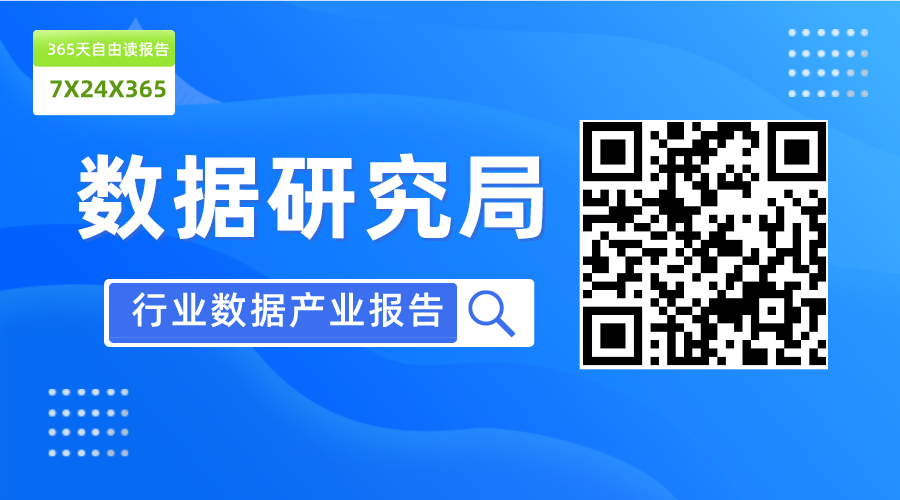
equitablegrowth.orgWorking paper seriesCovid-19 Businesses Reopenings and Consumer SpendingRaissa Dantas Jacob A. RobbinsMay 2021 https://equitablegrowth.org/working-papers/covid-19-businesses-reopenings-and-consumer-spending/© 2021 by Raissa Dantas and Jacob A. Robbins. All rights reserved. Short sections of text, not to exceed two paragraphs, may be quoted without explicit permission provided that full credit, including © notice, is given to the source.facebook.com/equitablegrowth @equitablegrowthequitablegrowth.org/feed info@equitablegrowth.org1156 15th St NW Suite 700 Washington DC 20005 202-545-6002COVID-19 BUSINESSES REOPENINGS AND CONSUMER SPENDING *Raissa Dantas† and Jacob A. Robbins‡April 14, 2021 Latest version here.Abstract This paper studies if Covid-19 retail and restaurant shutdowns and reopenings were responsible for the dramatic ‘V’ shaped pattern of consumer spending in the United States. We find reopening policies substantially increased spending for categories directly impacted by the laws: a 68.4 p.p. increase in non-essential in-store spending and a 16.7 p.p. increase in full-service indoor dining. For sectors not directly impacted — essential retail, limited-service restaurants, and online — we find a limited impact of reopenings. We estimate that retail reopenings are responsible for 34% of the total trough-to-peak recovery in spending, while restaurant reopenings are responsible for 15% of the recovery.Keywords: Covid-19, consumer spending, real-time data JEL Classification: E21*Many thanks to Daksh Joshi for excellent research assistance. We also thank Earnest Research for providing spending data.†University of Illinois at Chicago, Department of Economics, e-mail: rdanta2@uic.edu ‡University of Illinois at Chicago, Department of Economics, e-mail: jake.a.robbins@gmail.com1 INTRODUCTION1 IntroductionThe onset of Covid-19 initiated an unprecedented pattern of consumer spending in the United States. Figure 1 compares personal consumption expenditures in the Covid-19 recession and every other post-war recession, and displays a striking pattern: a decline of almost 20% by two months after the crisis, followed by a rapid recovery in the next two months, followed by a slower upward increase. Two obvious candidates for this striking pattern are (i) the fear of Covid-19, which drove many consumers to stay out of stores and (ii) stay at home (SAH) orders and retail shutdown policies, which forced them to. This paper studies the effect of these retail and restaurant shutdown policies on consumer spending, and attempts to disentangle the policy effect from the fear effect.1 We focus on the period after the initial weeks of crisis when states begin to allow businesses to reopen. We leverage the differential timing of states reopenings to estimate the effect of the reopenings on consumer spending.In making business shutdown and reopening decisions, state governments balance the public health benefits of slowing the spread of Covid versus the economic costs of business closures (Eichenbaum, Rebelo and Trabandt (2020)). This paper estimates the cost side of the equation for business shutdowns, which is useful for policymakers confronting the decision to reopen or re-close economies. Aside from the aggregate effects on spending, our detailed data allows us to pinpoint which businesses and industries are most affected by shutdowns. Policy makers can use this information in designing targeted aid packages for the businesses that are most affected by the lockdowns.Using detailed geographic data on consumer spending, we find retail reopenings caused large increases in non-essential in-store spending, with a point estimate of 68.4 p.p. y.o.y..2 Non-essential in-store spending can be thought of as the di-1It is possible that fear of Covid itself is endogenous to reopenings — in this case we will be distinguishing the effect of retail openings from the component of fear which is independent of state policies.2Because of the strong seasonality of retail spending, we use year over year spending growth throughout this paper, which compares spending in week w of 2020 with its corresponding week in 2019. A 10 p.p. increase in y.o.y. spending growth means an increase in spending of 10% of the level of spending a year ago.11 INTRODUCTIONFigure 1: Personal consumption expenditures during the Covid-19 recession, compared to previous recessions.Notes: PCE data from the BEA. This figure compares consumer spending in every post-war recession. Spending is normalized to 1 in the month before each recession.rect effects of the laws, since this was the only category of retail spending forced to close. Restaurant reopenings also had large direct effects, with a point estimate of 16.7 p.p. for indoor full-service dining. We find little evidence of indirect effects or spillovers on spending categories not directly affected by the law: results for essential retail, online spending, and limited-service restaurants are small and statistically insignificant.The overall macroeconomic effects on spending are a combination of direct and indirect effects. We estimate retail reopenings substantially increased total retail spending, with a point estimate of 9.5 p.p.. The dramatic results seen for nonessential in-store spending is tempered by the fact that this category represents only 8.0% of total retail spending — the categories considered as essential businesses included many of the largest retail in-store spending categories, such as groceries, general merchandise, and home improvement. We estimate reopenings caused total restaurant spending to increase by 8.6 p.p.. The larger increase in indoor full-service dining spending of 16.7 p.p. is offset by the small effects from limited-services21 INTRODUCTIONrestaurants, which remained open during the shutdowns. Our empirical results can be used to estimate to what extent businesses reopen-ings are responsible for the second leg of the “V” shaped recovery in aggregate retail sales. To do so, we estimate counterfactual retail and restaurant spending we would have seen if the states that locked down had never reopened. Our conclusion from this exercise is that businesses reopenings are not responsible for the spending growth seen in April and May, for two reasons: (i) it takes about a month after retail reopenings for spending to peak (ii) many states were still closed during that time. However, after May growth in spending is nearly all due to retail and restaurant reopenings. By September 1st, when all states were open, retail and restaurant reopenings were responsible for 34% and 15% of the trough-to-peak recovery, respectively. We thus find that the reopenings are substantially responsible for the overall recovery in consumer spending during the second half of 2020.We measure consumer spending with data from Earnest Research, a company that analyzes purchase data from a static panel of approximately 6mm de-identified US households. The data measures spending across credit cards, debit cards, and co-branded card paydowns, and is normalized to mitigate the effects of panel churn.3 It has broad coverage of industries, and is at the weekly level. Spending can be separated into online and in-store, and is available at the state level. We extensively benchmark the data, and find that retail and restaurant spending growth closely match totals from the Census Monthly Retail Trade Survey and the Quarterly Services Survey.Identification of the causal effects of retail reopenings is relative straightforward for non-essential in-store spending, due to a unique feature of the data: for states that reopened we can observe both their actual level of spending, and very accurately estimate their counterfactual level of spending had they remained closed, which is effectively zero, since stores are closed by law.4Our primary identification strategy for estimating other spending categories is an event study approach that exploits differential timing of states reopening. The3More specifically, Earnest leverages a "Consistent Shopper" normalization methodology, which measures spend across a subset of panelists who exhibit consistent spending behavior throughout the history of their panel.4See discussion in section 4.1.31 INTRODUCTIONidentifying