in this report is a static model and only provides information on the global economy for a single year (for example, 2014, 2021, or 2030); a linear interpolation is used to estimate yearly subsidy payments over2021–30. Annual differences in subsidy payments under the 2021–30 business-as-usual scenario and the 2021–30 subsidy repurposing scenario are then used to calculate the total available budget for R&D and payment for ecosystem services expenditures (last column, table G.1). Appendices 131 Table G.1. Agricultural output and intermediate input subsidies, 2014, 2021, and 2030 (US$, millions) GTAP37 country unit Argentina Bangladesh Brazil Central America Canada China Colombia Rest of East Asia Egypt, Arab Rep. Ethiopia European Union Indonesia India Japan Korea, Rep. Morocco Madagascar Rest of M East N Africa Mexico Malaysia Nigeria Oceania Rest of Central Asia Other Europe Pakistan Philippines Poland Rest of South Asia Rest of Southeast Asia Russian Federation Rest of South America Rest of Sub-Saharan Africa Turkey United States Vietnam Angola+Congo, Dem. Rep. South Africa TOTAL 2014 0.2 0.1 2,534.8 1,728.5 1,478.1 19,216.7 60.7 1.0 1.3 8,542.8 2,165.7 7,532.6 2,533.7 1,145.3 0.2 - 1,621.0 1,627.9 1,066.3 174.0 308.3 3,907.8 6,543.3 0.2 596.5 347.1 1,547.0 143.6 1,213.6 303.2 2,798.8 1,886.4 5,169.4 6.2 - 110.1 76,312.0 2021 0.2 - 2,531.6 1,922.3 1,569.7 20,171.6 66.1 1.1 1.4 - 8,621.0 2,841.5 7,384.6 2,486.4 1,411.1 0.2 - 2,704.3 1,552.8 1,226.7 276.8 327.4 4,024.7 6,732.4 0.2 632.4 344.1 1,783.1 163.1 1,252.7 301.2 3,924.5 2,065.4 5,029.5 6.3 - 124.1 81,481.0 2030 under 2021–30 discounted, business-asbusiness-as-usual usual versus subsidy repurposing 0.2 0.9 0.1 0.3 2,583.5 14,208.2 2,353.6 11,877.5 1,405.9 8,235.5 21,059.5 114,565.5 73.9 389.1 1.1 6.1 1.4 7.8 0.1 0.3 8,435.4 47,338.3 4,103.3 19,195.7 6,743.9 39,131.9 2,315.6 13,309.0 1,574.8 8,300.2 0.2 0.8 - - 4,510.3 19,781.8 1,471.9 8,387.5 1,432.4 7,390.4 507.3 2,134.9 352.1 1,888.6 4,025.4 22,353.0 6,670.6 37,209.2 0.1 0.8 776.1 3,912.4 334.1 1,881.8 2,074.3 10,721.3 186.6 972.1 1,450.4 7,513.2 294.6 1,653.3 6,400.4 28,351.9 2,337.4 4,877.2 6.3 - 121.4 88,481.0 12,238.7 27,489.0 35.0 0.2 681.4 471,164.0 132 The Economic Case for Nature Appendix H GTAP core model details The Global Trade Analysis Project (GTAP) model is a multi-commodity, multiregional computable general equilibrium (CGE) model that tracks bilateral trade flows between all countries in the world and explicitly models the consumption and production of all commodities of each national economy (Corong et al. 2017; Hertel 1997). The standard version of the model is a comparative static CGE model that shows differences between possible states of the global economy for a given year—with and without policy or with respect to base year and future year. At the core of the GTAP model is an input-output accounting framework wherein all sources and uses of each good and economic endowment are accounted for. Figure H.1 presents a stylized framework of the GTAP model and summarizes the key flows across economic agents in the model. Consumption framework. The model has a single representative household for each region (regional household). The red line in figure H.1 represents income flows to the household. The household receives all gross factor payments net of the capital depreciation allowance (payments of factors of production (VOA)), plus the receipts from all indirect taxes (including export and import taxes (XTAX and MTAX, respectively)). Regional income is distributed across three broad categories—private household, government expenditures, and savings—by maximizing a top-level Cobb-Douglas utility function. Saving is a unitary good, and private household and government expenditures utilize sub-level utility functions to determine the consumption of each domestic commodity (value of domestic purchases of private household and government (VDPA and VDGA, respectively, shown by the black lines in figure H.1)) and imported commodity (value of imported purchases of private household and government (VIPA and VIGA, respectively, shown by the blue lines in figure H.1). The sub-level utility function for the private household is based on a constant differences of elasticities function (Hanoch 1975). On the one hand, this is less demanding than the flexible functional forms; on the other hand, it permits calibration of income elasticities and own-price elasticities independently, and importantly, it is non-homothetic. The sub-utility function for public expenditure is based on a constant elasticity of substitution (CES) utility function (Arrow et al. 1961). Production framework. Nested CES functions are used for modeling producer behavior for each region. At the top level of the production framework, producers combine aggregate value-added and intermediate inputs, according to a single CES function. Sub-level CES functions produce aggregated value added from each primary factor commodity and aggregated intermediate input from each purchased input. Factors of production, or endowments, are of three types: perfectly mobile (for example, labor and capital), partially mobile or sluggish (for example, land), and sector-specific factors (natural resources). Each purchased input can be sourced domestically or internationally and this is modeled using another sub-level CES function (value of domestic production and imports of firms (VDFA and VIFA, respectively, in figure H.1)). Appendices 133 Figure H.1. Stylized framework of the GTAP model Regional household PRIVEXP Taxes SAVE GOVEXP Taxes Private household Taxes Savings VOA Endw Government VDPA NETINV VDGA XTAX VIPA Producer VDFA VIFA VXMD VIGA MTAX Rest of the world Source: The figure is adapted from Corong et al. 2017. 134 The Economic Case for Nature International trade. The most notable restriction on trade in the GTAP model is that commodity sourcing is at the border: for each product, all domestic agents (that is, the private household, government, and producers) in an economy use the same mix of imports from different countries, although each agent chooses its own combination of imported and domestic products. There is also a two-level system of substitution between products from different sources—an import-domestic top-level CES function above an import-import sub-level CES function. Trade flows generate supply and demand for international transport services and this is accounted for in the model. There is also no international trade in primary factors in the standard version of GTAP. In this report, the GTAP Data Base version 10 for year 2014 is used. It represents globally consistent data on consumption, production, and international trade (including transportation and protection data); energy data; and carbon dioxide emissions for 140 regions and 57 commodities. These regions and commodities are aggregated into 37 regions and 17 commodity groups. The GTAP Data Base is composed of input-output table statistics, which are mainly contributed by members of the GTAP Network. The GTAP 10 Data Base includes separate input-output tables for 121 individual countries representing 98 percent of global gross domestic product and 92 percent of the world’s population. Key value flows in the database include input-output flows within each region, bilateral international trade flows, capital stock and savings information, international transport costs, domestic input and output subsidies, export subsidies and import tariffs, as well as revenue flows from taxes and tariffs. Most of the flows are measured at both tax-free and tax-paid prices (that is, taxes are implicitly accounted for). The key