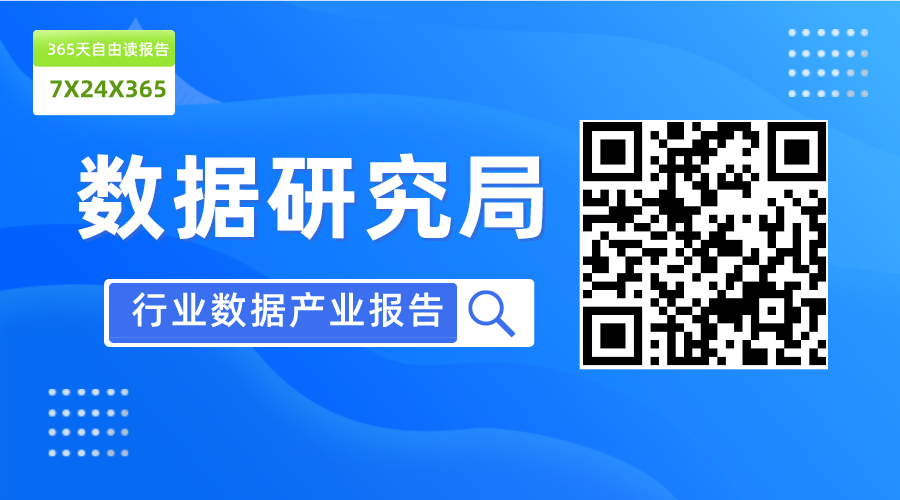
NBER WORKING PAPER SERIES THE GREAT UNEQUALIZER: INITIAL HEALTH EFFECTS OF COVID-19 IN THE UNITED STATES Marcella Alsan Amitabh Chandra Kosali I. Simon Working Paper 28958 http://www.nber.org/papers/w28958 NATIONAL BUREAU OF ECONOMIC RESEARCH 1050 Massachusetts Avenue Cambridge, MA 02138 June 2021 We thank Cong Gian, Joyce Kim, and Nikhil Shankar for excellent research assistance. Mary Bassett, David Cutler, Ryan Edwards, Ronald Lee, Maria Polyakova, and Jonathan Skinner provided helpful comments. We thank Erik Hurst, Heidi Williams, and Timothy Taylor for very useful comments and suggestions on a previous draft of the paper. The views expressed herein are those of the authors and do not necessarily reflect the views of the National Bureau of Economic Research. NBER working papers are circulated for discussion and comment purposes. They have not been peer-reviewed or been subject to the review by the NBER Board of Directors that accompanies official NBER publications. © 2021 by Marcella Alsan, Amitabh Chandra, and Kosali I. Simon. All rights reserved. Short sections of text, not to exceed two paragraphs, may be quoted without explicit permission provided that full credit, including © notice, is given to the source. The Great Unequalizer: Initial Health Effects of COVID-19 in the United States Marcella Alsan, Amitabh Chandra, and Kosali I. Simon NBER Working Paper No. 28958 June 2021 JEL No. I1,I14,I18,J1,J78,N0 ABSTRACT We measure inequities from the COVID-19 pandemic on mortality and hospitalizations in the United States during the early months of the outbreak. We discuss challenges in measuring health outcomes and health inequality, some of which are specific to COVID-19 and others that complicate attribution during most large health shocks. As in past epidemics, pre-existing biological and social vulnerabilities profoundly influenced the distribution of disease. In addition to the elderly, Hispanic, Black and Native American communities were disproportionately affected by the virus, particularly when assessed using the years of potential life lost metric. For example, Hispanic and Black Americans in 2020 saw 39.5 and 25 percent increases in excess mortality relative to trend, compared to a less than 15 percent increase for Whites; we find losses in potential years of life three to four times larger among Hispanic and Black compared to White Americans. Individual-level data from a commercially insured population show that otherwise similar Black and Hispanic enrollees were hospitalized due to COVID-19 at a higher rate than White enrollees. We provide a conceptual framework and initial empirical analysis which seek to shed light on contributors to pandemic-related health inequality, and suggest areas for future research. Marcella Alsan Kennedy School of Government Harvard University 79 John F. Kennedy St. Rubenstein Bldg R403 Cambridge, MA 02138 and NBER marcella_alsan@hks.harvard.edu Kosali I. Simon O’Neill School of Public and Environmental Affairs Indiana University 1315 East Tenth Street Bloomington, IN 47405-1701 and NBER simonkos@indiana.edu Amitabh Chandra John F. Kennedy School of Government Harvard University 79 JFK Street Cambridge, MA 02138 and NBER amitabh_chandra@harvard.edu Introduction When SARS-CoV-2 first appeared it was discussed as an equal opportunity pathogen: no one was immune, and therefore all potentially affected (Mein 2020, Krishnan et al. 2020). Early cases of COVID-19 disease among elites in entertainment, politics, and industry such as actor Tom Hanks, U.K. Prime Minister Boris Johnson and Morgan Stanley chief executive officer James Gorman gave credence to this view. Yet historical episodes of infectious disease are generally not experienced evenly across social strata. Rudolf Virchow, the founder of modern cellular pathology and a proponent of medicine as a social science, noted that "statistics will be our standard of measurement: we will weigh life for life and see where the dead lie thicker, among the workers or the privileged" (Virchow, 1848). Figure 1 demonstrates that for prominent novel infectious disease threats across the last two centuries in the United States, the dead indeed "lie thicker" among those less privileged. The upper-left panel shows that when cholera struck Boston in 1849, the mortality gap between native-born Bostonians and Irish immigrants and their children was about 50 percent larger than during a non-epidemic year like 1854. The upper-right panel illustrates how the age-adjusted mortality gap between White and non-White Americans rose during the influenza pandemic of 1918. The bottom-left panel displays how the HIV/AIDS pandemic worsened disparities in mortality between Black and White Americans. Finally, the lower-right panel shows that the age-adjusted difference in all-cause mortality rates between non-Hispanic White Americans and non-Hispanic Black Americans widened by over 80 percent during the first year of the COVID-19 pandemic.1 This paper discusses the initial health effects of COVID-19 in the United States. During 2020, the first year of the pandemic, COVID-19 was recorded as the underlying or contributing cause of 378,000 deaths nationwide (Ahmad et al., 2021). The impact of COVID-19 on health, however, extends beyond its direct toll on mortality. We begin by discussing the various ways by which COVID-19’s health effects have been measured, as well as the role pandemic-induced changes in the demand and supply sides of health care may have played in affecting mortality from causes other than the virus itself. Comparing the overall increase in mortality from 2019-20 to the number of recorded COVID-19 deaths in 2020 indicates that the pandemic has had substantial indirect effects on health. We next turn to examining inequality in the effect of COVID-19 on the health of different population groups. Infectious pathogens exploit both biological and social vulnerabilities, and the presentation of medical statistics can render gradients more or less conspicuous. Older age groups are particularly vulnerable to COVID-19, raising health risks for population groups with higher average ages, such as non-Hispanic Whites. Mortality rates conditional on age, however, are considerably higher for historically disadvantaged groups such as Black, Hispanic, and American Indians – especially due to their young average ages, these groups account for a disproportionate share of COVID-19 deaths. 1See Appendix Figure 1 for a comparison of 2019 and 2020 mortality rates including other races and ethnicities. 1 Mortality rate in Boston, 1854 (per 1000 population) Mortality rate in Boston, 1849 (per 1000 population) Panel A: Cholera (1849) Baseline Mortality 50 Pandemic Mortality 50 40 30 Δ = 15.97 40 Δ = 25.11 30 20 20 10 10 Native Irish Native Irish Cholera Other Causes Panel C: HIV-AIDS (1995) Baseline Mortality 4 Pandemic Mortality 4 Mortality rate, 1995 (per 1000 population) 3 Δ = 1.77 2 3 Δ = 2.26 2 1 White Black AIDS 1 White Other Causes Black Age-adjusted mortality rate, 2019 (per 1000 population) Age-adjusted mortality rate, 1917 (per 1000 population) Panel B: Influenza (1918) Baseline Mortality Pandemic Mortality 35 35 Age-adjusted mortality rate, 1918 (per 1000 population) 30 Δ = 9.32 25 30 Δ = 9.92 25 20 20 15 White Nonwhite 15 White Influenza/Pneumonia Other Causes Nonwhite Panel D: COVID-19 (2020) Baseline Mortality 11 Pandemic Mortality 11 10 9 Δ = 1.51 8 10 Δ = 2.78 9 8 Age-adjusted mortality rate, 2020 (per 1000 population) 7 7 6 Non-Hispanic White Non-Hispanic Black COVID-19 6 Non-Hispanic White Other Causes Non-Hispanic Black Mortality rate, 1985 (per 1000 population) Source: Panel A: Authors’ calculations from Shattuck (1846), Simonds (1850), Clark et al. (1850), Wright (1855), Curtis (1856), and Bushee (1899); Panel B: Authors’ calculations from Bureau of the Census (1919, 1920, 1922) and National Center for Health Statistics (2020a); Panel C: Authors’ calculations from National Center for Health Statistics (2020c); Panel D: Authors’ calculations from National Center for Health Statistics (2020a, 2020e, 2021a) and United States Census Bureau (2020a). Note: Figure reports mortality rate estimates by group during selected pandemics in U.S. history and for proximate non-pandemic years. Area shaded blue denotes recorded mortality from the pandemic disease (and in the case of influenza/pneumonia, includes seasonal baseline mortality patterns from influenza and/or pneumonia), while area shaded grey denotes mortality from other causes. See Data Appendix for computational details. Figure 1: Changes in Mortality for Different Groups during Pandemics, 1849-2020 2 The third section of the paper examines different explanations for why COVID-19 has had such unequal health effects, with a focus on racial and ethnic disparities. We