assumption is that in the absence of reopening policies, states had similar trends in spending. A challenge to this assumption is that states that reopened earlier tended to have fewer Covid cases and better economic conditions than states that remained closed. In addition, the decision to reopen for many states was sometimes based upon keeping the number of Covid-19 cases under control.We address these concerns in several ways. First, our results are robust to eliminating “early openers” states from the sample, thus comparing states that may be more similar. Second, we analyze state reopening decisions, and find that many states did not necessarily wait until Covid cases were under control before reopening, and that many opened at local maxima in Covid cases. Finally, throughout the analysis we will flexibly control for both Covid-19 cases, hospitalizations, and deaths.Our results show a substantial part of the spending recovery in the second half of 2020 was due to business reopenings. This has important policy implications for the economic policy response to the pandemic recession. Fiscal spending, stimulus payments, or other policies to boost demand may have limited effects if key businesses remain closed. It may be more effective to boost spending through public health measures that allow businesses to reopen, or through investment in infrastructure that allows some non-essential stores to safely remain open.1.1 Literature ReviewThe closest work to ours is Chetty et al. (2020), which also studies (among many other policies) the impact of retail reopenings on consumer spending. To the best of our knowledge, there is no other paper studying the impact of reopenings in the United States. Nonetheless, Chetty et al. (2020) results are quite different from ours: they estimate little to no impact of reopenings on aggregate consumer spending. Because of these divergent results, it is worthwhile to highlights the differences in data and methodology. First, their paper only studies reopenings for 23 states (reopened before May 4); we study reopenings of all US states (DC included), with data through the end of 2020. Second, the policies studied are very different. Chetty et. al. examine spending relative to the “day a state began a reopening process”,41 INTRODUCTIONwhile we look specifically at the end of retail and restaurant shutdowns. Their estimate is then “an assessment of the average impact of typical re-opening efforts on aggregate activity”, while our estimates are specific to the retail and restaurant shutdowns, and our measure of economic activity include retail and restaurant spending, the categories most directly affected by the policies.Practically, the different policies studied mean we have a different definition of treated groups, and within a treated group we use different dates for when treatment begins. Chetty et. al. include in their treated groups three states that never had retail shutdowns or stay at home orders: North Dakota, Wyoming, and Nebraska. When we study retail shutdowns, we do not consider them treated. Within the treated groups, the date of treatment can vary widely between our papers. For instance, the authors consider Minnesota as a treated state, with a reopening date of April 27, but retail stores did not reopen until May 18, and restaurants indoor dining reopened on June 10. Therefore, by the “first day of reopening”, most businesses were still closed. For a complete list of the dates see Table A.1. A final difference is that their difference-in-difference regressions only compare spending from two weeks before to two weeks after reopenings. In our analysis we allow for longer post periods, and show the bulk of the increase in spending happens after the first two weeks.A more extensive literature looks at the impact of the initial stay at home orders on economic activity, with somewhat mixed results. Goolsbee and Syverson (2021) use cellular phone records data on customer visits and compare consumer behavior within commuting zones across different policy regimes. They find that lockdowns have modest effects on economic activity.5 Andersen et al. (2020) use transaction data from a large bank in Scandinavia to compare different policy regimes across Denmark and Sweden, and find that shutdown orders are responsible for a modest part of the decline of economic activity, and the virus itself is responsible for most of the economic contraction. In contrast to these null results, Coibion, Gorodnichenko and Weber (2020) and Alexander and Karger (2020) find that lockdowns are associated with substantial declines in consumer spending.A distinguishing feature of our paper is the unusually rich data, as well as5Goolsbee and Syverson (2021) data go through May 16th, 2020 and they also examine the effects of the initial reopenings, and also find small effects.52 DATAthe care we take to reweight and benchmark the data to match aggregate spending trends. Our data allows us to distinguish between essential and non-essential spending, in-store and online, full-service and fast-food restaurants, as well as 133 different industries. This enables us to pinpoint the exact sectors reopenings impact, as well as those it has no effect. We develop a procedure to reweight our data by industry and online share, which allows us to closely match data from the Census Month Retail Sales and the Census Quarterly Service Survey.2 Data2.1 SpendingSpending data is from Earnest Research, a company that analyzes purchase data from a static panel of approximately 6 million de-identified US households. The data captures spending on credit card, debit card, wire, and check transactions. It breaks down spending by (i) 133 detailed industries (ii) geographic level i.e., states, CBSAs, and cities (iii) channel of spending i.e., online, in-store, and store card. The frequency of the data is at the weekly level.One concern about using this data is that households in the Earnest panel may not keep updated bank and credit card information, and thus changes in spending will reflect measurement error rather than true data. To get around this problem, Earnest developed a “Consistent Shopper Panel”, a group of 2 million households that show consistent bank account and credit card use. This paper uses the Consistent Shopper Panel for all results.The main drawback of the data is that it is non-representative/nonrandom in several dimensions, which makes it difficult to measure total consumer spending. The data is non-representative in the following ways:1. Spending is measured for a sample of roughly 2,500 of the largest companies. The sample of firms is non-random across categories. Certain categories are very well captured by the data, while other sectors have few firms. For example, General Merchandise has excellent coverage, with most big firms such as62 DATAWalmart and Amazon included in the data, while for automobile sales there are only two firms, Tesla and Harley Davidson.62. Within each industry, the Earnest tends to sample firms that have a greater percentage of their sales online.3. Only large firms are included in the sample of firms, and thus the data does not capture spending on small businesses. The same firms are captured in the panel over time and are not resampled every period.4. The Earnest sample of households is non-representative; households must have linked bank and credit card accounts. Because of this, they tend to skew wealthier than the average US household. In addition, the sample is not randomly distributed geographically, with the sample overweight on the coasts and Texas.5. Cash transactions are not measured in the data.7In practice then the data measures consumer spending on large firms, and thus we will be estimating the effects of lockdowns on spending on large firms. In order to study the effect of the lockdowns on total spending, we would need to estimate the effect of lockdowns on small businesses. It seems likely that the effects of lockdowns would be larger in magnitude for small businesses, for two reasons: (i) small businesses are less likely to have online shopping, thus it is not possible for consumers to substitute away from in-store to online spending (ii) small businesses are more likely to go out of business due to lockdowns, which would lead to a greater decline in spending. Therefore, our estimates represent a lower-bound of the effects of reopenings on total spending.6For this reason, we exclude auto sales from all analysis below. 