behavioral parameters provided with the GTAP Data Base include the source-substitution or Armington elasticities (used to differentiate goods by country or origin), the factor substitution elasticities, the factor transformation elasticities affecting the sluggish factors, the investment parameters, and the parameters governing the consumer demand elasticities. The first three sets of parameters are taken from external sources, and the rest are calibrated from the database. The standard GTAP model is implemented using the General Equilibrium Modelling PACKage (GEMPACK) suite of economic modeling software (Harrison and Pearson 1998). GEMPACK is distributed by the Centre of Policy Studies Knowledgebase at Victoria University, Melbourne, Australia (https://www.copsmodels.com/gempack.htm). Following the standard for the GEMPACK program, all the equations in the GTAP model are recorded not in levels (for example, $, millions) but in percentage change form. Due to nonlinearities in the formulas and update equations, which result in changes in the underlying shares and price elasticities, the solution requires nonlinear methods. The GTAP model can be run via command line as well as the Windows-based RunGTAP tool. RunGTAP is a visual interface to various GEMPACK programs and allows the user to run simulations interactively in a Windows environment using the GTAP general equilibrium model. No previous knowledge of the GEMPACK language or programming skills is necessary to use the program. Results and complementary information for further analysis are also provided in a Windows environment and can be accessed interactively. RunGTAP also has several add-on tools that can be helpful to users. The welfare decomposition tool permits the user to break down the regional equivalent variation metric into its component parts, including changes due to allocative efficiency, terms of trade, improved technology, and Appendices 135 endowments. The systematic sensitivity analysis tool allows uncertainty analysis in the model shocks and parameters, thereby generating the means and standard deviations of model output. Finally, the subtotals tool utilizes numerical integration techniques to decompose changes exactly in the model outputs as sums of the contributions made by the change in each exogenous variable (Harrison, Horridge, and Pearson 2000). The subtotals are particularly useful in understanding the key drivers of the model outcomes. All the input files are binary header array (HTTP Archive Format (HAR)) files, to keep the size of the files small. The HAR files are designed to work with the GEMPACK program. There is also a General Algebraic Modeling System (GAMS) version of the standard GTAP model and software exists for readily converting these HAR files to the GAMS data exchange file format, as well as to comma-separated values files. Capital and skilled and unskilled labor are perfectly mobile. This report assumes perfect labor mobility, which means that labor can move across sectors but not across skill types. In general, perfect mobility implies that the returns to each factor will be equated across all sectors. Therefore, there is a single economy-wide price for each mobile (capital and skilled and unskilled labor) factor, with market equilibrium determined by setting aggregate demand equal to (exogenous) supply. Land is partially mobile (or sluggish in GTAP terminology). The supply of aggregate land to individual activities is less than perfectly elastic, as there is a transformation frontier (constant elasticity of transformation) that moderates the movement of land across activities. This results in sector-differentiated land prices for each land-using sector. The economy-wide price of each land type is then calculated as the constant elasticity of transformation aggregate price of each land factor. 136 The Economic Case for Nature Appendix I Es ma ng the opportuni cost of protec ng 30 percent of terres ial land by 2030 The integrated ecosystem-economy model is run to assess the opportunity cost of protecting 30 percent of the Earth by 2030 (referred to here as the “30x30 target”). Other high-profile projects (Waldron et al. 2020) assess a similar question. Building on that effort, this report captures general equilibrium effects and, in particular, assesses gross domestic product and welfare losses that result from price changes and efficiency losses when land is removed from production (Table I.1). By directly incorporating a land supply curve parameterized uniquely for each agro-ecological zone (AEZ) and region in the GTAP-AEZ, the model used for this report provides a sophisticated tool to assess this question in a whole-economy framework. Selec ng a coun y-wide 30x30 conserva on scenario The first methodological choice is to formulate a reasonable assumption of how countries would split or share the burden of reaching the 30x30 target. This choice is steeped in the long history of international negotiations on multiple environmental topics, most notably for climate change, that include ethical debates about how historical contributions to environmental damage should be weighed against, for instance, future potential to mitigate damage. For this report, a single middle-of-the-road-scenario is presented to illustrate how the model can estimate the opportunity cost of large land protection policies, although it is recognized that many alternative scenarios with different national protection goals could be analyzed in future work. The global protection plan that is used assumes that every GTAP country unit would increase protected land area by 15.3 percent of the total area in the GTAP country unit, such that when summed, at the global level, with existing global protected land (14.7 percent of global terrestrial surface area), the 30x30 target is achieved. This plan is a midway point between two more extreme solutions, namely: (i) protect the most important land regardless of country (which would result in much higher protection responsibility in tropical or high–conservation value countries); and (ii) increase protection to 30 percent within each country (which would favor countries with more ambitious historic efforts and which would require zero expansion in countries that already are at 30 percent protection). This spectrum is discussed in more depth in Waldron et al. 2020 and is reflected by the range of scenarios defined therein. A minor extension of the rule above Appendices 137 Table I.1. Scenarios defined by Waldron et al. (2020) for meeting the 30x30 target Realm ID Category Name Description T T1 Non-PAexpansion Reference The counterfactual for comparison of not expanding protected areas baseline Scenario (REF) post-2020. Maintain the protected area estate at its current coverage (as of July 2019). M M1 Non-PAexpansion Reference The counterfactual for comparison of not expanding protected areas baseline Scenario (REF) post-2020. Maintain the marine protected area estate at its current coverage (as of July 2019). T T2 Productionfocused Three Conditions Protect all global habitat that retains a state of minimal human (THC) intervention (‘wilderness’), plus existing PAs T T3 Productionfocused Harsh Political First disqualify from protection any area needed for agricultural Reality (HPR) production up to 2050, then choose the areas of non-disqualified land that optimally minimises global species extinctions (plus existing PAs). M M3 Productionfocused Harsh Political Marine reserves are not allowed on any areas of ocean that are currently Reality Marine high-value fishing grounds. The existing MPA system is then expanded to (HPR) the next-best set