provide a simple framework for organizing factors that contribute to the observed gradients, and consider whether they are driven by pre-existing differences in health risks and socioeconomic factors, or by differential impacts from the same across advantaged versus disadvantaged groups. We conclude by pointing out that the patterns of health inequality seen during the pandemic mirrored those that existed in the United States prior to COVID-19, and offer thoughts about how the evolution of these gradients and resilience for the next pandemic will depend on technology, health policy, and broader social policy. Measuring the Overall Initial Health Impact of COVID-19 The first case of SARS-CoV-2 infection in the United States was reported on January 20, 2020 (Holshue et al., 2020). From then to the end of the year, there were an additional 20.4 million confirmed infections nationwide (Centers for Disease Control and Prevention, 2021c). Of these cases, 378,000 resulted in death from COVID-19, the disease caused by SARS-CoV-2. By the measure of confirmed deaths from disease, the COVID-19 pandemic ranks among the deadliest in United States history, comparable to the 1918 influenza and HIV pandemics (Goldstein and Lee, 2020). While the health effects of the COVID-19 pandemic have clearly been significant, quantifying them is complex. A first challenge in measurement is data quality, which varies substantially by outcome of interest. Case reports of COVID-19 are often submitted with little information on patient demographics or their severity; 37.5 percent of cases in the Centers for Disease Control and Prevention’s (CDC) COVID-19 surveillance system are missing race/ethnicity data and 88.4 percent lack information on underlying health conditions.2 Although reports of "long COVID" indicate that the disease may have persistent health effects among some of those infected, systematic data on the morbidity impacts of COVID-19 are scarce (COMEBAC Study Group, 2021). In light of these data constraints, we focus on COVID-19’s effects on mortality, which is a key health outcome of interest and reported by law to the CDC (National Research Council, 2009).3 Quantifying the impact of COVID-19 on mortality still has challenges. While the number of deaths attributed to COVID-19 disease in 2020 indicates the effects have been substantial, this figure may be an underestimate of the pandemic’s mortality impacts. An estimated 3.4 million deaths occurred in the United States during 2020, an increase of 504,000 from the 2.9 million deaths which occurred during 2019. Evolving and variable clinical presentations alongside failures in testing, both of which characterized the early pandemic, may have resulted in deaths from COVID-19 go- 2See Appendix Table 1 for details. 3We also do not compute period life expectancy at birth. As discussed in Goldstein and Lee (2020), "in the context of epidemic mortality, life expectancy at birth is a misleading indicator, because it implicitly assumes the epidemic is experienced each year over and over again as a person gets older." Estimates of reductions in US life expectancy during 2020 are nevertheless striking: Andrasfay and Goldman (2021) project a 1.13 year decline in 2020 life expectancy at birth compared to a scenario without COVID-19. 3 ing unrecorded (Wu et al., 2020).4 Spillover effects of COVID-19 also increased pandemic-related mortality. We describe these factors below. Effects of COVID-19 on the Demand for Health Care Widespread avoidance of health care facilities early in the pandemic has been documented. The CDC’s Morbidity and Mortality Weekly Report from September 2020 estimated that 41 percent of U.S. adults had delayed or avoided medical care due to concerns about COVID-19, including 12 percent who had avoided urgent or emergency care (Czeisler et al., 2020b). In a nationally representative survey of 1337 adults conducted in July 2020 by researchers at Johns Hopkins, 29 percent of respondents who reported needing care forwent it due to fear of viral transmission, with 7 percent forgoing care due to financial repercussions of the pandemic (Anderson et al., 2021). Similar results are found in the Census Bureau’s Household Pulse Survey, a repeated crosssection of 1.8 million U.S. adults from the Bureau’s Master Address File (United States Census Bureau, 2020b). Of those surveyed between April and December 2020, 37 percent reported having delayed medical care over the previous four weeks due to the ongoing pandemic. The Pulse data indicate that delay of care followed the general contours of national COVID-19 prevalence, reaching a peak through the spring of 2020, declining in late summer, and plateauing at a lower level in early autumn before rising again. The share delaying care, however, topped 30 percent in every week the Pulse survey was fielded, and stood at 35 percent in December — nine months after the nation’s national emergency began. Effects of COVID-19 on the Supply of Health Care On the supply side, many non-emergency interventions were suspended due to the pandemic. Shortly after President Donald Trump declared the COVID-19 outbreak a national emergency in March 2020, the Centers for Medicare and Medicaid Services (CMS) recommended the cancellation or delay of most elective surgeries and non-emergency medical, surgical, and dental procedures (Centers for Medicare and Medicaid Services 2020). National and state-level policies sought to curtail patient volume in order to conserve scarce personal protective equipment, free up beds and personnel for COVID-19 patients, and reduce SARS-CoV-2 transmission. These changes may have elevated non-COVID-19 morbidity and mortality (Chen and McGeorge, 2020). The sharp reductions in volume and increased costs providers faced during the pandemic resulted in financial distress for many. The $187 billion in federal aid allocated to providers during the crisis exhibited little relationship to COVID-19 disease burden or hospital financial health, and failed to save many struggling providers even as well-resourced hospital networks, their losses cushioned with aid, engaged in a renewed wave of consolidation (Kakani et al. 2020, Abelson 2021). The closure of an estimated eight percent of physician practices and a record number of 4Symptomatic COVID-19 disease varies from mild to severe and can lead to death in a variety of ways. Pneumonia and respiratory failure are prominent final pathways, but cardiac conditions, embolic events and systemic inflammation are also possible (Gupta et al. 2020, Malas et al. 2020, Jose and Manuel 2020, Long et al. 2020). 4 rural hospitals, along with the higher prices and lower quality of care generally accompanying provider consolidation, may affect patient outcomes in the longer term (Physicians Foundation 2020, Basu et al. 2019, Gaynor 2018). On net, the pandemic’s impact on the supply and demand channels described above resulted in extraordinary declines in health care utilization. Non-COVID-19 medical admissions fell by 40 percent during the first wave of COVID-19 and remained depressed nearly a year later (Birkmeyer et al. 2020, Heist et al. 2021). The implementation of policies such as stay at home or business closure orders may have contributed to the fall in outpatient visits (Ziedan et al., 2020). While substitution to telemedicine partly offset the drop in in-person care, important preventive services such as vaccinations and screenings could not be shifted online and saw precipitous declines – one study using data on over 5 million individuals with employer-sponsored insurance found decreases of 22 percent in vaccinations among children aged 0-2, 67 percent in mammograms among women 46-64, and 70 percent in colonoscopies among individuals aged 46-64 (Patel et al. 2021, Whaley et al. 2020). The consequences of these delays in care will likely reverberate in the form of delayed diagnosis of non-communicable disease, preventable cases of infectious disease, and strain on providers, long after the pandemic ends. Additional Spillover Effects The COVID-19 health crisis is also an economic crisis. Based on prior recessions, Ruhm (2000) has noted that mortality tends to be procyclical. Since the opportunity cost of leisure declines, individuals have more time to exercise, prepare healthy food and, in non-pandemic times, seek medical care. The quality of healthcare, particularly in nursing homes, may also display cyclical fluctuations (Stevens et al., 2015). Declines in economic activity and mobility during the pandemic recession may have led to reductions in non COVID-19 deaths, compensating in part for the rise in mortality from infectious disease and delayed care. While there is some suggestive evidence of declines in air pollution and motor vehicle deaths from the early months of the pandemic, as well as a decrease in seasonal flu deaths resulting from reduced social interaction, averted deaths are likely small in number (Cicala et al. 2020, Centers for Disease Control and Prevention 2021a). Unlike in most recessions, protective measures taken by governments and individuals to limit disease transmission during the pandemic resulted in unprecedented levels of social isolation. Disruptions in daily routines, community life, and support systems were accompanied by a troubling increase in substance use disorder, with the CDC’s Morbidity and Mortality Weekly Report from August 2020 estimating that 13 percent of US adults had started or increased substance use to cope with the pandemic’s effects (Czeisler et al., 2020a). Following three years of relative stability, drug overdose deaths nationwide sharply rose beginning in April 2020, the first full month of the COVID-19 national emergency, and grew through October 2020 (National Center for Health Statistics, 2021b). The rise in substance abuse deaths concomitant with the pandemic suggests another avenue through which the COVID-19 crisis may have indirectly elevated mortality. 