7Since cash transactions are not measured in the data, changes in card spending may not accurately reflect aggregate spending changes due to Covid-19. In fact, it would be expected that the virus would significantly curtail cash transactions, as (i) more purchases are made online (ii) consumers are wary about the spread of Covid-19 through contact with cash. To the extent that cash expenditures fall more than card spending, the totals in this paper would be a lower bound on the spending effects of Covid-19. According to the 2017 Diary of Consumer Payment Choice, consumers use credit and debit cards for 30.3% of payments, compared to using cash for 8.5% of payments.72 DATATable 1: Weekly retail spending by channel, 2019-present, $ millions.All 2019 2020 Wk 1-10 2020 Wk 11 2020 Wk 12-52Total retail 513.5 494.9478.8617.7543.1In-store352.5 361.5336.3469.7342.3% total 0.69 0.730.700.760.63Online130.8 103.4109.4117.9171.1% total 0.25 0.210.230.190.31Nonessential 94.9 99.388.175.591.4% total 0.18 0.200.180.120.17Essential418.6 395.6390.7542.2451.6% total 0.82 0.800.820.880.83N104 5210141Notes: Data from Earnest Research.Table 1 presents summary statistics for retail spending. Average weekly spending captured by Earnest on retail goods is $513.5 million, of which 69% is in-store and 25% is online.8 82% of spending is essential, while 18% is non-essential. Earnest has spending categories that roughly correspond to the industry breakdown of consumer spending from the Advance Monthly Sales for Retail and Food Services Survey (MARTS) conducted by the U.S. Census Bureau, and it will be seen that industry level data closely matches national trends. Table 2 compare 3-digit North American Industry Classification System (NAICS) industries for Earnest and the MARTS. Included in the table is the “coverage” of Earnest spending, which is a measure of how well Earnest data represents overall spending for the industry:CoverageI,t=Earnest CensusspendingI,t . spendingI,t(1)Also included is the correlation coefficient between Earnest m.o.m. growth and8The residual is on store cards, which is included in the in-store spending for our results.82 DATATable 2: Comparison of Census and Earnest dataCoverage (%) Corr m.o.m. m.o.m. adj Corr y.o.y. y.o.y. adjRetail trade ex autos0.620.910.930.380.80Food and drinking places0.460.850.950.730.98Vehicles and parts0.0410.670.630.640.65Home furnishing and furniture0.750.710.940.460.98Electronics0.760.620.92-0.330.94Building materials0.490.700.750.780.79Food and beverage0.740.940.940.910.91Health and personal care0.300.640.700.750.75Gas0.450.940.940.980.98Clothing and accessories0.680.760.950.970.98Sporting goods0.750.930.950.940.92General merchandise1.070.970.970.500.48Miscellaneous store retailers0.320.660.780.300.83Nonstore Retailer0.400.870.910.730.78Notes: Data from Earnest Research and Census Retail Sales. Coverage is defined as the ratio of aggregate Earnest spending to aggregate Census spending for the category. Correlations are between growth rates in Earnest and Census Retail Sales data.MARTS m.o.m. growth. For most industries, the correlation in month over month spending growth is fairly high.Figure 2 (a) compares m.o.m. percentage changes in retail spending excluding autos from Earnest to data from the MARTS, and shows a very tight correlation of spending growth, with ρ = .91. Although the series track each other closely, errors have increased somewhat since the pandemic began. There is a very strong seasonal component to retail sales, and a potential concern of the results of Figure 2 (a) is that they are driven by a common seasonal component, with little predictive power beyond seasonal effects. The y.o.y. correlation removes seasonable differences and92 DATAis shown in Figure 2 (b). The y.o.y. correlation is .38, although note this is for a relatively sample size of 24 y.o.y. observations.Figure 2: Comparison of Earnest and Census retail sales(a) m.o.m. growth(b) y.o.y. growthNotes: Data from Earnest Research and Census Monthly Retail Sales. Panel (a) compares m.o.m. growth rates of Earnest and Census retail sales. Panel (b) compares y.o.y. growth rates.While the overall y.o.y. correlation in retail spending is relatively low, many individual categories from Earnest do much better than the total. This motivates a procedure of reweighting the Earnest data to better match overall spending trends and adjust for Earnest’s non-representativeness. We will reweight spending growth on two dimensions: (i) share of spending by industry (ii) online spending share. We discuss both in turn.The first dimension we reweight is on industry spending share. Figure 3 (a) compares the share of retail spending for major NAICS categories for Earnest versus MARTS data. Earnest is overweight on grocery stores and general merchandise, and underweight on health and personal care stores, gas station, and non-store retailers.9 Although Earnest does have Amazon, the limited number of firms it covers means that it is underweight on nonstore retailers.9Earnest is overweight general merchandise and groceries because it has excellent coverage of the large chain stores such as Walmart and Target, as well as Whole Foods and Kroger.102 DATAFigure 3: Comparison of Earnest and Census(a) Share of spending(b) Online share of spendingNotes: Data from Earnest Research, Census Monthly Retail Sales, Census Quarterly Online Survey, Census Annual Retail Survey, Census Supplemental Annual Retail Survey. Panel (a) compares the share of retail spending for major NAICS categories for Earnest versus Census data. Panel (b) compares the share of spending that is online for Earnest compared with the Census quarterly Ecommerce report. The bar widths represent the difference between the share of retail spending in Earnest minus the share of retail spending in the Census.The second dimension we reweight is on the share of online spending. Earnest industries have a greater percentage of sales that are online compared with aggregate data. Figure 3 (b) compares the share of spending that is online for Earnest compared with the Census quarterly E-commerce report. About 25% of Earnest spending is online, compared with 11.3% for the Census data. Although the levels are off, spending growth is tightly correlated between the two sources. Figure A.1 compares the correlation of online spending growth for Earnest versus Census data, and finds a correlation of .99.We thus reweight data as follows. For each industry, we initialize total retail sales using the MARTS for January 2018. We then initialize spending for January 2018 between online and in-store spending for each using the shares for Q1 2018 from the Census E-commerce report. After January 2018, spending for online and112 DATAin-store are updated using growth rates from Earnest, and total spending formed by adding in-store and online.Figure 2 and Table 2, columns 3 and 5, show the reweighted results. The overall y.o.y. correlation of spending is .8, substantially higher than the unweighted data. Many individual categories also have higher y.o.y. correlations. The higher correlations show that when the Earnest data is put on an apples to apples basis with retail sales, it does a good job of matching aggregate spending trends. This will mean less measurement error and bias when estimating the effects of reopening on aggregate spending.2.2 Pandemic and aggregate spendingWe use Earnest data to measure the effect of the Covid-19 crisis on overall spending. Pre-crisis, retail sales were growing at a brisk clip of 4 p.p. y.o.y.. In mid-March (week 11) there was a “stocking up week”, with panicked consumers buying supplies of groceries and other essentials, leading to spending up 21 p.p. y.o.y.. After the stock-up week came the dramatic ”V” shape of spending. In the last week of March spending dropped to -22 p.p. y.o.y., before recovering slowly over the next three months. By July, spending returned roughly to its pre-pandemic trend.We further decompose spending between in-store and online. Figure 4 shows non-essential in-store spending fell by nearly 100% in the early weeks of the pandemic, then gradually increased