of ocean sites to preserve marine biodiversity T T4 Biodiversityfocused Biodiversity/ Protect all wilderness, KBAs and existing PAs, plus the optimal set of (hybrid) Wilderness all other sites needed to maintain global species viabilities (based on consensus (BIWI) minimum range coverage). NB 43% terrestrial coverage, compensated by lower coverage in the paired marine scenario M M4 Biodiversityfocused Biodiversity/ All existing MPAs and marine wildernesses are protected, then the (hybrid) Wilderness optimal set of areas needed to prevent marine biodiversity decline (NB consensus (BIWI) cover 26% of marine global area) T T5 Biodiversity/ Biodiversity/ Production Production Compromise (BPC) Compromise Add ~5% more of the land surface to the existing PA network (up to 20% planetary land), choosing sites to optimally reduce global species extinctions. The remaining 10% of new PAs are not allowed to go on potential agricultural land, but are placed in the next-best set of sites instead (using the same criteria) M M5a Biodiversity/ Production Compromise 50:50 EEZ (5050EEZ) Expand the existing MPA system to the 30% of ocean that optimally reduce global species extinctions, but 50% of the protected area inside Exclusive Economic Zones permits sustainable fishing, while the other 50% bans all economic activity M M5b Biodiversity/ Production Compromise 50:50 Coastal (5050COAST) Expand the existing MPA system to the 30% of ocean that optimally reduce global species extinctions, but 50% of the protected area immediately adjacent to coasts (where small scale fisheries tend to operate) permits sustainable fishing, while the other 50% bans all economic activity T T6 Biodiversityfocused Save Species from Expand the existing PA system to the 30% of land that optimally reduce Extinction (SSE) global species extinctions T T7 Biodiversityfocused Global Deal for Conserve a wide range of sites that have biodiversity importance under Nature (GDN) different criteria, including current PAs, sites with rare or endemic species, areas needed for wide-ranging mammals, etc. (see original paper). M M6 Biodiversityfocused Top 30 (TOP30) Expand the existing MPA system to the 30% of ocean that optimally reduce global species extinctions Source: Adapted from Waldron et al. 2020 138 The Economic Case for Nature was necessary, namely that because some GTAP country units did not have 15.3 percent of their area still available to be conserved (because, for example, they already have nearly all of their area preserved, or because there is no conservation benefit from expanding protection beyond a certain level), their protection area is instead increased by whatever was left in that GTAP country unit, and then the protected areas in all the other GTAP country units are slightly increased to ensure that the global goal was conserved. Table I.2 presents the resulting increase in area for each GTAP country unit. An additional assumption that must be specified is which grid cells within a GTAP country unit should be protected. Waldron et al. (2020) specify many different scenarios that address this point. Specifically, their Global Deal for Nature scenario is followed, using biodiversity and carbon storage indicators to identify the highest-value conservation grid cells (see geospatial computation steps below for details). Lacking spatially explicit information (or even exact regional totals) of where each scenario protects land, this report produces spatially explicit maps5 that implement the Global Deal for Nature scenario with a new level of spatial and regional explicitness than was previously available. Table I.2. Assumed protected area goals in the GTAP country units, geographic regions, and income groups GTAP37 country units, income groups and geographic regions Argentina Bangladesh Brazil Canada China Colombia Central Amer Egypt, Arab Rep. Ethiopia European Union Rest of East Asia Indonesia India Japan GTAP 226 (ISO 3 Code) ARG BGD BRAZIL CAN CHIHKG COL C_C_Amer EGY ETH EU27 E_Asia IDN INDIA JAPAN New protected land (ha) 44,916,779 2,396,960 107,139,304 159,836,030 166,127,098 17,013,895 9,542,450 14,792,445 16,317,814 53,826,931 24,885,780 29,563,645 53,543,895 5,165,156 2014 protected land (ha) 28,094,566 549,255 254,093,337 98,011,765 17,557,910 18,851,916 20,062,648 16,117,512 22,258,862 99,286,868 30,430,229 22,445,363 16,770,157 8,061,912 Total land (ha) 277,909,800 13,880,510 849,973,781 986,977,448 941,512,848 113,478,693 73,135,228 98,389,175 113,014,222 398,658,007 168,838,351 186,870,532 314,567,125 36,789,141 5. All maps presented in Appendix I were resampled to 5-arc-minute grid cells for visual clarity. Appendices 139 GTAP37 country units, income groups and geographic regions GTAP 226 (ISO 3 Code) Korea, Rep. Morocco Madagascar Rest of Middle East and North Africa Mexico Malaysia Nigeria Oceania Rest of Central Asia Other Europe Pakistan Philippines Poland Rest of Southeast Asia Rest of South Asia Russian Federation Rest of Sub-Saharan Africa Rest of South America Turkey United States Vietnam Angola+Congo, Dem. Rep. South Africa High-income Low-income Lower-middle income Upper-middle income East Asia & Pacific Europe & Central Asia Latin America & Caribbean Middle East & North Africa North America South Asia Sub-Saharan Africa KOR MAR MDG MEAS_NAfr MEX MYS NGA Oceania Oth_CEE_CIS Oth_Europe PAK PHL POL R_SE_Asia R_S_Asia Russia S_S_AFR S_o_Amer TUR USA VNM XAC ZAF High-income Low-income Lower-middle income Upper-middle income East Asia & Pacific Europe & Central Asia Latin America & Caribbean Middle East & North Africa North America South Asia Sub-Saharan Africa New protected land (ha) 1,446,899 9,697,848 9,053,681 156,455,520 30,019,571 5,140,359 14,197,049 121,126,507 87,217,685 6,876,338 14,072,884 4,441,680 3,400,711 24,180,065 13,773,245 268,312,151 247,273,978 69,896,505 13,946,955 147,907,681 4,983,678 56,954,772 19,019,604 670,988,753 136,291,002 351,155,667 876,028,125 387,060,868 433,591,319 278,389,467 181,633,696 307,840,543 83,786,983 362,160,670 2014 protected land (ha) 1,767,833 14,143,196 8,789,718 53,368,517 27,839,082 4,265,296 11,861,724 173,537,475 44,826,496 8,252,325 9,056,672 4,491,439 12,282,130 26,785,670 12,825,889 197,773,178 293,117,526 143,233,169 228,971 108,407,466 5,002,920 41,023,513 19,132,931 628,683,623 153,095,887 305,671,665 787,154,261 294,346,048 362,680,309 491,882,399 84,465,506 206,622,816 39,201,974 395,406,384 Total land (ha) 9,815,104 68,080,056 59,143,896 923,532,700 194,799,987 32,854,614 90,821,954 847,211,042 529,908,171 46,496,697 87,326,327 29,194,886 31,195,982 161,268,790 89,429,030 1,690,054,326 1,668,389,927 531,979,027 77,798,242 931,030,830 32,720,819 357,790,879 124,914,932 4,360,545,430 911,110,359 2,258,706,521 5,659,390,772 2,447,076,127 2,774,200,436 2,040,210,915 1,094,664,040 1,918,750,418 505,202,992 2,409,648,154 140 The Economic Case for Nature Iden fying a high-resolu on land use, land cover con gura on that meets the 30x30 rget Map I.1. The first step that is necessary to create the 30 percent protection scenario is the generation of an accurate, 10-arc-second grid (roughly 300 meters at the equator) resolution map of protected areas. This was done by rasterizing all the protected areas listed in the World Database on Protected Areas (Bingham et al. 2019), harmonizing them with the European Space Agency–Climate Change Initiative (ESA-CCI) land use, land cover maps, and expressing them as hectares protected per 10-arc-second grid cell. This map, which is presented as map I.1. When summed up, the protected area exactly equals the 14.7 percent of protected land assumed in the Waldron report (Waldron