5 Excess Deaths: A Summary of COVID-19’s Mortality Effects The many ways through which the COVID-19 pandemic affected mortality renders precise attribution to any one cause challenging. Indeed, as individuals are often at risk for more than one type of death, some deaths recorded as due to COVID-19 disease would have occurred even in the absence of the pandemic (Gichangi and Vach, 2005). Figure 2 illustrates how the process of assessing the mortality toll of COVID-19 is complicated by the phenomena of substitution between different causes of death (competing risks), indirect deaths (spillovers), and averted deaths. The intractability of individually ascertaining the number of deaths resulting from each possible cause has motivated the use of "excess deaths" to capture the overall effect of the pandemic. Averted Deaths Competing Risks Deaths 0 Excess Deaths Deaths Inodf ependent COVID-19 DCeDaOitahVgsInDdo-us1ee9dto (Direct) Udnudeiatg(oDnCoirseOecVdt)IDD-e1a9ths Delays, CDoena(sSthtrpsailidlnoutvsee, rtason)CdaArveoidance Mortality Decrease Mortality Neutral Figure 2: A Taxonomy of COVID-19’s Impacts on Mortality Mortality Increase Excess deaths refer to differences between observed deaths in a particular time period and historical or expected deaths in a similar time period (National Center for Health Statistics, 2020d). As Figure 1 suggests, years in which the United States experienced an infectious disease epidemic demonstrate higher death rates than contemporaneous non-epidemic years, with the increase in deaths attributable to both mortality among infected individuals and a net increase in deaths from other causes. The sum of recorded deaths from the disease and the difference in deaths from all other causes compared to proximate time periods provides a summary statistic of the epidemic’s total effect on mortality. This number of excess deaths, which can be adjusted to account for preexisting mortality trends, is typically then divided by the size of the population to yield a rate of excess mortality. Following Polyakova et al. (2020, 2021), we estimate excess mortality as the deviation from a linear mortality trend. Figure 3 plots all-cause mortality rates among all Americans for each year from 2011 to 2020, using death data from the National Center for Health Statistics and population estimates from the American Community Survey (National Center for Health Statistics 2021a, United States Census Bureau 2020a). After declining throughout much of the 20th century, mortality rates in the United States have generally risen since 2010, in part due to the nation’s aging 6 Mortality rate (per 1000 population) 2011 2012 2013 2014 2015 2016 2017 2018 2019 2020 population. The number of deaths recorded in 2020, however, was far above the number expected based on prior trends. The deviation in the 2020 all-cause mortality rate from the 2011-19 trend was 1.39 deaths per 1000 population, or a 15.4 percent increase relative to trend. By comparison, the mortality rate from COVID-19 disease in 2020 was 1.08 per 1000 population, suggesting that spillovers contributed to elevated mortality during the pandemic. 11 10 Δ = 1.39 9 8 Year Figure 3: All-Cause Mortality Rates in the United States, 2011-2020 Source: Authors’ calculations from National Center for Health Statistics (2020a, 2020e, 2021a) and United States Census Bureau (2020a). Note: Figure plots mortality rates from all causes for the United States from 2011 through 2020. The difference in the 2020 mortality rate compared to the 2011-19 linear trendline is labeled. Mortality rates are not adjusted for age. Measuring COVID-19 Health Inequality The health consequences of the COVID-19 crisis affected Americans of all backgrounds, with over half of respondents in a Pew Research Center survey reporting that they knew someone personally who had died or been hospitalized due to the disease (Pew Research Center, 2020). The toll of the pandemic, however, fell most heavily on Black, American Indian, and Hispanic individuals, who disproportionately bore the total mortality burden of COVID-19 in at least two ways: they died almost always at greater rates, and they died at younger ages. We examine inequality in pandemicrelated mortality, with a focus on inequality by race and ethnicity, in the section below. 7 Excess Mortality by Race/Ethnicity and Age Having estimated all-cause excess mortality during the first year of the pandemic for all Americans, we replicate this approach by race/ethnicity and age. Panel A of Figure 4 reports excess mortality rates in 2020 for non-Hispanic White, non-Hispanic Black, Hispanic, and non-Hispanic Asian Americans within six age groups (0-44, 45-54, 55-64, 65-74, 75-84, and 85 and over).5 Panel A: Excess Mortality Rates 30 29.3 28.6 Excess mortality, 2020 (per 1000 population) 25 20 19.1 Ratio of excess mortality rate to White rate, 2020 15 10 5 0.1 0.4 0.2 -0.1 0 0-44 5 4.3 4 3 2.6 14.1 12.2 7.0 6.8 4.9 4.9 3.0 3.2 1.6 1.4 0.5 0.1 0.8 2.3 0.4 1.7 45-54 55-64 65-74 75-84 White Black Hispanic Asian Panel B: Ratio of Excess Mortality Rates to non-Hispanic Whites 3.2 2.8 4.0 3.8 3.1 3.0 2.9 2.5 9.8 85+ 2 1 [Ref] 1 * 0 0-44 1 [Ref] 1 [Ref] 0.2 1 [Ref] 0.5 1 [Ref] 0.7 1.5 1.5 1.0 1 [Ref] 0.5 45-54 55-64 65-74 75-84 85+ White Black Hispanic Asian Source: Authors’ calculations from National Center for Health Statistics (2020e, 2021a) and United States Census Bureau (2020a). Note: The rate ratio for non-Hispanic Whites is 1 by construction within each age group, and is shown for reference. The rate ratio for Asians age 0-44 is omitted as this group did not experience excess mortality. Figure 4: All-Cause Excess Mortality in 2020 by Race/Ethnicity and Age Group 5While American Indians and Alaska Natives (AIAN) experienced high rates of COVID-19 infection during the pandemic, we do not assess excess mortality among these populations due to known data quality issues (Yellow Horse and Huyser 2021, National Center for Health Statistics 2021c). Using data from a limited set of states, a CDC Morbidity and Mortality Weekly Report from December 2020 found that disparities in COVID-19 death rates between AIAN and non-Hispanic White individuals were large and particularly so at younger ages (Arrazola et al., 2020). 8 Pandemic all-cause excess mortality rises sharply with age, largely because age is the strongest single determinant of mortality from COVID-19 (Petrilli et al., 2020) and because avoided nonCOVID-19 healthcare is more likely detrimental to the oldest adults. Appendix Table 2 shows how Americans age 70 and above experience case fatality rates (rates of death conditional on diagnosis) about 200 times higher than those below age 40. Rates of excess mortality at any given age, however, vary sharply by race and ethnicity. Panel B of Figure 4 plots the ratio of non-Hispanic Black, Hispanic, and non-Hispanic Asian excess mortality rates to the non-Hispanic White excess mortality rate for each age group. The rate ratios presented in Panel B point to two dimensions of mortality disadvantage that Black and Hispanic Americans faced. First, the Black-White and Hispanic-White ratios are above one at every age, indicating that Blacks and Hispanics experienced elevated rates of excess death compared to non-Hispanic Whites. Indeed, when all age groups are pooled and excess mortality is computed for each race and ethnicity as a whole, it is evident that Black and Hispanic populations suffered the highest rates of excess death in 2020. Panel A of Figure 5 shows that Black Americans experienced excess mortality of 2.14 deaths per 1000 population in 2020, or a 25.0 percent increase in mortality relative to trend, while Hispanic Americans in 2020 saw excess mortality