to -15% y.o.y.. Some non-essential spending shifted from in-store to online, which by mid-April increased 135 p.p. y.o.y.. Essential in-store spending fell moderately after the initial surge from the stock-up week. Essential online saw a large and sustained increase, more than doubling by mid-April.Restaurant spending saw a more dramatic spending decline and slower recovery, with a nadir of -48 p.p. y.o.y.. Throughout the spring and summer spending recovered until the end of September, when spending was actually positive y.o.y.. However, increased Covid cases and colder weather drove spending back down to -16 % y.o.y. by year’s end.123 BUSINESS SHUTDOWN AND REOPENING POLICIESFigure 4: Pandemic spending by channel and essential vs non-essential(a)(b)(c)(d)Notes: Data from Earnest Research.3 Business shutdown and reopening policiesState governments pursued three main policies that affected consumer spending: stay at home orders, retail shutdowns, and restaurant shutdowns. Stay at home orders direct individuals to remain at home except for essential activities. Retail and restaurant shutdowns close non-essential businesses and in-house dining, respec-133 BUSINESS SHUTDOWN AND REOPENING POLICIES tively.We collect data on shutdown and reopening dates for retail and restaurants from official state government websites, executive orders, and press reports. Forty-five states had state-wide SAHs, 46 states had retail shutdowns10 and 50 states had restaurant shutdowns.11 Figure 5 shows considerable variation in reopening dates for retail and restaurant. The earliest states reopened in mid-April, most opened in May, and a few opened in June.Figure 5: Percentage of states under state-level ordersNotes: Data on stay at home and business closure laws were compiled from state government websites and executive orders. The figure presents the percentage of US states for a given date under stay at home, retail shutdown, and restaurant shutdown orders.3.1 SAH vs shutdown ordersWhile most existing literature focuses on SAH orders, this paper studies retail and restaurant shutdowns. We do so because a preliminary analysis of the raw data shows that spending responds dramatically to the end of a business shutdown even10The exceptions are Arkansas, Nebraska, North Dakota, South Dakota, Wyoming. 11The exception being South Dakota. We are counting DC as a state.143 BUSINESS SHUTDOWN AND REOPENING POLICIESwhen a SAH order is in place, while it does not respond when SAH orders end while business shutdowns are in place. In this sense, it seems that business shutdowns are the binding constraint on spending.To show this, we focus on the 8 states in which the retail stores reopened while the SAH order was still in effect. In all those states, a graphical analysis shows that non-essential in-store spending sharply increases after retail shops reopen. 12 Figure A.3 displays spending for these states, and shows that state government defacto allowed people to visit non-essential retail shops even under a SAH order. In the weeks between the retail reopening and the end of the SAH, these 8 states averaged 7.6 p.p. y.o.y. spending growth, compared to an average growth of .6 p.p. in the two weeks before reopening. In contrast, there were five states in which the SAH ended before the retail shutdown. For these states,13 the end of the SAH had no effect: average spending growth between the SAH end and the retail reopening was .7 p.p..For restaurants there is a similar story. Twenty two states ended SAH orders before opening restaurants for indoor dining. For 19 of these 22 states, restaurant spending did not increase with the end of SAH orders, but only increased after indoor dining was reinstated.143.2 Curbside pickup vs capacity restrictionsWhen lifting retail shutdown orders, states chose between two alternatives: limiting stores to curbside pickup only, or permitting in-store shopping but imposing capacity restrictions. Most states started with a more restrictive in-store capacity limit such as 20%-33% and gradually relaxed the restriction to 50%-75% occupancy. For example, Louisiana opened for curbside pickup on April 30, and then allowed 25% capacity on May 15th. The raw data suggests that spending does not noticeably respond to opening stores for curbside pickup, but does respond to allowing in-store shopping. For this reason, we focus empirical analysis on reopening laws that allow12The states are HI, ME, NC, NH, NM, PA, SC, WA. 13CA, DC, KY, MA, NY 14For the remaining three states (DC, PA, VT), spending does not respond to either SAH or restaurant orders being lifted.153 BUSINESS SHUTDOWN AND REOPENING POLICIESindoor shopping. In the Louisiana example, we define May 15th as the treatment date.153.3 Outdoor vs indoor diningWhen lifting restaurants shutdown orders, states chose between two alternatives: reopening for outdoor dining only or allowing some restricted indoor capacity. The pattern was for more restrictive capacity rules at first, followed by a gradual reduction of restrictions.16 For instance, Maryland opened outdoor dining on May 29 but only allowed indoor dining on June 12 at 50% capacity, then 75% capacity on September 21st. Graphical analysis of the raw data suggests that restaurant spending does not noticeably respond to allowing only outdoor dining, but does react to allowing indoor dining — for this reason we focus our empirical analysis on the causal effects of the capacity reopening. For instance, in the Maryland example, we define June 12 as the treatment date.173.4 Within state variationMost variation in retail and restaurant reopenings come from across state variation, however there is some in-state variation. Among the 46 states that closed retail business, seven states had within-state variation greater than one week (i.e., different counties reopened stores on dates at least one week apart). In these cases, though counties opened at different times, we define the treatment date as the earliest in-store capacity reopening date in the state. For example, in Kansas, most counties opened retail at 50 percent capacity on May 4, but Kansas City, Johnson County, Wyandotte opened on May 11 — we define May 4 as the treatment date. The only exception is Washington state. In WA most counties opened stores on May15Note that we are not defining our treatment variable based upon any observed spending growth — it is still based on legally when states reopen. However, in our preliminary analysis of what hypotheses to test, we focus on the particular reopening policies that seem to have substantial effects.16Eighteen states first opened for outdoor dining only, only later allowing for indoor dining. 17When summer is underway, a few states show responses when outdoor dining reopens while in-store is closed. However, this is infrequent. Only four states (i.e., MA, PA, NJ, and MS) see noticeable increases in spending when outdoor dining reopens, but indoor remains closed.163 BUSINESS SHUTDOWN AND REOPENING POLICIES12, but Seattle (King county) opened on June 5. Given that Earnest’s Washington data is concentrated on Seattle, we use June 5 as our treatment date.Among the 50 states with restaurant shutdowns, nine states had within-state variation greater than one week. In these cases, we use the earliest indoor capacity reopening date as treatment date. The only exceptions are Washington and California. For Washington state, we use the reopening date for King County (June 5) for the same reason as above. For California, we use the Los Angeles county restaurant reopening date (May 29) as there is a lot of within-state variation, and Earnest’s California data is concentrated in LA.3.5 Endogenous reopening decisionsA concern for our identification strategy is that states may have endogenously chosen reopening dates conditional on their levels of Covid cases. However, data comparing cases per capita and reopening dates shows that many states did not necessarily prioritize public health in the reopening decisions: in fact, 13 states reopened retail businesses when Covid cases were at a local maximum.18 In addition, during the first wave of Covid there were 25 states that were relatively unaffected by the virus and show no detectable trend break at reopening. For these 38 states endogenous reopenings are unlikely to