et al. 2020). Current protected land from the world database Currently protected land 8605 hectares per 5-arc-minute grid cell 0 Most Least land Appendices 141 Next, a map that scores the relative value of each grid cell based on the amount of biodiversity and carbon storage present is produced. Specifically, the PREDICTS biodiversity model (described in section 2 of the main text) is used to estimate the relative species protection impact of each grid cell and carbon storage data from Johnson et al. 2019, which harmonize a variety of recent carbon storage data sets to attain the global coverage needed. To weight carbon storage and biodiversity equally, the carbon storage data were first min-max scaled such that the lowest carbon storage pixel was 0 and the highest was 1. This scaled map was then used in the following equation for conservation value as a simple weighted sum present in each grid cell x: conservation_valuex=0.5*biodiversityx+0.5*normalized_carbon_storagex These conservation values are presented in map I.2, where darker green indicates a higher overall conservation score. Map I.2. Conservation score based on a weighted sum of normalized carbon storage and biodiversity (darker green indicates higher conservation value) Conservation score index 0 0.001 0.01 0.1 0.26 0.325 0.39 0.45 0.5 142 The Economic Case for Nature The values calculated for map I.2 are then ranked globally and assigned a prioritization score, such that the value 1 is assigned to the highest-value grid cell globally, the value 2 is assigned to the next highest-value, and continuing for all the grid cells. This is presented in map I.3, where red represents the first priority grid cells and blue represents the last priority cells. The difference between maps E.2 and E.3 is that map I.2 represents the absolute conservation score, while map I.3 represents the conservation importance of each cell relative to all other cells. Map I.3. Conservation prioritization of grid cells based on conservation score (red cells indicate highest priority; blue cells indicate lowest priority) Conservation prioritization Highest priority Lowest priority Appendices 143 Based on the conservation prioritization in map I.3, an algorithm that identifies which grid cells in each region–agro-ecological zone (AEZ) should be protected to maximize the conservation value protected within each region-AEZ while also meeting the explicit goal for protected hectares in the region-AEZ (the method discussed above, with the explicit values presented in Table I.2). The algorithm is similar to that used in Johnson et al. (forthcoming), which proceeds iteratively through the conservation prioritization while keeping track of specific region-AEZ protection. The results of this algorithm are presented in map I.4, which overlays the newly protected hectares in purple on the current protected land (green). Map I.4. Current protected land (green) and proposed new protected land (purple) to reach the 30x30 goal while maximizing biodiversity and carbon storage Proposed new protected land Currently protected 8605 hectares per 5-arc-minute grid cell Most 0 Least land 144 The Economic Case for Nature The impact of protecting land on the economy depends on the extent to which protection prevents grid cells from being used as inputs for agriculture, grazing, and managed forestry (or other land-intensive sectors) production. This links to the endogenous land use supply function in the economic model. In particular, one needs to estimate which of the protected area grid cells (in map I.4) are cells that are in current or potential economic use zones. This depends on land availability which is estimated using the land supply function described in section 2 in the main text. These data, which report the number of hectares present in each 10-arc-second grid cell that are currently in agriculture or able to be converted into agriculture based on growing conditions and other constraints, is presented in map I.5 (resampled to 5-arc-minute grid cells for visual clarity). Map I.5. Current and potential agricultural land (darker magenta indicates more hectares are available for agriculture or are currently in use) Current and potential agricultural land 8605 hectares per 5-arc-minute grid cell 0 Most Least land Appendices 145 The key input into the economic component of the integrated ecosystem-economy model is based on the number of hectares that are newly preserved to meet the 30 percent goal (map I.4) that also are on grid cells that have current or potential economic use (map I.5), where potentially usable is defined based on soil, topographic, climatic, and other conditions. These locations, which have conflict between protection and economic use are shown in map I.6, with darker orange indicating more hectares in conflict, which is used to parameterize the land supply curves. Map I.6. Current or potential agricultural land displaced by new protected land (darker orange indicates greater conflict between protection and economic use) Displaced cropland 8605 hectares per 5-arc-minute grid cell 0 Most Least land 146 The Economic Case for Nature Finally, to visualize these layers all together, map I.7 shows the currently protected hectares in green, potentially protected areas that are not in conflict with agricultural expansion in purple, and protected areas that are in conflict in orange. The next step is to calculate the total number of hectares in conflict for each GTAP country unit. This value then shifts leftward the asymptote (and thus also the shape) of the land supply curve (depicted in Figure 2 of the main text). The reduction in land available increases the elasticity (slope of the intersect), reduces the quantity of land actually used (because the rent is higher), and increases the equilibrium land rent. The land supply curve relates how changes in land used by the economy determine the land rental rate. Protecting land shifts the asymptote inward, which will decrease the amount of economically used land and increase the land rental rate (Figure 3 in the main text). Map I.7. Current protected land (green), newly protected land with no agricultural conflict (purple), and newly protected land that displaces current or potential agricultural land (orange) Displaces cropland Newly protected (no conflict) Currently protected 8605 hectares per 5-arc-minute grid cell Most 0 Least land Appendices 147 The final step in the process is to run GTAP-1 with this shock to calculate the new endogenous value for land use change, and then to apply this change in the land use change downscaling step (Spatial Economic Allocation Landscape Simulator) with the constraint that the expansion cannot happen in the protected areas. This new land use map is then the new input to InVEST and the subsequent GTAP-2. Comparing the total gross domestic product (GDP) outputted by the GTAP-2 with this land use contraction shock with business-as-usual (unconstrained), GDP defines the total opportunity cost (or welfare effects or other sectoral changes) that would result from protecting 30 percent of the terrestrial Earth’s surface. 148 The Economic Case for Nature References Acemoglu D., A. Ozdaglar, and A. Tahbaz-Salehi. 2017. “Microeconomic Origins of Macroeconomic Tail Risks.” American Economic Review 107 (1): 54–108. https://doi.org/10.1257/aer.20151086. Aguiar, A., B. Narayanan, and R. McDougall. 2016. “An Overview of the GTAP 9 Data Base.” Journal of Global Economic Analysis 1 (1): 181–208 (accessed February 5, 2018), https://jgea.org/resources/jgea/ojs/ index.php/jgea/article/view/23. Alston, J. M., J. M. Beddow, and P. G. Pardey. 