of 1.44 deaths per 1000 population in 2020, or a startling 39.5 percent rise relative to trend. Non-Hispanic Whites and Asians experienced increases in mortality of 1.29 and 0.58 deaths per 1000 population respectively, which are both increases of less than 15 percent relative to trend. As Panel B of Figure 5 indicates, these disparities widen further when excess mortality rates are age-adjusted to account for differences in age distributions between races and ethnicities — namely, the younger Hispanic and older non-Hispanic White age structures.6 Second, the ratios in Panel B of Figure 4 are strikingly higher at younger ages compared to older groups. The Black-White ratio in excess mortality is above four for the youngest age group of 0-44, and above three for all age groups through 65-74. Similarly, the Hispanic-White ratio in excess mortality is above 2.5 for all age groups from 0-44 to 65-74. By contrast, the Black-White and Hispanic-White excess mortality ratios for individuals age 85 and over are a comparatively low 1.5. The steep age gradient in excess mortality disparities indicates that the already high number of Black and Hispanic pandemic-related deaths disproportionately occurred among the young. Mortality rates, whether age-adjusted or unadjusted, do not differentiate between deaths at younger or older ages. Examining death rates alone therefore overlooks an important dimension of inequality: differences in the number of years individuals in each group would likely have lived had they not died due to pandemic-related causes. 6Age-adjusted statistics are computed by weighting deaths in different age groups among a given race or ethnicity in a manner that matches the share of each age group in the general population. Under age-adjustment, races and ethnicities with an age distribution younger than the general population have deaths at older ages weighted relatively more and deaths at younger ages weighted relatively less, whereas the converse would be true for races or ethnicities with an age distribution older than the general population. 9 Excess mortality rate in 2020, unadjusted for age (per 1000 population) Excess mortality rate in 2020, adjusted for age (per 1000 population) YPLL-65 (per 100,000 population age 0-64) Panel A: Crude Excess Mortality 2.14 2 1.29 1 1.44 0.58 0 White Black Hispanic Asian Panel B: Age-Adjusted Excess Mortality 2.16 2.12 2 1 0.84 0.48 0 White Black Hispanic Asian Panel C: Years of Potential Life Lost by Age 65 1400 1350.3 1200 1000 800 925.0 600 400 316.2 200 0 -200 White Black Hispanic -182.0 Asian Source: Authors’ calculations from National Center for Health Statistics (2020a, 2020e, 2021a) and United States Census Bureau (2020a). Note: Figure reports excess mortality rates unadjusted for age (Panel A), excess mortality rates adjusted for age (Panel B), and excess rates of years of potential life lost before age 65 (Panel C) during 2020. Figure 5: Measures of Racial and Ethnic Disparities in COVID-19 Pandemic Mortality Disparities in Lost Years of Life The concept of years of potential life lost, or YPLL, is used in the public health literature to quantify premature mortality (Gardner and Sanborn, 1990). As premature death is typically defined as a death occurring before age 65, the years of potential life lost for an individual who died prematurely is calculated by subtracting their age at death from 65, with those dying at age 65 or older assigned a years of potential life lost value of zero. The total years of potential life lost in a population is then computed by summing up the years of potential life lost among all individuals in the population who died early. This sum is usually normalized by dividing it by the number of individuals in the population under age 65. The aim of the years of potential life lost statistic is to measure life lost in years of life foregone as opposed to deaths incurred, providing a complementary measure to mortality rates. As with excess death rates, we estimate "excess" years of potential life lost during the COVID-19 pandemic as the deviation in 2020 from the 2011-19 linear trend. Panel C of Figure 5 plots excess years of potential life lost from all causes per 100,000 individuals under age 65 by race and ethnicity during 2020. Among all Americans, rates of YPLL rose by 12.8 percent in 2020 compared to trend. Similarly with mortality rates, however, this increase was again concentrated among Black and Hispanic Americans. Black rates of YPLL rose in 2020 by 1350.3 per 100,000 individuals under 65, or an increase of 19.5 percent relative to the 2011-19 trend. Among Hispanics, YPLL rose in 2020 by 925 per 100,000 individuals under 65, or 29.2 percent relative to trend. By comparison, YPLL rates among non-Hispanic Whites increased by 316.2 per 100,000 individuals under 65, or 8.4 percent, and among Asian Americans YPLL rates fell slightly relative to trend. Examining ratios of Black and Hispanic excess YPLL rates to the non-Hispanic White YPLL rate, in a similar manner to Figure 4, underscores the mortality disadvantage Blacks and Hispanics have faced during the pandemic. The Black-White ratio in excess years of potential life lost is 4.2, 10 whereas the Hispanic-White ratio in excess years of potential life lost is 2.9. In contrast, the BlackWhite ratio for age-adjusted excess mortality is 2.6, and the Hispanic-White age-adjusted excess mortality ratio is 2.5. The elevated YPLL ratios suggest that not only have Black and Hispanic Americans died at greater rates during the pandemic, but those who died on average had many more years of life left to live. Far from being an equal opportunity pathogen, SARS-CoV-2 has exposed societal cleavages between less privileged and more advantaged groups. Understanding the Unequal Health Effects of COVID-19 The COVID-19 pandemic affected some groups, particularly Black and Hispanic Americans, more than others. Why was this the case? Our goal in this section is twofold: to provide a simple framework for organizing the main factors that contribute to the observed disparities, and to present the results of a decomposition that examines the relative importance of some of these factors. A Simple Framework for Understanding COVID-19 Health Inequality We begin by focusing on deaths directly associated with COVID-19. Inequality in COVID-19 disease may be due to social determinants (such as differences in occupation, income or education), medical determinants (including differences in comorbidities, health care quality and insurance), and long-standing institutional features that perpetuate systemic racism and intergenerational poverty (Snowden and Graaf, 2020). These factors are not exhaustive nor are they mutually exclusive. They can, however, be mapped into an expanded model of disease transmission. The probability of death from COVID-19 is the product of the probability of SARS-CoV-2 infection and death from COVID-19 conditional on infection. Following standard epidemiological models, the probability of SARS-CoV-2 infection can be written as P(SARS-CoV-2 infection) = 1 − (1 − p)ni(1−mi), where i refers to an individual, p represents prevalence, n is the number of contacts, and m is the proportion of mitigated contacts (Halloran, 2009). All else equal, the probability of infection rises as prevalence in the community increases, and also increases if one has more contact with others. An individual’s infection probability, however, declines if more mitigating measures, such as mask-wearing, physical distancing, and vaccination, are taken. Prevalence (p) in an individual’s community and their number of contacts (n) can be affected by social inequality, population density, and local policies.7 Black and Hispanic populations both live in areas with higher COVID-19 prevalence and face higher costs of reducing their number of contacts. Black and Hispanic Americans live in larger households that are often multi-generational, and often have poor housing conditions (Pew Research Center, 2018). They are also more likely to be frontline workers who must work in-person despite the risk of infection, and cannot stop working or cut back on hours due to relatively low wealth levels or, particularly in the case of Hispanics, challenges in accessing federal benefits (Blau et al., 2020). 7Prevalence can be expressed as a function of the behavior of others around the individual (p(n−i(1−m−i))), which in turn is affected by the factors noted above. 