be a problem.For 7 states, however, Covid cases are on a clear downward trend when states reopened, suggesting a potential endogenous reopening. In other 5 states, retail businesses were reopened only after covid cases had declined significantly and plateaued, also suggesting an endogenous reopening date. While we will flexibly control for Covid cases and deaths in our regressions, we will also explore robustness to results that exclude these twelve states.18Detailed state-by-state plots in Figure A.4.174 ESTIMATION STRATEGY4 Estimation strategy4.1 Non-essential in-storeFor non-essential in-store spending, a unique feature of the data is that we can observe the actual level of spending when states reopen, and very accurately estimate counterfactual spending levels if the states were still locked down, which should be quite close to zero by law. This fact motivates the following identification strategy.Let τ be weeks relative to the beginning of the retail reopening and s denote state. For a given state s, Y1sτ is spending τ weeks after retail reopening, Y0sτ is spending for the state if it were still locked down.We are interested in identifying the causal effect of retail reopenings on closed in-store spending. In other words,θsτ ≡ Y1sτ − Y0sτ τ > 0(2)After reopening, Y1sτ is observed. To estimate Y0sτ we use the strong restrictionfrom the fact that under lockdowns spending should be zero or close to zero, sincewhen a state is shutdown, people can’t shop at the stores. In practice, spendingis not exactly zero due to imperfections in the data and imperfect characterizationof non-essential businesses.19 As seen in the case studies, Figure 6, Illinois settlesat -91% y.o.y. spending, Massachusetts at -.94% y.o.y., and other states at similarlevels.We estimate Y0sτ by taking the sample mean of spending for the three weeksbefore reopening, Y0sτ = Y0s = (1/3)−1 t=−3Yst.Wethusformθsτ ≡ Ysτ − Y0s(3)19Non-essential in-store spending did not fall by exactly 100% for three reasons. First, there is a little cross-state variation on which businesses are considered essential and non-essential. Second, Earnest’s spending categories do not perfectly correspond to non-essential businesses. Therefore, it is possible that some essential businesses’ spending is classified as non-essential. Finally, it is possible that in-store pickup may be measured as in-store spending, and in-store pickup was sometimes allowed during the shutdowns.184 ESTIMATION STRATEGYTo identify the average treatment effect on the treated, this would beθτ = E[θsτ |Ds = 1](4)where Ds is a dummy variable that is 1 if the state reopened. We estimate the average treatment effect on the treated by taking an average across all states with shutdowns.4.2 Other spendingWhen studying total retail spending or other variables, it is no longer possible to use restrictions from laws to estimate counterfactual spending. Instead, we estimate counterfactuals from similar states that are still in lockdown, or that reopened previously.Figure 6 provides evidence that the effect of lifting lockdowns is not instantaneous, but rather accrues over about 5 weeks. This suggests that an event study rather than a simple difference in difference specification is appropriate.We estimate the following event study model5Spend y.o.y.sw = α + βτ 1{Week rel. reopen==τ } + γs + θw + υsw + sw (5)τ =−5 τ =−1where γs are state fixed effects, θw are week fixed effects, and υsw are a vector of state level controls. The excluded group of the event study is the week before the reopening. For our main specification, controls include the number of current Covid cases per capita,20 the number of cases squared, the growth rate of cases, and the growth rate squared. Given that the effects mostly stabilize after five weeks, the final lead includes all periods beyond the five weeks after treatment. Similarly, as most states shut their business for at least five weeks, the initial lag incorporates all periods prior to five weeks before treatment. Notably, as we focus on the later20There is a possibility that reopening retail and restaurants would lead to an increase in Covid cases. In this case, controlling for Covid cases would mean we are the effect of retail reopening on spending that is not caused by changes in Covid cases.195 RESULTSreopenings rather than the initial closings, we restrict the sample to weeks later than April 8, 2020, when all states that put shutdown orders in place were already closed.We also restrict the sample to exclude states that never locked down. We do so in order to better match counterfactual levels of spending growth. The trends of states that never locked down are very different than the states that reopen. In particular, the states that never locked down saw very large levels of spending growth during the period in which states were reopening, likely due to a decrease in the fear of Covid-19. Our final sample thus, for retail, has 1768 observations: 46 states over 39 weeks (week 14 of 2020 to week 52), minus 27 weeks for states that had a second shutdown.21 Similarly, for restaurants, our final sample has 1764 observations: 50 states over 39 weeks (week 14 of 2020 to week 52), minus 186 weeks for states that had a second shutdown.5 Results5.1 Non-essential in-store5.1.1 Case studiesThe patterns induced by retail reopenings are sufficiently stark to be directly viewed in the raw data. Figure 6 shows non-essential in-store spending for four states with retail shutdowns. After the beginning of the retail shutdowns, non-essential instore spending falls close to zero (down 100% y.o.y.). It stays close to zero until the shutdown ends, when it sharply increases. For non-essential in-store spending, 40/46 states that had shutdowns perfectly follow this pattern, i.e. spending sharply increases in the exact first week the state reopens.22 The fact that the spending increases sharply exactly at the end of the retail shutdown week suggests that the change in spending is not being driven by another unobserved factor, unless that unobserved factor is also changing sharply at the week of the end of retail shutdown.21New Mexico closed after November 13th (loss of 7 weeks), Hawaii after August 27th (loss of 19 weeks.22The other 6/46 states come quite close to following the pattern as well; either their spending increases a week early, or a week late.205 RESULTSFigure 6: Retail shutdown case studies(a) Illinois(b) Massachusetts(c) New York(d) FloridaNotes: Data from Earnest Research. Figures display non-essential in-store spending, businesses which were forced to close during the pandemic. Vertical lines denote various state policies. The red vertical lines indicate the beginning of the stay at home order ("sahb") and the end of the stay at home order ("sahe1"). When the stay at home order was lifted in parts, the end dates are indicated as: "sahe1" "sahe2". Gray vertical lines indicate retail reopenings at different in-store capacities. "ret25" indicates the date retailers were allowed to open at 25% in-store capacity, "ret50" at 50% capacity, and so on.215 RESULTS5.1.2 State shutdown groupsFigure 7 groups retail spending by the date that states reopened. We separate states into five groups: those that never locked down, early openers, mid openers, late openers, and very late openers.23 For each group, we calculated average spending across states.Figure 7: Retail spending by reopening group(a)(b)Notes: Data from Earnest Research. States are grouped into bins based on retail opening date, with groups roughly 11 days apart. Panel (a) displays the reweighted average y.o.y. spending across state reopening groups by calendar week. Panel (b) shows the spending across state reopening groups by week relative to retail reopening date.The pattern is fairly stark. During the initial phase of the shutdown all states saw dramatic declines in spending, even states that did not shut down. The declines of the different state groups are similar in magnitude. When shutdowns end, there is an immediate acceleration of spending, and later state groups begin to catch up with states that opened earlier.23Full list of states in each group see Table A.2.225 RESULTS5.1.3 Estimation resultsFigure 8 gives our estimate of the average treatment effect of reopenings, E[θsτ |Ds = 1]. The error bands