2009. “Agricultural research, productivity, and food prices in the long run.” Science, 325 (5945): 1209-1210. Alston, J. M., M. A. Andersen, J. S. James, and P. G. Pardey. 2011. “The Economic Returns to U.S. Public Agricultural Research.” American Journal of Agricultural Economics 93 (5): 1257–77. https://doi. org/10.1093/ajae/aar044. Arrow K. J., H. B. Chenery, B. S. Minhas, and R. M. Solow. 1961. “Capital-Labor Substitution and Economic Efficiency.” Review of Economics and Statistics 43 (3): 225–50. Baldos, U. 2017. “Development of GTAP Version 9 Land Use and Land Cover Database for Years 2004, 2007 and 2011.” GTAP Research Memorandum No. 30, Global Trade Analysis Project (GTAP), Purdue University, West Lafayette, IN. https://www.gtap.agecon.purdue.edu/resources/res_display.asp?RecordID=5424. Baldos, U. L. C., and E. Corong. 2020. “Development of GTAP version 10 Land Use and Land Cover Data Base for years 2004, 2007, 2011 and 2014.“ GTAP Research Memoranda 6187, Center for Global Trade Analysis, Department of Agricultural Economics, Purdue University Baldos, U. L. C., K. O. Fuglie, and T. W. Hertel. 2020. “The Research Cost of Adapting Agriculture to Climate Change: A Global Analysis to 2050.” Agricultural Economics 51 (2): 207–20. https://doi.org/10.1111/ agec.12550. Banerjee, O., K. J. Bagstad, M. Cicowiez, S. Dudek, M. Horridge, J. R. R. Alavalapati, M. Masozera, E. Rukundo, and E. Rutebuka. 2020. “Economic, Land Use, and Ecosystem Services Impacts of Rwanda’s Green Growth Strategy: An Application of the IEEM+ESM Platform.” Science of the Total Environment 729. https://doi.org/10.1016/j.scitotenv.2020.138779. Banerjee, O., M. Cicowiez, M. Horridge, and R. Vargas. 2016. “A Conceptual Framework for Integrated Economic– Environmental Modeling.” Journal of Environment & Development 25 (3): 276–305. https://doi. org/10.1177/1070496516658753. Banerjee, O., M. Cicowiez, M. Horridge, and R. Vargas. 2019. “Evaluating Synergies and Trade-offs in Achieving the SDGs of Zero Hunger and Clean Water and Sanitation: An Application of the IEEM Platform to Guatemala.” Ecological Economics 161: 280–91. https://doi.org/10.1016/j.ecolecon.2019.04.003. Barrett, S. 1994. “Self-Enforcing International Environmental Agreements.” Oxford Economic Papers 46: 878–94. http://www.jstor.org/stable/2663505. References 149 Bauer, D. M., and I. S. Wing. 2016. “The Macroeconomic Cost of Catastrophic Pollinator Declines.” Ecological Economics 126: 1–13. https://doi.org/10.1016/j.ecolecon.2016.01.011. Baumol, W., and W. Oates. 1988. The Theory of Environmental Policy. Cambridge University Press. Beintema, N., G.-J. Stads, K. Fuglie, and P. Heisey. 2012. “ASTI Global Assessment of Agricultural R&D Spending: Developing Countries Accelerate Investment.” International Food Policy Report, Agricultural Science and Technology Indicators, Global Forum on Agricultural Research, International Food Policy Research Institute, Washington, DC. Bingham, H. C., D. J. Bignoli, E. Lewis, B. MacSharry, N. D. Burgess, P. Visconti, Marine Deguignet, et al. 2019. “Sixty Years of Tracking Conservation Progress Using the World Database on Protected Areas.” Nature Ecology and Evolution 3 (5): 737–43. Bolton, P., M. Despres, L. A. Pereira da Silva, F. Samama, and R. Svartzman. 2020. “The Green Swan: Central Banking and Financial Stability in the Age of Climate Change.” Working Paper 5, Bank for International Settlements and Bank of France, Basel, Switzerland. https://www.bis.org/publ/othp31.pdf. Boulanger, P., G. Philippidis, and H. G. Jensen. 2019. “GTAP v. 10 2014 Domestic Support in the European Union.” Background paper for the GTAP model’s database, Purdue University, West Lafayette, IN. Brundtland, G. H. 1987. Our Common Future: Report of the World Commission on Environment and Development. Oxford: Oxford University Press. Burney, J. A., S. J. Davis, and D. B. Lobell. 2010. “Greenhouse Gas Mitigation by Agricultural Intensification.” Proceedings of the National Academy of Sciences 107 (26): 12052–57. https://doi.org/10.1073/ pnas.0914216107. Cai, Y., T. Lenton, and T. Lontzek. 2016. “Risk of Multiple Interacting Tipping Points Should Encourage Rapid CO2 Emission Reduction.” Nature Climate Change 6: 520–25. https://doi.org/10.1038/nclimate2964. CBD Secretariat (Secretariat of the Convention on Biological Diversity). 2020a. “Update of the Zero Draft of the Post-2020 Global Biodiversity Framework.” CBD/POST2020/PREP/2/1. CBD Secretariat, Montreal, Quebec, Canada. https://www.cbd.int/doc/c/3064/749a/0f65ac7f9def86707f4eaefa/post2020prep-02-01-en.pdf. CBD Secretariat (Secretariat of the Convention on Biological Diversity). 2020b. Global Biodiversity Outlook 5. Montreal, Quebec, Canada: CBD Secretariat. https://www.cbd.int/gbo5. Chaplin-Kramer, R., R. P. Sharp, C. Weil, E. M. Bennett, U. Pascual, K. K. Arkema, K. A. Brauman, et al. 2019. “Global Modeling of Nature’s Contributions to People.” Science 366 (6462): 255–58. doi:10.1126/ science.aaw3372. Chen, S., and M. Ravallion. 2000. “How Did the World’s Poorest Fare in the 1990s?” Global Poverty Monitoring Database, World Bank, Washington, DC. http://www.worldbank.org/research/povmonitor/method.htm. Chen, Y.-H. Henry, S. Paltsev, J. Reilly, J. Morris, V. Karplus, A. Gurgel, N. Winchester, P. Kishimoto, É. Blanc, and M. H. Babiker. 2017. “The MIT Economic Projection and Policy Analysis (EPPA) Model: Version 5.” In Joint Program Technical Note 16. Joint Program on the Science and Policy of Global Change, Massachusetts Institute of Technology, Cambridge, MA. https://globalchange.mit.edu/ publication/16620. 150 The Economic Case for Nature Corong, E. L., T. W. Hertel, R. McDougall, M. E. Tsigas, and D. van der Mensbrugghe. 2017. “The Standard GTAP Model, Version 7.” Journal of Global Economic Analysis 2 (1): 1–119 (accessed February 5, 2018), https://jgea.org/resources/jgea/ojs/index.php/jgea/article/view/47. Crépin, A. S., R. Biggs, S. Polasky, M. Troell, and A. De Zeeuw. 2012. “Regime Shifts and Management.” Ecological Economics 84: 15–22. Crossman, N., O. Banerjee, L. Brander, P. Verburg, and J. Hauck. 2018. Global Socio-Economic Impacts of Future Changes in Biodiversity and Ecosystem Services: State of Play and Approaches for New Modelling. Adelaide, Australia: University of Adelaide. Cuaresma, J. C. 2017. “Income Projections for Climate Change Research: A Framework Based on Human Capital Dynamics.” Global Environmental Change 42: 226–36. http://doi.org/10.1016/j.gloenvcha.2015.02.012 Dasgupta, P. 2021. The Economics of Biodiversity: The Dasgupta Review. London: HM Treasury. Dixon P., M. Rimmer, H. Van Meijl, and A. Tabeau. 2012. “RED Versus REDD: The Battle between Extending Agricultural Land Use and Protecting Forest.” Paper for International Association of Agricultural Economists Conference 2012, Foz do Iguaçu, Brazil. Dobson, A. P., S. L. Pimm, L. Hannah, L. Kaufman, J. A. Ahumada, A. W. Ando, A. Bernstein, et al. 2020. “Ecology and Economics for Pandemic Prevention.” Science 369 (6502): 379–81. Eickhout B., H. Van Meijl, A. Tabeau, and E. Stehfest. 2009. “The Impact of Environmental and Climate Constraints on Global Food Supply.” In Economic Analysis of Land Use in Global Climate Change Policy, edited by T. Hertel, S. Rose, and R. Tol. Routledge. FAO (Food and Agriculture Organization). 2018. The State of World Fisheries and Aquaculture 2018: Meeting the Sustainable Development Goals. Rome: FAO. FAO and UNEP (Food and Agriculture Organization and United Nations Environment Programme). 2020. The State of the World’s Forests 2020: Forests, Biodiversity and People. Rome: FAO. https://doi. org/10.4060/ca8642en. FCPF (Forest Carbon Partnership Facility). 