11 Mitigation (m) can help offset the risk associated with labor or leisure-related interactions. However, ability to follow public health guidance depends on access to public health information, complementary tools such as vaccines and masks, and beliefs in the credibility of such information. A survey of approximately 5000 Americans conducted early in the pandemic showed that Black and Hispanic individuals, younger people, and men were less likely to have accurate information about COVID-19 transmission than other groups (Alsan et al., 2020a). Mitigation behavior by individuals during the pandemic has also been shaped by the dissemination of misinformation and features of the messenger, such as whether they are of the same race or ethnicity as the individual or whether they are an expert or peer (Simonov et al. 2020, Alsan et al. 2020b, Alsan and Eichmeyer 2021). Although communication with Black physicians has been shown to raise take-up of preventive health among Black Americans, just 4 percent of physicians in the United States are Black (Alsan et al., 2019). As discussed above, mortality during the COVID-19 pandemic can be conditional on infection (i.e. direct) or due to spillover effects. Access to quality health care is important in determining mortality, direct and indirect, from COVID-19. While higher-quality hospitals are associated with lower mortality rates, low-income, Black, and Hispanic Americans obtain health care from lower-quality facilities (Jha et al. 2011, Doyle et al. 2019, Chandra et al. 2020). Elevated COVID-19 caseloads in Black and Hispanic communities also contribute to non-COVID-19 excess deaths by reducing the ability of health care facilities to treat non-COVID-19 patients and causing individuals to avoid or delay necessary care due to fear of contagion. Black and Hispanic adults were also less likely at the start of the pandemic to be covered by health insurance, potentially contributing to care delays (Cohen et al., 2020). The distribution of pre-existing conditions differs by race and ethnicity, raising the probability of death conditional on COVID-19 infection and the inability to receive needed care for other chronic illnesses. Relative to non-Hispanic Whites, rates of diabetes are 1.7 and 1.8 times higher among Black and Hispanic populations (Centers for Disease Control and Prevention, 2020). Rates of obesity and hypertension are similarly elevated among Black and Hispanic individuals as well (Reeves and Smith, 2020). Diabetes, obesity and hypertension are conditions that increase the risk of death from COVID-19 (Centers for Disease Control and Prevention, 2021b). COVID-19 Health Inequality: A Decomposition The potential drivers of health disparities seen during the COVID-19 pandemic are manifold. Black and Hispanic Americans are disadvantaged socioeconomically relative to non-Hispanic Whites and tend to have a greater number of comorbidities that heighten the risk of severe COVID-19 disease. To what extent are racial and ethnic disparities in COVID-19 health outcomes driven by differences in these characteristics? Or, are Black, Hispanic, and non-Hispanic White individuals differentially impacted by COVID-19 even when they possess the same attributes? We aim to examine the relative importance of each of these factors through a decomposition exercise. As CDC datasets largely lack detailed comorbidity data or information on individuals who have not 12 contracted COVID-19, we obtain the necessary data from the Optum Clinformatics® Data Mart (CDM), a comprehensive commercial and Medicare Advantage claims database. In addition, we shift focus from the relatively rare outcome of mortality to COVID-19 hospitalizations. The Optum database includes approximately 67 million unique lives of all ages across 20072020 and is broadly representative geographically. We include adults age 21 or older who identify as either non-Hispanic White, non-Hispanic Black, or Hispanic, enrolled prior to July 2019, and filed a medical claim at least once during 2019 (thus avoiding cases where comorbidities have been undiagnosed and allowing us to have three quarters of data prior to the peak of the pandemic’s first wave). Our analysis sample includes all enrollees who were hospitalized for COVID-19 during the first three quarters of 2020, along with a five percent random sample of those not hospitalized for COVID-19 as a control group.8 Our final sample includes approximately 322,000 non-Hispanic White, 50,000 non-Hispanic Black, and 61,000 Hispanic enrollees. We first measure whether sampled enrollees were previously diagnosed with medical conditions that increase the risk of severe illness from COVID-19.9 We also extract social and demographic information including age, sex, average educational attainment in the enrollee’s Census block of residence, and Census division of residence. We conduct a "threefold" Oaxaca-Blinder decomposition that parcels out racial and ethnic differences in the likelihood of hospitalization due to COVID-19 into three components (Jann, 2008). The first component captures how much of the gap is from group differences in the predictors (the "endowments"). The second component captures the part due to differences in the coefficients (the "return to endowments"). The third component is the interaction between endowments and returns to endowments. Furthermore, we perform a "detailed" decomposition, as we track two sets of predictors: comorbidities, which are indicators for the medical conditions we measure, and sociodemographics.10 The decomposition is formulated from the viewpoint of Black or Hispanic enrollees. Although it is well-known that the reverse decomposition from the White perspective may provide different results, our approach is designed to produce two relevant counterfactuals. First, what is the expected change in hospitalization rates for Black and Hispanic Americans if the relevant minority group had the majority group’s predictor levels? Second, what would be the expected change if the minority group had the majority group’s coefficients?11 Table 1 presents the decomposition results. The left panel displays results for non-Hispanic Black vs. non-Hispanic White enrollees and the right panel displays results for Hispanic vs. nonHispanic White enrollees. The hospitalization rate for COVID-19 in our constructed sample is 8COVID-19 testing and results are not reliably included in claims data; moreover, testing was not necessarily evenly distributed across groups (Rubin-Miller et al., 2020). 9We extract information on hypertension, diabetes, obesity, cancer, heart disease, and chronic obstructive pulmonary disease based on diagnosis codes in claims filed between January 1 and December 31, 2019. 10For categorical variables, such as census block educational attainment and census division, we follow Yun (2005) to normalize the effects of categorical variables to avoid the issue of varying coefficients due to the choice of omitted group. 11We estimate with a linear probability model following Montenovo et al. (2020), but note that a logistic model provides similar results in terms of the importance of coefficients. See Data Appendix for additional data and methodological details. 13 7 percentage points higher for Black than White enrollees and 4.6 percentage points higher for Hispanic that White enrollees. For both groups, coefficients contribute much more to the overall difference than predictors. Perhaps surprisingly, the presence of comorbidities explains a much smaller share of the overall difference than the return to sociodemographics for both Black and Hispanic groups. Indeed, the return to sociodemographics is the single largest contributor to the overall gaps in hospitalization, accounting for 28.6 percent of the raw gap for Black compared to White enrollees and 56.7 percent of the raw gap for Hispanic compared to White enrollees.12 The results indicate that the same predictors exert a more damaging impact on Black and Hispanic sampled enrollees. As an example, Black or Hispanic male enrollees might be more likely than White male enrollees in similar situations to be engaged in work-related activities that place them at higher risk of contracting the disease and/or have less access to care and therefore present at a later stage, thus requiring hospitalization. In sum, the decomposition results suggest that the stark differences in COVID-19 health outcomes for Black and Hispanic Americans compared to non-Hispanic Whites cannot be attributed to a greater prevalence of pre-existing conditions, lower neighborhood levels of educational attainment, or (broad) geographical disadvantage alone. Rather, otherwise similar Black and Hispanic individuals, all of whom are insured in our sample, are hospitalized due to COVID-19 at a higher rate than non-Hispanic Whites. These results are specific to our sample and decomposition decisions we have taken, but they are consistent with the broader narrative that Black and Hispanic individuals face institutional disadvantages including inconsistent providers, lower-quality care, and systemic racism, that worsen their returns to similar endowments and contribute to COVID-19 health inequality. 