are the estimated stand deviations of θsτ across the 46 states with lockdowns.Figure 8: Estimate of θτ .Notes: Figure displays estimate of the average treatment effect of reopenings on non-essential instore spending ( θτ from equation 4). Error bands are standard deviations of θsτ across states s.Figure 8 shows that lockdowns had a very large effect on non-essential in-store spending. The effects grow over time: on impact, reopenings had relatively small effects, but steadily grow before reaching a maximum about 5 weeks after reopening, with a point estimate of 68.4 percentage points y.o.y., significantly different than zero.We thus see that while lockdowns may not have been a binding constraint on non-essential in-store spending in early-March, they were a binding constraint later on as states reopened.235 RESULTS5.1.4 Second round of retail shutdownsTwo states passed second retail shutdown orders: Hawaii (from August 27th to September 23rd) and New Mexico (from Nov 13th-30th). Figure 9 displays nonessential in-store spending for both states. Both show declines in spending comparable in magnitude as the first retail shutdown. In terms of total retail spending, both states saw substantial declines: New Mexico saw a drop of 26 p.p. y.o.y., and Hawaii a decline of 25 p.p. y.o.y..Figure 9: States with second shutdowns: non-essential in-store spending(a) Hawaii(b) New MexicoNotes: Data from Earnest Research. Figures display non-essential in-store spending, businesses which were forced to close during the pandemic. Vertical lines denote various state policies. The red vertical lines indicate the beginning of the stay at home order ("sahb") and the end of the stay at home order ("sahe1"). When the stay at home order was lifted in parts, the end dates are indicated as: "sahe1" "sahe2". Gray vertical lines indicate retail reopenings at different in-store capacities. "ret25" indicates the date retailers were allowed to open at 25% in-store capacity, "ret50" at 50% capacity, and so on.5.2 Other Spending CategoriesAppendix Figure A.2 shows total retail spending for 4 representative states. The grey line indicates reopening dates. Unlike with essential in-store spending, there is no longer any clear pattern that jumps out from the raw data. We rely upon245 RESULTSthe event study regressions to estimate the effects of retail reopenings on aggregate spending. Figure 10 (a) presents event study regressions for total retail spending. For our preferred specification, the pattern is as follows: little evidence of a pretrend, followed by steadily increasing effects of reopenings on total retail spending. One week after a reopening total spending increases by 3.5 percentage points y.o.y., rising to a maximum of 9.5 p.p. after five weeks.Figure 10: Event study results and counterfactual analysis for total retail spending(a)(b)Notes: Panel (a) presents event study regression coefficients (equation 5) for total retail spendings. Panel (b) presents counterfactual estimates of total retail spending if states with retail shutdowns had remained closed and not reopened.Figure 11 show the effect of reopenings on non-essential and essential spending. Panel (a) shows that retail reopenings are associated with large increases in nonessential spending, with a maximum effect of 37.9 p.p. 5 weeks after reopening. Panel (b) shows results for essential spending and does not show a statistically significant effect of reopenings, although the point estimates are positive.255 RESULTSFigure 11: Retail event study results by spending type(a) Non-essential(b) EssentialNotes: Panels show event study regression coefficients (equation 5) for (a) non-essential retail (b) essential retail.Figure 12 shows results for in-store and online retail spending. Panel (a) shows that retail reopenings are associated with a large increase in total in-store spending, with a point estimate of 11.9 p.p. five weeks after the reopening. The results for online spending, on Panel (b), show that there is an increasing pre-trend in spending prior to reopenings; after reopenings the pre-trend does not continue, and if anything it reverses.265 RESULTSFigure 12: Retail event study results by spending channel(a) In-store(b) OnlineNotes: Panels show event study regression coefficients (equation 5) for (a) in-store retail (b) online retail.Overall, our estimates show that retail reopenings are associated with a substantial increase in total retail spending, driven mainly by non-essential and in-store spending. Figure 10 (b) presents a counterfactual estimation of what spending would be if all the states that closed retail stores had never reopened. For each state and week, the week-specific point estimate from the event study regressions are subtracted from actual spending if the state is in lockdown, and a weighted average of y.o.y. spending growth is taken across states. Retail reopenings are not responsible for the initial increase in spending since most states did not start opening until at least April 15th. Instead, the retail reopenings started having effects in May, June, and July, and contributed to spending almost reaching pre-Covid levels. By September 1st, all states had reopened, and the trough-to peak recovery in spending was 27 p.p.; had states remained closed, the recovery would only be 18 p.p.. Overall, retail reopenings are thus responsible for 34% of the total recovery in spending.275 RESULTS5.3 Restaurant spending 5.3.1 Case studiesFigure A.5 displays case studies for four states for trends in restaurant spending. Red vertical lines depict when restaurants open for outdoor dining, while the grey lines depict when they open for indoor dining.24We focus first on the trend for full-service spending. The pattern is that when states reopen for outdoor dining, there is often no immediate impact. In contrast, when indoor dining is reopened, there is a large jump in spending.25 This motivates our regression specification in which the main dependent variable represents when a state opens for indoor dining.For limited-service restaurants, there is no clear trend break when states reopen for indoor dining, suggesting a small overall effect. For overall spending, the pattern is a combination of limited and full-service: a noticeable increase when states reopen for indoor dining, but more modest than for full-service restaurants.5.3.2 State reopening groupsFigure 13 presents full-service restaurant in-store spending by reopening group. The pattern is a small pre-trend for most groups, followed by a sharp increase in spending when the state reopens for indoor dining.24There may be multiple lines, depicting different capacity restrictions. 25There are a few states that also show large responses to when outdoor dining is reopened, while in-store is closed. This is more apparent for states that reopened when summer was well underway. In Massachusetts, for example, when outdoor dining is opened there is a noticeable uptick in spending. Overall, there are only four states that see noticeable increases in spending when outdoor dining is reopened, but indoor remains closed: MA, PA, NJ, and MS.285 RESULTSFigure 13: Full-service in-store restaurant spending by reopening group(a) Calendar week(b) Week relative to reopeningNotes: Data from Earnest Research. States are grouped into bins based on restaurant reopening date, with groups roughly 11 days apart. Panel (a) displays the reweighted average restaurant y.o.y. spending across state reopening groups by calendar week. Panel (b) shows the reweighted average y.o.y. spending across state reopening groups by week relative to reopening date.Figure A.6 presents results for limited-service restaurants. Prior to reopening there is an upward trend in spending, with no obvious change in trend post reopening. The evidence suggests limited causal effect of restaurant reopenings on limited-service restaurants. Panel (b), which displays spending relative to reopening date, shows that different state reopening groups have different slopes in their trend-lines. This is evidence that, for this spending category, the parallel trends assumption may be violated. For this reason, the event study regression results for limited-service restaurants should be interpreted with caution.5.3.3 Event study regressionsFigure 14 (a) displays regression