2020. FCPF 2020 Annual Report. Washington, DC: World Bank Group. https://www.forestcarbonpartnership.org/system/files/documents/FCPF percent202020 percent20Annual percent20Report_Web_update.pdf. Fischer, G., F. Nachtergaele, S. Prieler, H. T. van Velthuizen, L. Verelst, and D. Wiberg. 2008. Global AgroEcological Zones Assessment for Agriculture (GAEZ 2008). Laxenburg, Austria: International Institute for Applied Systems Analysis, and Rome: Food and Agriculture Organization. Free, J. B. 1993. Insect Pollination of Crops, second edition. Academic Press. Fuglie, K. 2017. “R&D Capital, R&D Spillovers, and Productivity Growth in World Agriculture.” Applied Economic Perspectives and Policy. https://doi.org/10.1093/aepp/ppx045. Fullerton, D. 2008. “Distributional Effects of Environmental and Energy Policy: An Introduction.” Working Paper 14241, National Bureau of Economic Research, Cambridge, MA. Gibb, R., D. W. Redding, K. Q. Chin, C. A. Donnelly, T. M. Blackburn, T. Newbold, and K. E. Jones. 2020. “Zoonotic Host Diversity Increases in Human-Dominated Ecosystems.” Nature 584 (7821): 398–402. References 151 Griliches, Z. 1979. “Issues in Assessing the Contribution of Research and Development to Productivity Growth.” Bell Journal of Economics 10 (1): 92–116. https://doi.org/10.2307/3003321. Griscom, B. W., J. Adams, P. W. Ellis, R. A. Houghton, G. Lomax, D. A. Miteva, W. H. Schlesinger, et al. 2017. “Natural Climate Solutions.” Proceedings of the National Academy of Sciences 114 (44): 11645–50. Hanoch, G. 1975. “Production and Demand Models with Direct or Indirect Implicit Additivity.” Econometrica 43 (3): 395–419. http://www.jstor.org/stable/1914273. Harrison, W. J., J. M. Horridge, and K. R. Pearson. 2000. “Decomposing Simulation Results with Respect to Exogenous Shocks.” Computational Economics 15 (3): 227–49. Harrison, W., and K. Pearson. 1998. “An Introduction to GEMPACK.” GEMPACK Document No. GPD-1, 4th edition, Centre of Policy Studies and Impact Project, Monash University, Melbourne, Victoria, Australia. Heisey, P. W., and K. O. Fuglie. 2018. “Agricultural Research Investment and Policy Reform in High-Income Countries.” ERR-249, U.S. Department of Agriculture, Economic Research Service, Washington, DC. https://ageconsearch.umn.edu/record/276235/. Heisey, P., S. L. Wang, and K. Fuglie. 2011. “Public Agricultural Research Spending and Future U.S. Agricultural Productivity Growth: Scenarios for 2010-2050.” Economic Brief EB-17, Economic Research Service, U.S. Department of Agriculture, Washington, DC. Hertel, T. 1997. “Global Trade Analysis: Modeling and Applications.” Global Trade Analysis Project, Department of Agricultural Economics, Purdue University, West Lafayette, IN. Hertel, T. W., S. K. Rose, and R. S. J. Tol. 2009. Economic Analysis of Land Use in Global Climate Change Policy. Routledge. Hertel, T. W., T. A. West, J. Börner, and N. B. Villoria. 2019. “A Review of Global-Local-Global Linkages in Economic Land-Use/Cover Change Models.” Environmental Research Letters 14 (5): 053003. Hijmans, R. J., S. E. Cameron, J. L. Parra, P. G. Jones, and A. Jarvis. 2005. International Journal of Climatology 25: 1965 78. WorldClim 1.4: Current conditions (~1960-1990) and WorldClim 1.4 downscaled (CMIP5) data, by www.worldclim.org. http://biogeo.ucdavis.edu/data/climate/worldclim/1_4/grid/cur/prec_30s_bil.zip, http://biogeo.ucdavis.edu/data/climate/cmip5/30s/he26pr50.zip, http://biogeo.ucdavis.edu/data/climate/cmip5/30s/he60pr50.zip, http://biogeo.ucdavis.edu/data/climate/cmip5/30s/he85pr50.zip. Hoegh-Guldberg, O., D. Jacob, M. Taylor, T. Guillen Bolanos, M. Bindi, S. Brown, I.A. Camilloni, et al. 2019. “The Human Imperative of Stabilizing Global Climate Change at 1.5°C.” Science 365 (6459): eaaw6974. doi:10.1126/science.aaw6974. Huang, H. 2013. “Agricultural Domestic Support.” In GTAP 8 Data Base Documentation, chapter 10.A, West Lafayette, IN: Center for Global Trade Analysis, Purdue University. https://www.gtap.agecon.purdue. edu/resources/res_display.asp?RecordID=4296. Huffman, W. E. 2009. “Measuring Public Agricultural Research Capital and Its Contribution to State Agricultural Productivity.” No. 09022, Department of Economics, Iowa State University, Ames, IA. Hummels, D. 1999. “Toward a Geography of Trade Costs.” No. 1237-2019-190, GTAP Working Papers 1162, Center for Global Trade Analysis, Department of Agricultural Economics, Purdue University, West Lafayette, IN. 152 The Economic Case for Nature Hurtt, G. C., L. Chini, R. Sahajpal, S. Frolking, B. L. Bodirsky, K. Calvin, et al. 2020. “Harmonization of global land use change and management for the period 850–2100 (LUH2) for CMIP6.” Geoscientific Model Development 13 (11): 5425-5464. IIASA (International Institute for Applied Systems Analysis). 2015. “SSP Database 2012-2015: Preliminary Scenarios.” IIASA, Laxenburg, Austria. https://tntcat.iiasa.ac.at/SspDb. IPBES (Intergovernmental Science-Policy Platform on Biodiversity and Ecosystem Services). 2019. Global Assessment Report on Biodiversity and Ecosystem Services of the Intergovernmental Science-Policy Platform on Biodiversity and Ecosystem Services, edited by E. S. Brondizio, J. Settele, S. Díaz, and H. T. Ngo. Bonn, Germany: IPBES Secretariat. IPCC (Intergovernmental Panel on Climate Change). 2018. Global Warming of 1.5°C.An IPCC Special Report on the Impacts of Global Warming of 1.5°C above Pre-Industrial Levels and Related Global Greenhouse Gas Emission Pathways, in the Context of Strengthening the Global Response to the Threat of Climate Change, Sustainable Development, and Efforts to Eradicate Poverty, edited by V. Masson-Delmotte, P. Zhai, H.-O. Pörtner, D. Roberts, J. Skea, P. R. Shukla, A. Pirani, et al. Geneva: IPCC. IPCC (Intergovernmental Panel on Climate Change). 2019. “Summary for Policymakers.” In Climate Change and Land: An IPCC Special Report on Climate Change, Desertification, Land Degradation, Sustainable Land Management, Food Security, and Greenhouse Gas Fluxes in Terrestrial Ecosystems, edited by P. R. Shukla, J. Skea, E. Calvo Buendia, V. Masson-Delmotte, H.-O. Pörtner, D. C. Roberts, P. Zhai, et al. Geneva: IPCC. Johnson, J. A. 2019. “Globally Harmonized Carbon Storage Data.” bioRxiv 727750. Johnson, J., U. L. Baldos, T. Hertel, C. Nootenboom, S. Polasky, and T. Roxburgh. 2020. “Global Futures: Modelling the Global Economic Impacts of Environmental Change to Support Policy-Making.” Technical Report, Center for Global Trade Analysis, Department of Agricultural Economics, Purdue University, West Lafayette, IN. Johnson, J., U. L. Baldos, T. Hertel, and S. Polasky. 2019. “Assessing the Economic Impacts of Ecosystem Services within the GTAP Framework.” https://www.gtap.agecon.purdue.edu/resources/res_display. asp?RecordID=5724. Johnson, J. A., K. Kennedy, J. Oakleaf, and J. Kiesiker. Forthcoming. “Energy Matters: Mitigating the Impacts of Future Land Expansion Will Require Managing Energy and Extractive Footprints.” Ecological Economics. Johnson, J. A., C. F. Runge, B. Senauer, and S. Polasky. 2016. “Global Food Demand and Carbon-Preserving Cropland Expansion under Varying Levels of Intensification.” Land Economics 92 (4): 579–92. Judd, M., J. Boyce, and R. Evenson. 1991. “Investment in Agricultural Research and Extension Programs: A Quantitative Assessment.” In Research and Productivity in Asian Agriculture, edited by R. Evenson and C. Pray, 7–46. Cornell University Press. Kennedy, C. M., E. Lonsdorf, M. C. Neel, N. M. Williams, T. H. Ricketts, R. Winfree, R. Bommarco, et al. 2013. “A Global Quantitative Synthesis of Local and Landscape Effects on Wild Bee Pollinators in Agroecosystems.” Ecology Letters 16: 584–99. Kim, H., I. Rosa, R. Alkemade, P. Leadley, G. Hurtt, A. Popp, D. P. van Vuuren, et al. 2018. “A Protocol for an Intercomparison of Biodiversity and Ecosystem Services Models Using Harmonized Land-Use and References 153 Climate Scenarios.” Geoscientific Model Development Discussions 11 (11): 4537–62. ff10.5194/gmd-114537-2018ff. ffhal-01983410f. Klinsky, S., and H. Winkler. 