12The difference in intercept contributes negatively to the differences in returns to sociodemographic variables. 14 Table 1: Decomposition of Race-Based Differentials in Likelihood of Hospitalization Due to COVID-19 Black vs. White Hispanic vs. White Overall Gap in Sample 0.070 0.046 Endowments Percent of Total Difference Returns to Endowments Percent of Total Difference Interaction Percent of Total Difference Number of Obs. Comorbidities Sociodemographics 0.011 16.2% 0.016 22.8% 0.009 12.4% 371,483 0.007 10.1% 0.020 28.6% 0.007 9.9% Comorbidities Sociodemographics 0.003 6.5% 0.012 24.9% 0.002 4.0% 382,425 0.001 1.9% 0.026 56.7% 0.003 6.1% Source: Authors’ calculations from Optum (2021). Notes: Table reports results from a threefold Oaxaca-Blinder decomposition from the perspective of the minority group. Sociodemographics includes age and age squared, gender, education dummies, and census division fixed effects. Effects of education and census division are normalized. Comorbidities represent a series of dummy variables for hypertension, diabetes, obesity, heart disease, cancer and chronic obstructive pulmonary disease. The difference in intercepts is included in the difference in returns to endowments of sociodemographics. 15 Conclusion This paper has drawn on history, medicine and economics to place the initial health effects of the current pandemic into broader context. That COVID-19 disproportionately killed the frail and disadvantaged could be expected based on viral dynamics, past epidemics and marked differences in the ability of individuals to protect their health during the crisis. Yet the heightened salience of these patterns, along with the stark mortality burden of the pandemic, may serve as a catalyst for change — in particular, for changing how Americans view the importance of public health and the social safety net (Rees-Jones et al., 2020). The initial health effects we outlined may have consequences for years to come. Most directly, a growing body of evidence suggests that a substantial proportion of individuals infected with COVID-19 suffer a range of long-term health consequences, including cognitive dysfunction, fatigue, and injury to the heart and lungs (COMEBAC Study Group 2021, del Rio et al. 2020). The pandemic’s long-term effects, however, will likely reach past those who contracted the disease and extend beyond health alone. Disruptions in screenings and routine health care may beget future premature morbidity and mortality from other communicable and non-communicable diseases (Chen and McGeorge, 2020). Scarring in utero exhibited in the influenza pandemic of 1918 and other epidemics of infectious disease may emerge with consequences for disability, educational attainment, and earnings (Almond, 2006). The disruptive effects of the COVID-19 crisis on education could widen inequality in income and health for future generations. These factors may exacerbate immediate economic disparities already experienced through labor markets as a consequence of the pandemic (Montenovo et al., 2020). With the introduction of recently approved vaccines against COVID-19 has come hope that the disruption the disease has wrought on health and society will soon cease. The same health gradients seen during the country’s descent into the pandemic, however, are likely to be observed as we emerge from it. Reports suggest that vaccination distribution by race and ethnicity has not been aligned with who has been affected most by the virus, placing vulnerable individuals at risk of adverse outcomes during a time in which SARS-CoV-2 continues to mutate (Ndugga et al. 2021). The medium and long-run health effects of COVID-19 — as well as the consequences of future novel infectious disease outbreaks which will assuredly emerge — will be shaped by how effectively and equitably policymakers respond to these formidable, yet not wholly unprecedented, challenges. 16 References Abelson, Reed (2021), “Buoyed by federal covid aid, big hospital chains buy up competitors.” The New York Times, https://www.nytimes.com/2021/05/21/health/covid-bailout-hospital-merger. html. Ahmad, Farida B., Jodi A. Cisewski, Arialdi Miniño, and Robert N. Anderson (2021), “Provisional mortality data — united states, 2020.” Morbidity and Mortality Weekly Report, 70, 519–522. Almond, Douglas (2006), “Is the 1918 influenza pandemic over? long-term effects of in utero influenza exposure in the post-1940 u.s. population.” American Economic Review, 114, 672–712. Alsan, Marcella and Sarah Eichmeyer (2021), “Experimental evidence on the effectiveness of nonexperts for improving vaccine demand.” National Bureau of Economic Research, 1–27. Alsan, Marcella, Owen Garrick, and Grant Graziani (2019), “Does diversity matter for health? experimental evidence from oakland.” American Economic Review, 109, 4071–4111. Alsan, Marcella, Stefanie Stantcheva, David Yang, and David Cutler (2020a), “Disparities in coronavirus 2019 reported incidence, knowledge, and behavior among us adults.” JAMA network open, 3, e2012403–e2012403. Alsan, Marcella M., Fatima Cody Stanford, Abhijit Banerjee, Emily Breza, Arun G. Chandrasekhar, Sarah Eichmeyer, Paul Goldsmith-Pinkham, Lucy Obgu-Nwobodo, Benjamin A. Olken, Carlos Torres, Anirudh Sankar, Pierre-Luc Vautrey, and Esther Duflo (2020b), “Comparison of knowledge and information seeking behavior after general covid-19 public health messages and messages tailored for black and latinx communities: A randomized controlled trial.” Annals of Internal Medicine. Anderson, Kelly E., Emma E. McGinty, Rachel Presskreischer, and Colleen L. Barry (2021), “Reports of forgone medical care among us adults during the initial phase of the covid-19 pandemic.” JAMA Network Open, 4. Andrasfay, Theresa. and Noreen Goldman (2021), “Reductions in 2020 us life expectancy due to covid-19 and the disproportionate impact on the black and latino populations.” Proceedings of the National Academy of Sciences of the United States of America, 118, e2014746118. Arrazola, Jessica, Matthew M. Masiello, Adrian E. Dominguez, Sujata Joshi, Amy Poel, Crisandra M. Wilkie, Jonathan M. Bressler, Joseph McLaughlin, Jennifer Kraszewski, Kenneth K. Komatsu, Xandy Peterson Pompa, Megan Jespersen, Gillian Richardson, Nicholas Lehnertz, Pamela LeMaster, Britney Rust, Alison Keyser Metobo, Brooke Doman, David Casey, Jessica Kumar, Alyssa L. Rowell, Tracy K. Miller, Mike Mannell, Ozair Naqvi, Aaron M. Wendelboe, Richard Leman, Joshua L. Clayton, Bree Barbeau, Samantha K. Rice, Victoria Warren-Mears, Abigail EchoHawk, Andria Apostolou, and Michael Landen (2020), “Covid-19 mortality among american 17 indian and alaska native persons — 14 states, january–june 2020.” Morbidity and Mortality Weekly Report, 69, 1853–1856. Basu, Sanjay, Seth A. Berkowitz, Robert L. Phillips, Asaf Bitton, Bruce E. Landon, and Russell S. Phillips (2019), “Association of primary care physician supply with population mortality in the united states, 2005-2015.” JAMA Internal Medicine. Birkmeyer, John D., Amber Barnato, Nancy Birkmeyer, Robert Bessler, and Jonathan Skinner (2020), “The impact of the covid-19 pandemic on hospital admissions in the united states.” Health Affairs, 39. Blau, Francine, Josefine Koebe, and Pamela A. Meyerhofer (2020), “Who are the essential and frontline workers?” National Bureau of Economic Research, 1–10. Bureau of the Census (1919), “Mortality statistics 1917, eighteenth annual report.” Bureau of the Census (1920), “Mortality statistics 1918, nineteenth annual report.” Bureau of the Census (1922), “1920 census: Volume 2. population, general report, and analytical tables, chapter 3.” Bushee, Frederick A. (1899), “The growth of the population of boston.” Publications of the American Statistical Association, 6, 239–274. Centers for Disease Control and Prevention (2020), “National diabetes statistics report, 2020.” Atlanta, GA: Centers for Disease Control and Prevention, US Department of Health and Human Services. Centers for Disease Control and Prevention (2021a), “2020-2021 influenza season for week 21, ending may 29, 2021.” https://www.cdc.gov/flu/weekly/index.htm. Centers for Disease Control and Prevention (2021b), “Science brief: Evidence used to update the list of underlying medical conditions that increase a person’s risk of severe illness from covid-19.” https://www.cdc.gov/coronavirus/2019-ncov/science/science-briefs/ underlying-evidence-table.html. Centers for Disease Control and Prevention (2021c), “Trends in number of covid-19 cases and deaths in the us reported to cdc, by state/territory.” https://covid.cdc.gov/covid-data-tracker/ #trends_dailytrendscases. Accessed 5/20/2021. Centers for Disease Control and Prevention, COVID-19 Response (2020), “Covid-19 case surveillance public data access, summary, and limitations (version date: December 31, 2020).” https://data.cdc.gov/Case-Surveillance/COVID-19-Case-Surveillance-Public-UseData/vbim-akqf. Accessed 1/04/2021. 