coefficients for total restaurant spending, showing that reopenings are associated with a 8.6 p.p. increase in total restaurant spending, with little evidence of a pre-trend.295 RESULTSFigure 14: Event study results and counterfactual analysis for restaurants(a) Results for total restaurant spending(b) Counterfactual spending under no reopeningNotes: Panel (a) presents event study regression coefficients (equation 5) for total restaurant spendings. Panel (b) presents counterfactual estimates of total restaurant spending if states with restaurant shutdowns had remained closed and not reopened.Figure 15 shows results for in-restaurant dining and online spending. Panel (a) shows that the total increase is driven by the in-restaurant component, with a maximum effect of 13 p.p.. Panel (b) shows that there is no evidence that reopenings increased online restaurant spending, and in fact the coefficients are generally negative, with a maximum estimated effect of -29.5 p.p. y.o.y.. Figure 16 display results for online delivery aggregators (like Grubhub and Uber Eats) and again shows negative coefficients, with a maximum effect of -15.3% y.o.y..305 RESULTSFigure 15: Restaurant event study results by spending channel(a) In-store(b) OnlineNotes: Panels show event study regression coefficients (equation 5) for (a) in restaurant spending (b) online restaurant spending.Figure 16: Online delivery aggregators(a)Notes: Panels show event study regression coefficients (equation 5) for online delivery aggregators (Grubhub, Uber Eats, etc).Figure 17 presents results for full and limited-service. Prior to reopening, Panel 315 RESULTS(a) shows that full-service restaurants had a declining trend in spending, but this is reversed upon reopening. Five weeks after reopening, the estimated coefficient is an increase of 16.7 p.p. in spending. In contrast, Panel (b) shows that for limitedservice restaurants, there is a pre-trend in spending, and no discernible break in trend after reopening.Figure 17: Restaurant event study results by spending category(a) Full-service restaurant spending(b) Limited-service restaurant spendingNotes: Panels show event study regression coefficients (equation 5) for (a) full service restaurant spending (b) limited service restaurant spending.Figure 14 (b) presents a counterfactual estimation of what spending would be if all the states that closed restaurants for indoor dining had never reopened. For each state and week, the week-specific point estimates from the event study regressions are subtracted from actual spending if the state is in lockdown, and a weighted average of y.o.y. spending growth is taken across states. The estimates show that restaurant reopening policies are not responsible for increases in restaurant spending in April and May, but start to have their effects in June, July, and August. By the end of September, when all policies have been in place, restaurant reopening policies are responsible for 15% of the total trough-to-peak recovery in spending.326 CONCLUSION5.4 RobustnessAppendix B displays results for three robustness exercises. First, we add additional controls for Covid-19 variables, flexibly controlling for current Covid-19 cases, cumulative cases, and deaths by interacting these variables with calendar time dummies. Second, we restrict the sample to exclude states that opened early, that may have differential trends in spending from other states. Finally, we restrict the sample to exclude states that may have endogenously opened their economy when Covid cases decreased, as discussed in section 3.5.The results of these robustness exercises reaffirm the conclusions from our main specifications, with only small to moderate changes in the main coefficients of interest.6 ConclusionIn this paper we provide the first evidence that the business reopening policies pursued in the wake of Covid-19 substantially increased consumer spending. The largest effects are seen for categories directly affected by the policies: a 68.4 p.p. increase in non-essential in-store spending, and a 16.7 p.p. increase in indoor full service restaurant spending. For categories not directly affected (essential spending, online spending, and limited-service restaurants) we find little evidence of any effect. The overall effects on spending are large and economically significant: an 9.5 p.p. increase in total retail and 8.6 p.p. increase in restaurant spending. The reopenings were responsible for a substantial portion of the recovery in retail spending in the second half of 2020. By September 1st, when all states were open, retail and restaurant reopenings were responsible for 34% and 15% of the trough-to-peak recovery, respectively.There are two main limitations to our results. First, retail and restaurant spending is only a subset of consumer spending, and we do not observe most service spending. It is possible that some spending substituted from goods to services due to retail reopenings, and thus our estimates for retail spending would not carry over to aggregate consumer spending. Second, even within retail spending we do336 CONCLUSION not have a random sample of spending — our sample captures spending on large businesses only, and from individuals who are wealthy enough to have both bank accounts and credit cards. To the extent that spending changed differentially between large and small businesses, and between wealthy and poor individuals, our estimates will not be representative.Our findings have important implications for governments facing tradeoffs between lockdowns and reopenings, and for optimal macroeconomic stabilization policies during a pandemic. Lockdowns cause substantial declines in consumer spending, which could lead to layoffs in the businesses most affected. From a stabilization perspective, stimulus payments, government spending, or other measures to boost aggregate demand may fail to work if businesses remain closed. It may be more effective to boost spending through public health measures that allow businesses to reopen, or through investment in infrastructure that allows some non-essential stores to safely remain open.34REFERENCESReferencesAlexander, Diane, and Ezra Karger. 2020. “Do stay-at-home orders cause people to stay at home? Effects of stay-at-home orders on consumer behavior.”Andersen, Asger Lau, Emil Toft Hansen, Niels Johannesen, and Adam Sheridan. 2020. “Pandemic, shutdown and consumer spending: Lessons from Scandinavian policy responses to COVID-19.” arXiv preprint arXiv:2005.04630.Chetty, Raj, John Friedman, Nathaniel Hendren, Michael Stepner, et al. 2020. “How did covid-19 and stabilization policies affect spending and employment? a new real-time economic tracker based on private sector data.” NBER working paper, , (w27431).Coibion, Olivier, Yuriy Gorodnichenko, and Michael Weber. 2020. “The cost of the covid-19 crisis: Lockdowns, macroeconomic expectations, and consumer spending.” National Bureau of Economic Research.Eichenbaum, Martin S, Sergio Rebelo, and Mathias Trabandt. 2020. “The macroeconomics of epidemics.” National Bureau of Economic Research.Goolsbee, Austan, and Chad Syverson. 