2018. “Building Equity In: Strategies for Integrating Equity into Modelling for a 1.5°C World.” Philosophical Transactions of the Royal Society A: Mathematical, Physical, and Engineering Sciences 376: 2119. doi:10.1098/rsta.2016.0461. Kremen, C. 2005. “Managing Ecosystem Services: What Do We Need to Know about Their Ecology?” Ecology Letters 8: 468–79. Kriegler, E., J. W. Hall, H. Held, R. Dawson, and H. J. Schellnhuber. 2009. PNAS 106 (13): 5041–46. doi:10.1073/ pnas.0809117106. Laborde, D., A. Mamun, W. Martin, V. Piñeiro, and R. Vos. 2020. “Modeling the Impacts of Agricultural Support Policies on Emissions from Agriculture.” World Bank, Washington, DC. Lange, G. M., Q. Wodon, and K. Carey, eds. 2018. The Changing Wealth of Nations 2018: Building a Sustainable Future. Washington, DC: World Bank. Lapola, D. M., P. Pinho, C. A. Quesada, B. B. N. Strassburg, A. Rammig, B. Kruijt, F. Brown, et al. 2018. “Limiting the High Impacts of Amazon Forest Dieback with No-Regrets Science and Policy Action.” PNAS 115 (46): 11671–79. doi:10.1073/pnas.1721770115. Liu, Z., and A. Kontoleon. 2018. “Meta-Analysis of Livelihood Impacts of Payments for Environmental Services Programmes in Developing Countries.” Ecological Economics 149: 48–61. Lotze, H. K., D. P. Tittensor, A. Bryndum-Buchholz, T. D. Eddy, W. W. Cheung, E. D. Galbraith, M. Barange, et al. 2019. “Global Ensemble Projections Reveal Trophic Amplification of Ocean Biomass Declines with Climate Change.” Proceedings of the National Academy of Sciences. 116 (26): 12907–12. https://doi. org/10.1073/pnas.1900194116. Lovejoy, T. E., and L. Hannah. 2019. Biodiversity and Climate Change: Transforming the Biosphere. New Haven, CT: Yale University Press. Lovejoy, T. E., and C. Nobre. 2018. “Amazon Tipping Point.” Science Advances 4 (2): eaat2340. doiI:10.1126/ sciadv.aat2340. Lovejoy, T. E., and C. Nobre. 2019. “Amazon Tipping Point: Last Chance for Action.” Science Advances 5 (12): eaba2949. doi:10.1126/sciadv.aba2949. Mace, G. M., M. Barrett, N. B. Burgess, S. E. Cornell, R. Freeman, M. Grooten, and A. Purvis. 2018. “Aiming Higher to Bend the Curve of Biodiversity Loss.” Nature Sustainability 1 (9): 448–51. Mackie, A., and I. Haščič. 2019. “The Distributional Aspects of Environmental Quality and Environmental Policies: Opportunities for Individuals and Households.” OECD Green Growth Papers No. 2019/02, OECD Publishing, Paris. https://doi.org/10.1787/e0939b52-en. Miller, D. C., J. Z. Cheek, P. Newton, C. Wildburger, T. C. H. Sunderland, and P. Jagger. 2020. “Introduction: Forests, Trees and Poverty.” In Forest, Trees and the Eradication of Poverty: Potential and Limitations, edited by D. C. Miller, S. Mansourian, and C. Wildburger, 13–32. A Global Assessment Report. Vienna, Austria: International Union of Forest Research Organizations. 154 The Economic Case for Nature Monfreda, C., N. Ramankutty, and T. W. Hertel. 2009. “Global Agricultural Land Use Data for Climate Change Analysis.” In Economic Analysis of Land Use in Global Climate Change Policy, edited by T. W. Hertel, S. K. Rose, and R. S. J. Tol, 33–48. Oxon, UK: Routledge. Nallari, R., and B. Griffith. 2011. Understanding Growth and Poverty: Theory, Policy, and Empirics. Directions in Development: Poverty. Washington, DC: World Bank. https://openknowledge.worldbank.org/ handle/10986/2281. Noack, F., S. Wunder, A. Angelsen, and J. Börner. 2015. “Responses to Weather and Climate: A Cross-Section Analysis of Rural Incomes.” Policy Research Working Paper 7478, World Bank, Washington, DC. Nordhaus, W. D. 2007. “A Review of the Stern Review on the Economics of Climate Change.” Journal of Economic Literature 45 (3): 686–702. OECD (Organisation for Economic Co-operation and Development). 2006. “Decoupling: A Conceptual Overview.” OECD Papers 5/11. https://dx.doi.org/10.1787/oecd_papers-v5-art37-en. OECD (Organisation for Economic Co-operation and Development). 2017. The Political Economy of Biodiversity Policy Reform. Paris: OECD Publishing. https://doi.org/10.1787/9789264269545-en. ———. 2019. Agricultural Policy Monitoring and Evaluation 2019. Paris: OECD Publishing. https://doi. org/10.1787/39bfe6f3-en———. 2020a. “Towards Sustainable Land Use: Aligning Biodiversity, Climate and Food Policies.” OECD Publishing, Paris. https://doi.org/10.1787/3809b6a1-en. ———. 2020b. “Agricultural Policy Monitoring and Evaluation 2020.” OECD Publishing, Paris. https://doi. org/10.1787/928181a8-en. ———. 2020c. “A Comprehensive Overview of Global Biodiversity Finance.” OECD Publishing, Paris. OECD and IEA (Organisation for Economic Co-operation and Development and International Energy Agency). 2019. “Update on Recent Progress in Reform of Inefficient Fossil-Fuel Subsidies That Encourage Wasteful Consumption.” OECD, Paris. https://oecd.org/fossil-fuels/publication/OECD-IEA-G20-FossilFuel-Subsidies-Reform-Update-2019.pdf. Oldekop, J. A., K. R. Sims, B. K. Karna, M. J. Whittingham, and A. Agrawal. 2019. “Reductions in Deforestation and Poverty from Decentralized Forest Management in Nepal.” Nature Sustainability 2 (5): 421–28. Olivero, J., J. E. Fa, R. Real, A. L. Márquez, M. A. Farfán, J. Mario Vargas, D. Gaveau, et al. 2017. “Recent Loss of Closed Forests Is Associated with Ebola Virus Disease Outbreaks.” Scientific Reports 7: 14291. O’Neill, B. C., E. Kriegler, K. Riahi, K. L. Ebi, S. Hallegatte, T. R. Carter, R. Mathur, and D. P. van Vuuren. 2014. “A New Scenario Framework for Climate Change Research: The Concept of Shared Socioeconomic Pathways.” Climatic Change 122 (3): 387–400. Pardey, P. G., C. Chan-Kang, S. P. Dehmer, and J. M. Beddow. 2016. “Agricultural R&D Is on the Move.” Nature News 537 (7620): 301. Pardey, P. G., and J. Roseboom. 1989. ISNAR Agricultural Research Indicator Series: A Global Database on National Agricultural Research System. Cambridge University Press. Pardey, P. G., J. Roseboom, and J. Anderson. 1991. Agricultural Research Policy: International Quantitative Perspectives. Cambridge University Press. References 155 Pigato, M. 2019. Fiscal Policies for Development and Climate Action. Washington, DC: World Bank Group. Pimm, S. L., C. N. Jenkins, R. Abell, T. M. Brooks, J. L. Gittleman, L. N. Joppa, P. H. Raven, C. M. Roberts, and J. O. Sexton. 2014. “The Biodiversity of Species and Their Rates of Extinction, Distribution, and Protection.” Science 344: 6187. Polasky, S., B. Bryant, P. Hawthorne, J. A. Johnson, B. Keeler, and D. Pennington. 2015. “Inclusive Wealth as a Metric of Sustainable Development.” Annual Review of Environment and Resources 40: 445–66. Popp, A., K. Calvin, S. Fujimori, P. Havlik, F. Humpenöder, E. Stehfest, B. L. Bodirsky, et al. 2017. “Land-Use Futures in the Shared Socio-Economic Pathways.” Global Environmental Change 42: 331–45. Pretty, J., and Z. P. Bharucha. 2014. “Sustainable Intensification in Agricultural Systems.” Annals of Botany 114 (8): 1571–96. https://doi.org/10.1093/aob/mcu205. Ramankutty, N., A. T. Evan, C. Monfreda, and J. A. Foley. 2008. “Farming the Planet: 1. Geographic Distribution of Global Agricultural Lands in the Year 2000.” Global Biogeochemical Cycles 22 (1). http://www.agu. org/pubs/crossref/2008/2007GB002952.shtml. Ramankutty, N., and J. A. Foley. 