18 Centers for Medicare and Medicaid Services (2020), “Cms releases recommendations on adult elective surgeries, non-essential medical, surgical, and dental procedures during covid-19 response.” https://www.cms.gov/newsroom/press-releases/cms-releases-recommendationsadult-elective-surgeries-non-essential-medical-surgical-and-dental. Chandra, Amitabh, Pragya Kakani, and Adam Sacarny (2020), “Hospital allocation and racial disparities in health care.” National Bureau of Economic Research, 1–33. Chen, Julius and Rebecca McGeorge (2020), “Spillover effects of the covid-19 pandemic could drive long-term health consequences for non-covid-19 patients.” Health Affairs Blog. Cicala, Steve, Stephen P. Holland, Erin T. Mansur, Nicholas Z. Muller, and Andrew J. Yates (2020), “Expected health effects of reduced air pollution from covid-19 social distancing.” National Bureau of Economic Research, 1–17. Clark, Henry G., Charles E. Buckingham, John C. Dalton, and Henry W. Williams (1850), “Report on the cholera in boston, in 1849.” Cohen, Robin A, Amy E Cha, Michael E Martinez, and Emily P Terlizzi (2020), “Health insurance coverage: Early release of estimates from the national health interview survey, 2019.” National Health Interview Survey Early Release Program, 1–18. COMEBAC Study Group (2021), “Four-month clinical status of a cohort of patients after hospitalization for covid-19.” Journal of the American Medical Association, 325, 1525–1534. Curtis, Josiah (1856), “Report of the joint special committee on the census of boston, may, 1855, including the report of the censors, with analytical and sanitary observations.” Czeisler, Mark E., Rashon I. Lane, Emiko Petrosky, Joshua F. Wiley, Aleta Christensen, Rashid Njai, Matthew D. Weaver, Rebecca Robbins, Elise R. Facer-Childs, Laura K. Barger, Charles A. Czeisler, Mark E. Howard, and Shantha M.W. Rajaratnam (2020a), “Mental health, substance use, and suicidal ideation during the covid-19 pandemic — united states, june 24–30, 2020.” Morbidity and Mortality Weekly Report, 69, 1049–1057. Czeisler, Mark E., Kristy Marynak, Kristie E.N. Clarke, Zainab Salah, Iju Shakya, JoAnn M. Thierry, Nida Ali, Hannah McMillan, Joshua F. Wiley, Matthew D. Weaver, Charles A. Czeisler, Shantha M.W. Rajaratnam, and Mark E. Howard (2020b), “Delay or avoidance of medical care because of covid-19–related concerns — united states, june 2020.” Morbidity and Mortality Weekly Report, 69, 1250–1257. del Rio, Carlos, Lauren F. Collins, and Preeti Malani (2020), “Long-term health consequences of covid-19.” Journal of the American Medical Association, 324, 1723–1724. Doyle, Joseph J., John A. Graves, and Jonathan Gruber (2019), “Evaluating measures of hospital quality: Evidence from ambulance referral patterns.” Review of Economics and Statistics, 101. 19 Gardner, John W. and Jill S. Sanborn (1990), “Years of potential life lost (ypll) - what does it measure?” Epidemiology, 1, 322–329. Gaynor, Martin (2018), “Examining the impact of health care consolidation: Statement before the committee on energy and commerce oversight and investigations subcommittee, u.s. house of representatives.” Gichangi, Anthony and Werner Vach (2005), “The analysis of competing risks data: a guided tour.” Statistics in Medicine, 132, 1–41. Goldstein, Joshua R. and Ronald D. Lee (2020), “Demographic perspectives on the mortality of covid-19 and other epidemics.” Proceedings of the National Academy of Sciences of the United States of America, 117, 22035–22041. Gupta, Aakriti, Mahesh V. Madhavan, Kartik Sehgal, Nandini Nair, Shiwani Mahajan, Tejasav S. Sehrawat, Behnood Bikdeli, Neha Ahluwalia, John C. Ausiello, Elaine Y. Wan, Daniel E. Freedberg, Ajay J. Kirtane, Sahil A. Parikh, Mathew S. Maurer, Anna S. Nordvig, Domenico Accili, Joan M. Bathon, Sumit Mohan, Kenneth A. Bauer, Martin B. Leon, Harlan M. Krumholz, Nir Uriel, Mandeep R. Mehra, Mitchell S.V. Elkind, Gregg W. Stone, Allan Schwartz, David D. Ho, John P. Bilezikian, and Donald W. Landry (2020), “Extrapulmonary manifestations of covid-19.” Nature Medicine, 26, 1017–1032. Halloran, M. Elizabeth (2009), “Communicable diseases and data analysis.” In Biometrics - Volume II, 148–161, EOLSS Publishers. Heist, Tyler, Karyn Schwartz, and Sam Butler (2021), “Trends in overall and non-covid-19 hospital admissions.” Kaiser Family Foundation. Holshue, Michelle L., Chas DeBolt, Scott Lindquist, Kathy H. Lofy, John Wiesman, Hollianne Bruce, Christopher Spitters, Keith Ericson, Sara Wilkerson, Ahmet Tural, George Diaz, and et al. Cohn, Amanda (2020), “First case of 2019 novel coronavirus in the united states.” New England Journal of Medicine, 382, 929–936. Jann, Ben (2008), “The blinder–oaxaca decomposition for linear regression models.” The Stata Journal, 8, 453–479. Jha, Ashish K., E. John Orav, and Arnold M. Epstein (2011), “Low-quality, high-cost hospitals, mainly in south, care for sharply higher shares of elderly black, hispanic, and medicaid patients.” Health Affairs, 30, 1904–1911. Jose, Ricardo J and Ari Manuel (2020), “Covid-19 cytokine storm: the interplay between inflammation and coagulation.” Lancet Respiratory Medicine, 8, E46–E47. Kakani, Pragya, Amitabh Chandra, Sendhil Mullainathan, and Ziad Obermeyer (2020), “Allocation of covid-19 relief funding to disproportionately black counties.” Journal of the American Medical Association. 20 Krishnan, Lakshmi, S. Michelle Ogunwole, and Lisa A. Cooper (2020), “Historical insights on coronavirus disease 2019 (covid-19), the 1918 influenza pandemic, and racial disparities: Illuminating a path forward.” Annals of Internal Medicine, 173, 474–482. Long, Brit, William J Brady, Alex Koyfman, and Michael Gottlieb (2020), “Cardiovascular complications in covid-19.” American Journal of Emergency Medicine, 38, 1504–1507. Malas, Mahmoud B., Isaac N. Naazie, Nadin Elsayed, Asma Mathlouthi, Rebecca Marmor, and Bryan Clary (2020), “Thromboembolism risk of covid-19 is high and associated with a higher risk of mortality: A systematic review and meta-analysis.” Lancet EClinicalMedicine, 29. Mein, Stephen A (2020), “Covid-19 and health disparities: the reality of “the great equalizer”.” Journal of General Internal Medicine, 35, 2439–2440. Montenovo, Laura, Xuan Jiang, Felipe Lozano Rojas, Ian M Schmutte, Kosali I Simon, Bruce A Weinberg, and Coady Wing (2020), “Determinants of disparities in covid-19 job losses.” Technical report, National Bureau of Economic Research. National Center for Health Statistics (2020a), “Bridged-race population estimates 1990-2002.” https://wonder.cdc.gov/Bridged-Race-v2002.HTML. Accessed 5/11/2021. National Center for Health Statistics (2020b), “Bridged-race population estimates 1990-2019.” https://wonder.cdc.gov/Bridged-Race-v2019.HTML. Accessed 5/11/2021. National Center for Health Statistics (2020c), “Compressed mortality file: 1979-1998 with icd-9 codes.” https://wonder.cdc.gov/cmf-icd9.html. Accessed 1/11/2021. National Center for Health Statistics (2020d), “Excess deaths associated with covid-19.” Center for Disease Control and Prevention, Washington, DC. National Center for Health Statistics (2020e), “Underlying cause of death, 1999-2019.” https:// wonder.cdc.gov/ucd-icd10.html. Accessed 5/11/2021. National Center for Health Statistics (2021a), “Ah monthly provisional counts of deaths for select causes of death by age, and race and hispanic origin.” https://data.cdc.gov/NCHS/AHMonthly-Provisional-Counts-of-Deaths-for-Select/r5pw-bk5t. National Center for Health Statistics (2021b), “Provisional drug overdose death counts.” https: //www.cdc.gov/nchs/nvss/vsrr/drug-overdose-data.htm. National Center for Health Statistics (2021c), “Technical notes: Provisional death counts for coronavirus disease (covid-19).” https://www.cdc.gov/nchs/nvss/vsrr/covid19/tech_notes.htm. National Research Council (2009), “Vital statistics: Summary of a workshop.” 87–109, https:// www.ncbi.nlm.nih.gov/books/NBK219884/. 