2021. “Fear, lockdown, and diversion: Comparing drivers of pandemic economic decline 2020.” Journal of public economics, 193: 104311.35A APPENDIX FIGURESOnline Appendix for Covid-19 Businesses Reopenings and ConsumerSpendingRaissa Dantas and Jacob A. RobbinsA Appendix figuresFigure A.1: Comparison of Earnest and Quarterly Census online spending growth.Notes: The figure compares the correlation of online spending level and q.o.q growth for Earnest versus Census quarterly E-commerce report data. The q.o.q. correlation is .97.A.1A APPENDIX FIGURESFigure A.2: Total retail spending(a) Illinois(b) Massachusetts(c) New York(d) FloridaNotes: Data from Earnest Research. Figures display total retail spending, businesses which were forced to close during the pandemic. Vertical lines denote various state policies. The red vertical lines indicate the beginning of the stay at home order ("sahb") and the end of the stay at home order ("sahe1"). When the stay at home order was lifted in parts, the end dates are indicated as: "sahe1" "sahe2". Gray vertical lines indicate retail reopenings at different in-store capacities. "ret25" indicates the date retailers were allowed to open at 25% in-store capacity, "ret50" at 50% capacity, and so on.A.2A APPENDIX FIGURESFigure A.3: Non-essential retail in-store spending for states in which retail reopened before SAH end(a) Hawaii(b) Maine(c) New Hampshire(d) New Mexico(e) North Carolina(f) Pennsylvania(g) South Carolina(h) WashingtonNotes: Data from Earnest Research. Figures display non-essential in-store spending, businesses which were forced to close during the pandemic. Vertical lines denote various state policies. The red vertical lines indicate the beginning of the stay at home order ("sahb") and the end of the stay at home order ("sahe1"). When the stay at home order was lifted in parts, the end dates are indicatedas: "sahe1" "sahe2". Gray vertical lines indicaAte.3retail reopenings at different in-store capacities."ret25" indicates the date retailers were allowed to open at 25% in-store capacity, "ret50" at 50% capacity, and so on.A APPENDIX FIGURESFigure A.4: Non-essential retail in-store, Full service restaurant spending, and Covid cases per capita(a) Colorado(b) Iowa(c) Maryland(d) Kansas(e) Minnesota(f) Mississipi(g) Nebraska(h) Virginia(i) WisconsinNotes: Data from Earnest Research. Figures display non-essential in-store spending, full service restaurant spending, and Covid cases per capita for states that reopened retail or restaurants when Covid cases per capita were at a local maximum. Vertical lines denote various state policies. Green vertical lines indicate retail reopenings at different in-store capacities. "ret25" indicates the dateretailers were allowed to open at 25% in-store Aca.p4acity, "ret50" at 50% capacity, and so on. Bluevertical lines indicate restaurant reopenings at different indoor capacities. "r1_50" indicates the date restaurants were allowed to open at 50% in-house capacity, and so on. Orange vertical line represents capacity changes for a second wave of restaurant reopenings/closures. When restaurant and retail opened at the same date, we represent with a green vertical line.A APPENDIX FIGURESFigure A.5: Restaurant spending in different states(a) Illinois(b) Massachusetts(c) Maryland(d) FloridaNotes: Data from Earnest Research. Figures display in-store restaurant spending. Vertical lines denote various state policies. The red vertical lines indicate the beginning of the restaurant shutdown ("c1r") and the end of the restaurant shutdown ("r1out"). For "r1out" states allowed outdoor dining but indoor dining was closed. Gray vertical lines indicate restaurant reopenings at different in-house capacities. "r1_25" indicates the date restaurants were allowed to open at 25% in-house capacity, "r1_50" at 50% capacity, and so on. Blue vertical line represents capacity changes for a second wave of restaurant reopenings/closures.A.5A APPENDIX FIGURESFigure A.6: Limited-service in-store restaurant spending by reopening group(a) Calendar week(b) Week relative to reopeningNotes: Data from Earnest Research. States are grouped into bins based on restaurant reopening date, with groups roughly 11 days apart. Panel (a) displays the reweighted average restaurant y.o.y. spending across state reopening groups by calendar week. Panel (b) shows the reweighted average y.o.y. spending across state reopening groups by week relative to reopening date.A.6A APPENDIX FIGURESTable A.1: Reopening groupsState South Carolina Alaska Georgia Oklahoma Minnesota Mississippi Montana Tennessee Alabama Colorado Iowa Maine North Dakota Texas Utah Wyoming Florida Indiana Kansas Missouri Nebraska Ohio West Virginia“first day” 20-Apr 24-Apr 24-Apr 24-Apr 27-Apr 27-Apr 27-Apr 27-Apr 1-May 1-May 1-May 1-May 1-May 1-May 1-May 1-May 4-May 4-May 4-May 4-May 4-May 4-May 4-MayIn-store Retail 20-Apr 24-Apr 24-Apr 1-May 18-May 27-Apr 27-Apr 1-May 30-Apr 1-May 1-May 11-May1-May 1-May4-May 4-May 4-May 4-May12-May 4-MayIndoor dining 11-May 24-Apr 27-Apr 1-May 10-Jun 7-May 4-May 27-Apr 11-May 27-May 1-May 18-May 1-May 1-May 1-May 15-May 4-May 11-May 4-May 4-May 4-May 21-May 21-MayNotes: The table compares reopening dates across different approaches. The first column displays the "first day of reopening" used by Chetty et al. (2020). The second and third columns represent the dates of in-store retail and indoor dining reopenings, respectively.A.7A APPENDIX FIGURESTable A.2: Reopening groupsState Arkansas Nebraska North Dakota South Dakota Wyoming Alabama Alaska Colorado Georgia Idaho Iowa Louisiana Michigan Mississippi Montana Oklahoma South Carolina Tennessee Texas Utah Vermont Arizona California Florida Hawaii Indiana Kansas Maine Missouri Nevada New Hampshire North Carolina Ohio Rhode Island West Virginia Wisconsin Connecticut Delaware Kentucky Maryland Minnesota New Jersey New Mexico Oregon Pennsylvania Virginia District of Columbia Illinois Massachusetts New York WashingtonRetail Never locked Never locked Never locked Never locked Never locked Early Openers Early Openers Early Openers Early Openers Early Openers Early Openers Early Openers Early Openers Early Openers Early Openers Early Openers Early Openers Early Openers Early Openers Early Openers Early Openers Mid Openers Mid Openers Mid Openers Mid Openers Mid Openers Mid Openers Mid Openers Mid Openers Mid Openers Mid Openers Mid Openers Mid Openers Mid Openers Mid Openers Mid Openers Late Openers Late Openers Late Openers Late Openers Late Openers Late Openers Late Openers Late Openers Late Openers Late OpenersVery LAate.8OpenersVery Late Openers Very Late Openers Very Late Openers Very Late OpenersRestaurant Mid Openers Early Opener Early Opener Never locked Mid Openers Mid Openers Early Opener Late Openers Early Opener Mid Openers Early Opener Mid Openers Very Late Openers Mid Openers Early Opener Early Opener Early Opener Early Opener Early Opener Early Opener Very Late Openers Mid Openers Late Openers Early Opener Late Openers Mid Openers Early Opener Mid Openers Early Opener Mid Openers Mid Openers Mid Openers Mid Openers Mid Openers Early Opener Mid Openers Mid Openers Late Openers Mid Openers Late Openers Very Late Openers Very Late Openers Late Openers Mid Openers Very Late Openers Very Late Openers Late Openers Late Openers Very Late Openers Very Late Openers Very Late OpenersNotes: The table lists the reopenings groups based on the day of first capacity reopening for retailA APPENDIX FIGURES A.9B ROBUSTNESSB RobustnessFigure A.7: Retail event study robustness for a sample without early opener states(a) Total retail spending(b) Non-essential retail spending(c) Essential retail spending(d) In-store retail spending(e) Online retail spendingNotes: Panels show event study regression coefficients (equation 5) for retail spending by channeland category. Early openers states were excludAed..10B ROBUSTNESSFigure A.8: Retail event study robustness for a sample without states in which reopening decisions were potentially endogenous(a) Total retail spending(b) Non-essential retail spending(c) Essential retail spending(d) In-store retail spending(e) Online retail spendingNotes: Panels show event study regression coefficients (equation 5) for retail spending by channel and category. Early openers and states in which reopening decisions were potentially endogenous were excluded.A.11B ROBUSTNESSFigure A.9: Restaurant event study robustness for a sample without early opener states(a) Total restaurant spending(b) Total in-store restaurant spending(c) Total online restaurant spending(d) Online delivery aggregators spending(e) Full-service restaurant spending(f) Limited-service restaurant spendingNotes: Panels show event study regression coefficients (equation 5) for restaurant spending by channel and category. Early openers states were excluded.A.12B ROBUSTNESSFigure A.10: Restaurant event study robustness for a sample without states in which reopening decisions were potentially endogenous(a) Total restaurant spending(b) Total in-store restaurant spending(c) Total online restaurant spending(d) Online delivery aggregators spending(e) Full-service restaurant spending(f) Limited-service restaurant spendingNotes: Panels show event study regression coefficients (equation 5) for restaurant spending by channel and category. Early openers and states in which reopening decisions were potentially endogenous were excluded.A.13
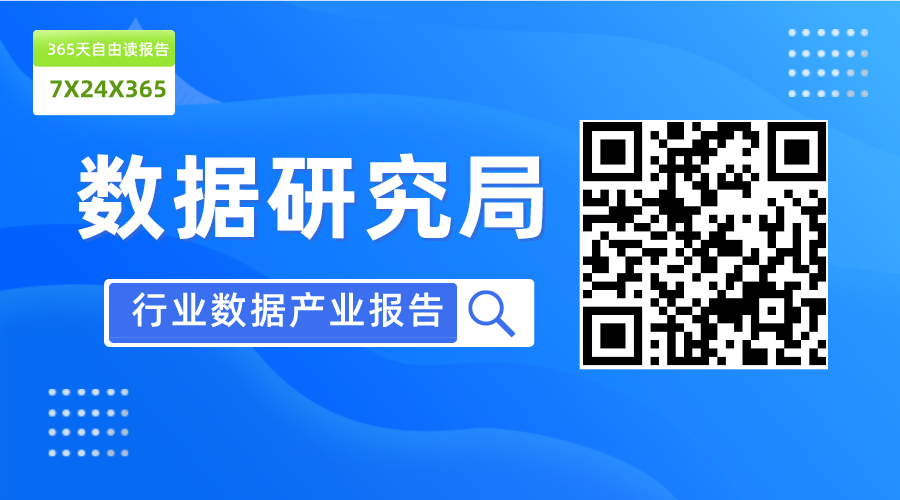
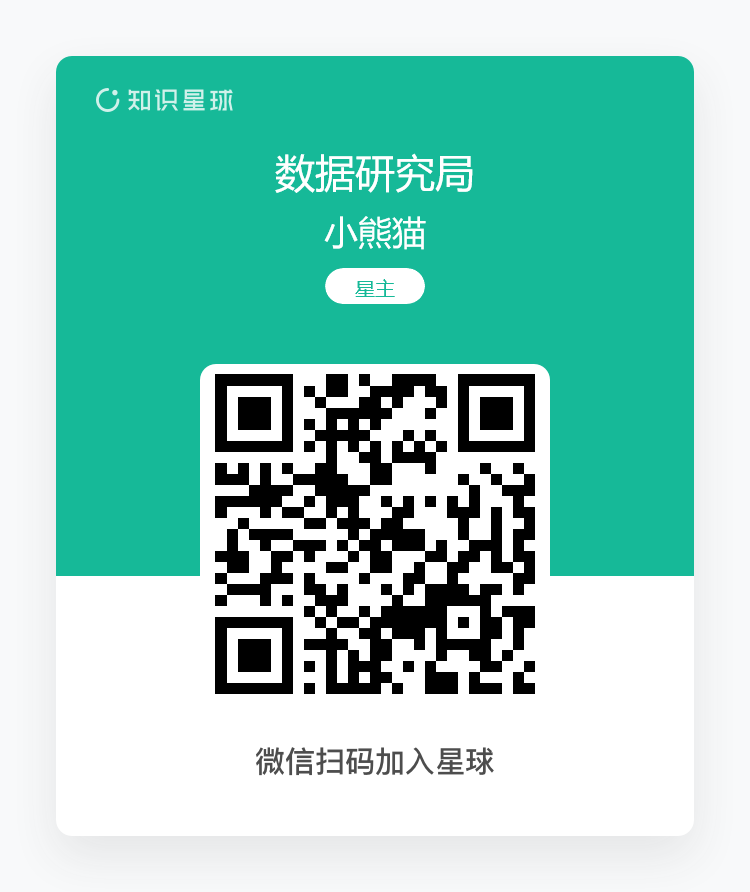