1999. “Estimating Historical Changes in Global Land Cover: Croplands from 1700 to 1992.” Global Biogeochemical Cycles 13 (4): 997–1027. https://doi.org/10.1029/1999GB900046. Rockström, J., W. L. Steffen, K. Noone, Å. Persson, F. S. Chapin III, E. F. Lambin, T. M. Lenton, et al. 2009. “Planetary Boundaries: Exploring the Safe Operating Space for Humanity.” Ecology and Society 14 (2): 32. Ruesch, A., and H. K. Gibbs. 2008. “New IPCC Tier-1 Global Biomass Carbon Map for the Year 2000.” Carbon Dioxide Information Analysis Center, Oak Ridge National Laboratory, Oak Ridge, TN. http://cdiac. ornl. gov. Salati, E., A. Dall ‘Ollio, E. Matsui, and J. R. Gat. 1979. “Recycling of Water in the Amazon, Brazil: An Isotopic Study.” Water Resources Research 15: 1250–58. https://doi.org/10.1029/WR015i005p01250. Samir, K. C., and W. Lutz. 2017. “The Human Core of the Shared Socioeconomic Pathways: Population Scenarios by Age, Sex and Level of Education for all Countries to 2100.” Global Environmental Change 42: 181–92. http://doi.org/10.1016/j.gloenvcha.2014.06.004. Schneider, A., M. A. Friedl, and D. Potere. 2009. “A New Map of Global Urban Extent from MODIS Satellite Data.” Environmental Research Letters 4 (4): 044003. https://doi.org/10.1088/1748-9326/4/4/044003. Schneider, A., M. A. Friedl, and D. Potere. 2010. “Mapping Global Urban Areas Using MODIS 500-m Data: New Methods and Datasets Based on Urban Ecoregions.” Remote Sensing of Environment 114 (8): 1733–46. https://doi.org/10.1016/j.rse.2010.03.003. Sharp, R., H. T. Tallis, T. Ricketts, A. D. Guerry, S. A. Wood, R. Chaplin-Kramer, E. Nelson, et al. 2020. InVEST 3.8. 4. post2+ ug. g0932391 User’s Guide. Stanford, CA: The Natural Capital Project, Stanford University. Sharp, R., H. T. Tallis, T. Ricketts, A. D. Guerry, S. A. Wood, R. Chaplin-Kramer, E. Nelson, et al. 2018. InVEST 3.7.0.post22+ug.h3b687e57fad0 User’s Guide. Stanford, CA: The Natural Capital Project, Stanford University, University of Minnesota, The Nature Conservancy, and World Wildlife Fund. 156 The Economic Case for Nature Sims, K. R., and J. M. Alix-Garcia. 2017. “Parks versus PES: Evaluating Direct and Incentive-Based Land Conservation in Mexico.” Journal of Environmental Economics and Management 86: 8–28. Sohngen, B., C. Tennity, M. Hnytka, and K. Meeusen. 2009. “Global Forestry Data for the Economic Modelling of Land Use.” In Economic Analysis of Land Use in Global Climate Change Policy, edited by T. W. Hertel, S. K. Rose, and R. S. J. Tol, 49–71. Oxon, UK: Routledge. Steffen, W., K. Richardson, J. Rockström, S. E. Cornell, I. Fetzer, E. M. Bennett, R. Biggs, et al. 2015. “Planetary Boundaries: Guiding Human Development on a Changing Planet.” Science 347 (736): 1259855. Stern, N. H., S. Peters, V. Bakhshi, A. Bowen, C. Cameron, S. Catovsky, D. Crane, et al. 2006. Stern Review: The Economics of Climate Change, volume 30. Cambridge: Cambridge University Press. Stevenson, J. R., N. Villoria, D. Byerlee, T. Kelley, and M. Maredia. 2013. “Green Revolution Research Saved an Estimated 18 to 27 Million Hectares from Being Brought into Agricultural Production.” Proceedings of the National Academy of Sciences 110 (21): 8363–68. https://doi.org/10.1073/pnas.1208065110. Suh, S., J. A. Johnson, L. Tambjerg, S. Sim, S. Broeckx-Smith, W. Reyes, and R. Chaplin-Kramer. 2020. “Closing Yield Gap Is Crucial to Avoid Potential Surge in Global Carbon Emissions.” Global Environmental Change 63: 102100. Sumaila, U., V. Lam, F. Le Manach, W. Swartz, and D. Pauly. 2016. “Global Fisheries Subsidies: An Updated Estimate.” Marine Policy 69: 143–46. Tittensor, D. P., T. D. Eddy, H. K. Lotze, E. D. Galbraith, W. Cheung, M. Barange, J. L. Blanchard, et al. 2018. “A Protocol for the Intercomparison of Marine Fishery and Ecosystem Models: Fish-MIP v1.0.” Geoscientific Model Development 11 (4): 1421–42. Tol, R. S. 2008. “The Social Cost of Carbon: Trends, Outliers and Catastrophes.” Economics: The Open-Access, Open-Assessment E-Journal 2: 2008-25. van der Mensbrugghe, D. 2019. “The Environmental Impact and Sustainability Applied General Equilibrium (ENVISAGE) Model Version 10.01.” Center for Global Trade Analysis, Purdue University, West Lafayette, IN. https://mygeohub.org/groups/gtap/envisage-docs. Venter, O., W. F. Laurance, T. Iwamura, K. A. Wilson, R. A. Fuller, and H. P. Possingham. 2009. “Harnessing Carbon Payments to Protect Biodiversity.” Science 326 (5958): 1368. Waldron, A., V. Adams, J. Allan, A. Arnell, G. Asner, S. Atkinson, A. Baccini, et al. 2020. “Protecting 30 Percent of the Planet for Nature: Costs, Benefits and Economic Implications.” Campaign for Nature 49. http:// pure.iiasa.ac.at/id/eprint/16560/1/Waldron_Report_FINAL_sml.pdf. Walmsley, T., and T. Hertel. 2000. “China’s Accession to the WTO: Timing Is Everything.” GTAP Working Paper No. 13, Global Trade Analysis Project (GTAP), Department of Agricultural Economics, Purdue University, West Lafayette, IN. https://www.gtap.agecon.purdue.edu/resources/res display.asp?RecordID=403. WEF (World Economic Forum). 2020. The Global Risks Report 2020. Cologny, Switzerland: WEF. WEF (World Economic Forum). 2020. Nature Risk Rising: Why the Crisis Engulfing Nature Matters for Business and the Economy. Geneva: WEF. References 157 Weitzman, M. L. 2011. “Revisiting Fat-Tailed Uncertainty in the Economics of Climate Change.” Review of Environmental Economics and Policy 5 (2): 275–92. Woltjer, G. B., M. Kuiper, A. Kavallari, H. van Meijl, J. P. Powell, M. M. Rutten, L. J. Shutes, and A. A. Tabeau. 2014. The MAGNET Model: Module Description (No. 14-57). Den Haag, The Netherlands: LEI Wageningen UR. World Bank. 2006. Where Is the Wealth of Nations? Measuring Capital for the 21st Century. Washington, DC: World Bank. ———. 2011. The Changing Wealth of Nations: Measuring Sustainable Development in the New Millennium. Washington, DC: World Bank. ———. 2018a. “Poverty and Shared Prosperity 2018: Piecing Together the Poverty Puzzle.” World Bank, Washington, DC. ———. 2018b. “World Development Indicators.” http://datatopics.worldbank.org/ world-development-indicators. ———. 2021. Global Economic Prospects, January 2021. Washington, DC: World Bank. https://openknowledge. worldbank.org/handle/10986/34710. ———. Forthcoming. The Changing Wealth of Nations 2021: Managing Assets for the Future. Washington, DC: World Bank. World Bank Group. 2020. “Mobilizing Private Finance for Nature: A World Bank Group Paper on Private Finance for Biodiversity and Ecosystem Services.” World Bank, Washington, DC. ———. Forthcoming. “Unlocking Nature-Smart Development: An Approach Paper on Biodiversity and Ecosystem Services.” World Bank Group, Washington, DC. WWF (World Wildlife Fund). 2020. Living Planet Report 2020—Bending the Curve of Biodiversity Loss, edited by R. E. A. Almond, M. Grooten, and T. Petersen. Gland, Switzerland: WWF. The Economic Case for Nature is part of a series of papers by the World Bank that lays out the economic rationale for investing in nature and recognizes how economies rely on nature for services that are largely underpriced. This report presents a first-of-its-kind global integrated ecosystem-economy modelling exercise to assess economic policy responses to the global biodiversity crisis. Modeling the interaction between nature’s services and the global economy to 2030, the report points to a range and combination of policy scenarios available to reduce the impact of nature’s loss on economies. This modeling framework represents an important steppingstone towards ‘nature-smart’ decision-making, as it seeks to support policymakers who face complex tradeoffs involving the management of natural capital, and hence achieving growth that is resilient and inclusive.
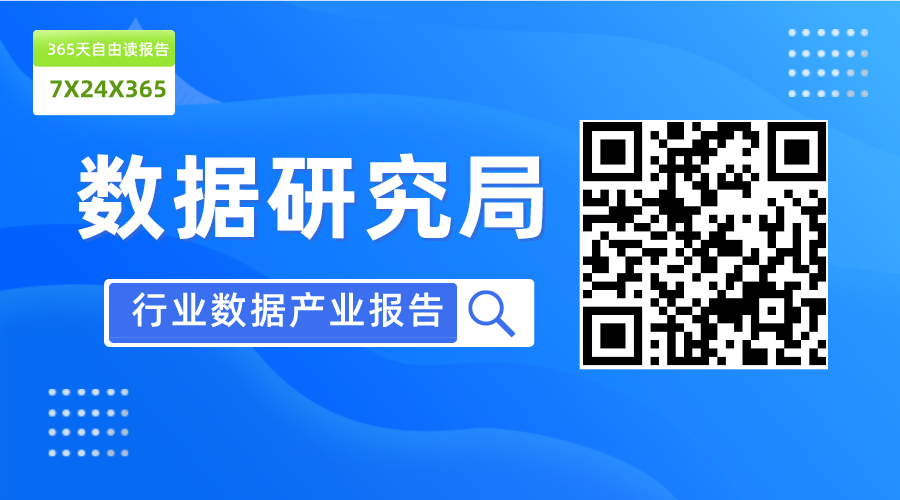
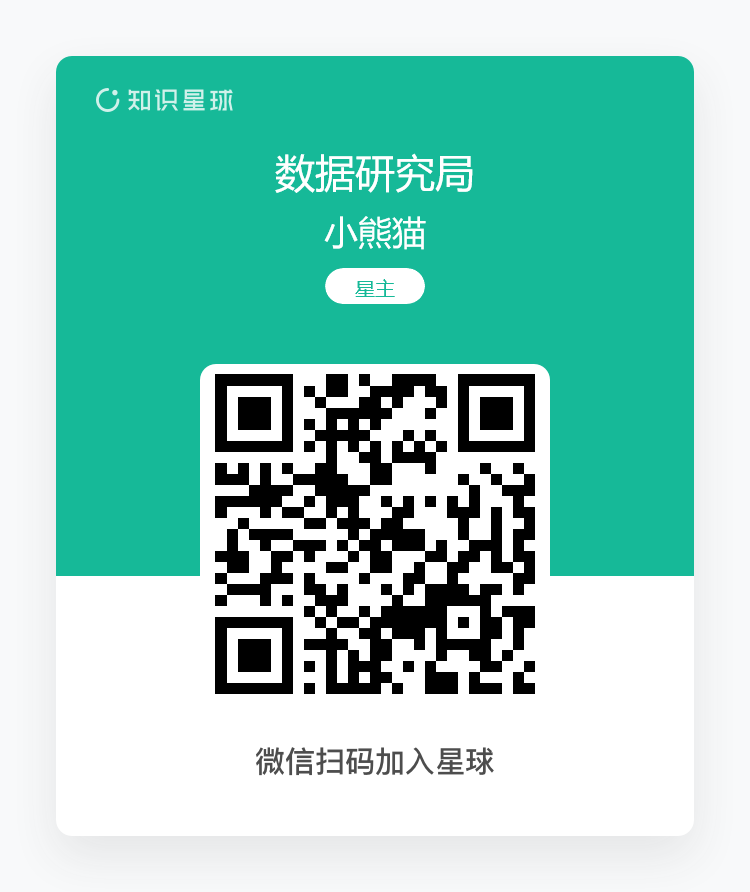