21 Ndugga, Nambi, Olivia Pham, Latoya Hill, Samantha Artiga, Raisa Alam, and Noah Parker (2021), “Latest data on covid-19 vaccinations race/ethnicity.” Kaiser Family Foundation. Optum (2021), “Clinformatics® data mart.” Accessed 5/11/2021. Patel, Sadiq Y., Ateev Mehrotra, Haiden A. Huskamp, Lori Uscher-Pines, Ishani Ganguli, and Michael L. Barnett (2021), “Trends in outpatient care delivery and telemedicine during the covid-19 pandemic in the us.” JAMA Internal Medicine, 181. Petrilli, Christopher M, Simon A Jones, Jie Yang, Harish Rajagopalan, Luke O’Donnell, Yelena Chernyak, Katie A Tobin, Robert J Cerfolio, Fritz Francois, and Leora I Horwitz (2020), “Factors associated with hospital admission and critical illness among 5279 people with coronavirus disease 2019 in new york city: prospective cohort study.” BMJ, 369. Pew Research Center (2018), “A record 64 million americans live in multigenerational households.” https://www.pewresearch.org/fact-tank/2018/04/05/a-record-64-million-americanslive-in-multigenerational-households/. Pew Research Center (2020), “Intent to get a covid-19 vaccine rises to 60% as confidence in research and development process increases.” https://www.pewresearch.org/science/2020/12/ 03/intent-to-get-a-covid-19-vaccine-rises-to-60-as-confidence-in-research-and-developmentprocess-increases/. Physicians Foundation (2020), “2020 survey of america’s physicians.” physiciansfoundation.org/research-insights/2020physiciansurvey/. https:// Polyakova, Maria, Geoffrey Kocks, Victoria Udalova, and Amy Finkelstein (2020), “Initial economic damage from the covid-19 pandemic in the united states is more widespread across ages and geographies than initial mortality impacts.” Proceedings of the National Academy of Sciences of the United States of America, 117, 27934–27939. Polyakova, Maria, Victoria Udalova, Geoffrey Kocks, Katie Genadek, Kieth Finlay, and Amy Finkelstein (2021), “Racial disparities in excess all-cause mortality during the early covid-19 pandemic varied substantially across states.” Health Affairs, 40. Rees-Jones, Alex, John D’Attoma, Amedeo Piolatto, and Luca Salvadori (2020), “Covid-19 changed tastes for safety-net programs.” National Bureau of Economic Research, 1–20. Reeves, Richard J and Faith Smith (2020), “Black and hispanic americans at higher risk of hypertension, diabetes, obesity: Time to fix our broken food system.” Brookings Up Front, https://www.brookings.edu/blog/up-front/2020/08/07/black-and-hispanic-americansat-higher-risk-of-hypertension-diabetes-obesity-time-to-fix-our-broken-food-system/. Rubin-Miller, Lily, Christopher Alban, Samantha Artiga, and Sean Sullivan (2020), “Covid-19 racial disparities in testing, infection, hospitalization, and death: Analysis of epic patient data.” Kaiser Family Foundation. 22 Ruhm, Christopher J (2000), “Are recessions good for your health?” The Quarterly journal of economics, 115, 617–650. Shattuck, Lemuel (1846), “Report to the committee of the city council appointed to obtain the census of boston for the year 1845, embracing collateral facts and statistical researches, illustrating the history and condition of the population, and their means of progress and prosperity.” Simonds, Artemas (1850), “Report by the city registrar of the births, marriages, and deaths, in the city of boston, for the year 1849.” Simonov, Andrey, Szymon K. Sacher, Jean-Pierre H. Dubé, and Shirsho Biswas (2020), “The persuasive effect of fox news: Non-compliance with social distancing during the covid-19 pandemic.” National Bureau of Economic Research, 1–69. Snowden, Lonnie R and Genevieve Graaf (2020), “Covid-19, social determinants past, present, and future, and african americans’ health.” J Racial Ethn Health Disparities, 8. Stevens, Ann H., Douglas L. Miller, Marianne E. Page, and Mateusz Filipski (2015), “The best of times, the worst of times: Understanding pro-cyclical mortality.” American Economic Journal: Economic Policy, 7, 279–31. United States Census Bureau (2020a), “2010-19 american community survey 1-year estimates, tables b01001b, b01001c, b01001d, b01001h, and b01001i.” https://data.census.gov/cedsci/. Accessed 5/11/2021. United States Census Bureau (2020b), “Household pulse survey public use file (puf).” https:// www.census.gov/programs-surveys/household-pulse-survey/datasets.html. Virchow, Rudolf (1848). Die Medizinische Reform. Eine Wochenschrift, 1, 182. Whaley, Christopher M., Megan F. Pera, Jonathan Cantor, Jennie Chang, Julia Velasco, Heather K. Hagg, Neeraj Sood, and Dena M. Bravata (2020), “Changes in health services use among commercially insured us populations during the covid-19 pandemic.” JAMA Network Open, 3. Wright, Ephraim M. (1855), “Thirteenth report to the legislature of massachusetts, relating to the registry and returns of births, marriages, and deaths, in the commonwealth, for the year ending december 31, 1854.” Wu, Sean L., Andrew N. Merten, Yoshika S. Crider, Anna Nyugen, Nolan N. Pokpongkiat, Stephanie Djajadi, Anmol Seth, Michelle S. Hsiang, John M. Colford Jr., Art Reingold, Benjamin F. Arnold, Alan Hubbard, and Jade Benjamin-Chung (2020), “Substantial underestimation of sarscov-2 infection in the united states.” Nature Communications, 11. Yellow Horse, Aggie J. and Kimberly R. Huyser (2021), “Indigenous data sovereignty and covid-19 data issues for american indian and alaska native tribes and populations.” Journal of Population Research. 23 Yun, Myeong-Su (2005), “A simple solution to the identification problem in detailed wage decompositions.” Economic Inquiry, 43, 766–772. Ziedan, Engy, Kosali Simon, and Coady Wing (2020), “Effects of state covid-19 closure policy on non-covid-19 health care utilization.” National Bureau of Economic Research, 1–68. 24 Appendix Age-adjusted mortality rate, 2019 (per 1000 population) Age-adjusted mortality rate, 2020 (per 1000 population) Baseline Mortality 12 10 Δ = 1.51 Δ = 0.88 8 Ref. 6 Δ = -2.02 Δ = -3.14 4 Pandemic Mortality 12 Δ = 2.78 10 Δ = 1.44 Ref. 8 Δ = -0.82 6 Δ = -3.57 4 2 2 0 Asian Hispanic White AIAN Black 0 Asian Hispanic White AIAN Black COVID-19 Other Causes Appendix Figure 1: All-Cause Mortality by Race/Ethnicity, 2019 and 2020 Source: Authors’ calculations from National Center for Health Statistics (2020a, 2020e, 2021a) and United States Census Bureau (2020a). Note: Figure plots age-standardized mortality rates from COVID-19 and other causes for non-Hispanic Asian, Hispanic, non-Hispanic White, non-Hispanic American Indian and Alaska Native (AIAN), and non-Hispanic Black Americans during 2019 and 2020. This figure expands on Figure 1, which displays age-standardized mortality rates in 2019 and 2020 for non-Hispanic White and Black individuals. Labels above each bar indicate the difference in the age-standardized rate of mortality in the given year between the racial/ethnic group of interest and non-Hispanic Whites. Area shaded blue denotes recorded mortality from the pandemic disease, while area shaded grey denotes mortality from other causes. Appendix Table 1: Missing Elements in the CDC Case Surveillance Datafile Cases (%) (1) Age 114,238 (0.69) (2) Sex 180,080 (1.09) (3) Race/Ethnicity 6,198,330 (37.50) (4) Comorbidities 14,603,487 (88.35) (5) Any 14,938,556 (90.38) A.2 Hospitalizations 400 2,070 (%) (0.05) (0.27) 125,967 (16.24) 572,170 (73.76) 598,599 (77.17) Deaths (%) 21 774 (0.01) (0.26) 52,573 (17.34) 233,414 (76.98) 239,672 (79.04) Source: Authors’ calculations from Centers for Disease Control and Prevention, COVID-19 Response (2020). Note: Table shows the number and percent of missing elements indicated as column headers in the CDC COVID-19 case surveillance database. Of cases, hospitalization, and deaths reported to the CDC, Columns 1 to 4 show the number and percent of missing age, sex, race/ethnicity, and comorbidities data, respectively. Column 5 shows the number and percent of missing data on any of the four elements. Percentages are reported in parentheses. Note that we do not investigate the extent to which cases are missing entirely from this data source, relative to the counts present in other standard sources of COVID-19 data. Appendix Table 2: COVID-19 Case-Fatality Rates (CFRs) (1) (2) (3) Panel A: Age Groups Panel A: Age Groups Age 0 to 39 Case Fatality Rate 0.126 Percent of Deaths 1.81% Percent of Population 52.08% Age 40 to 49 0.705 2.92% 12.28% Age 50 to 59 1.885 7.63% 12.90% (4) Age 60 to 69 5.759 16.68% 11.58% (5) Age 70+ 24.307 70.97% 11.15% A.3 Case Fatality Rate (Crude) Case Fatality Rate (Age-adjusted) Percent of Deaths Percent of Population Panel B: Race/Ethnicity White Black Hispanic/Latino 5.196 5.534 2.373 2.919 4.627 3.377 60.98% 14.18% 19.57% 61.21% 13.17% 18.45% Asian 4.574 3.967 4.35% 6.32% American Indian 4.538 4.687 0.92% 0.84% Source: Authors’ calculations from Centers for Disease Control and Prevention, COVID-19 Response (2020) and National Center for Health Statistics (2020a, 2020b, 2020e, 2021a). Note: Table reports COVID-19 related case-fatality rates (CFRs) by age groups (Panel A) and race/ethnicity (Panel B). Population and death shares by race/ethnicity are computed as shares of population and deaths with race/ethnicity recorded as non-Hispanic White, non-Hispanic Black, Hispanic/Latino, non-Hispanic Asian, or non-Hispanic American Indian. For clarity of presentation, Alaska Natives are included in the American Indian category, whereas Pacific Islanders are included in the Asian category. Of the 303,211 deaths recorded in the CDC COVID19 case surveillance database, 3.20% are among individuals of races/ethnicities other than those noted above, while 17.34% do not contain information on race/ethnicity.
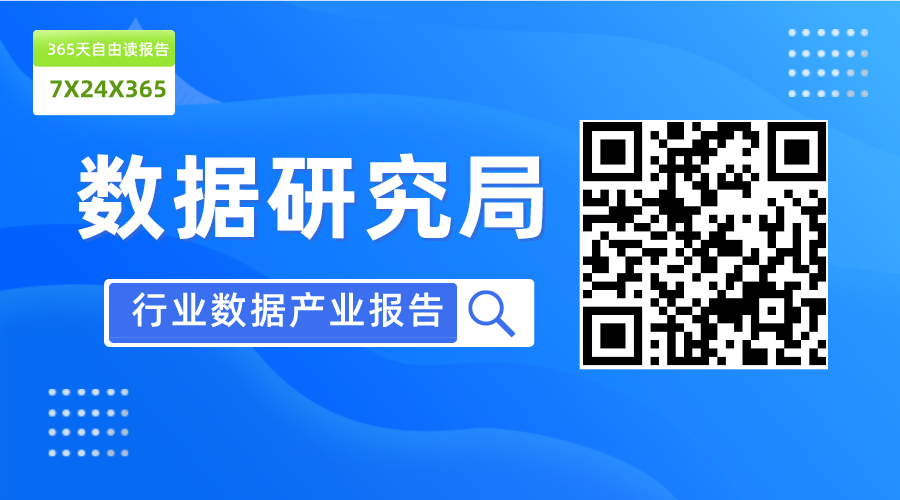
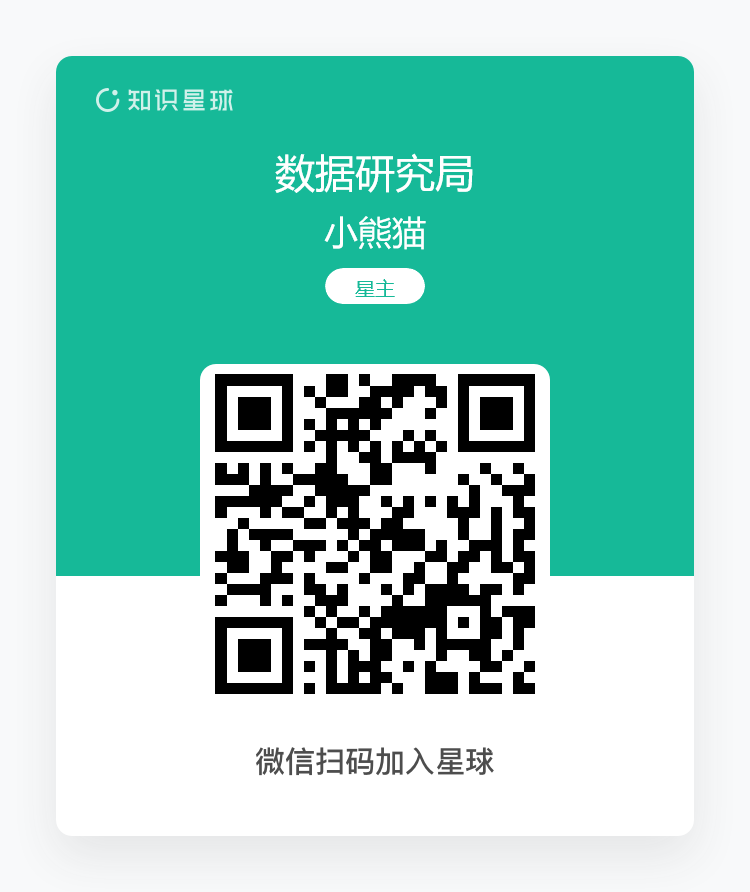